Reconstruction of Incomplete Surface Electromyography Based on an Adversarial Autoencoder Network
SSRN Electronic Journal(2022)
摘要
Surface electromyography (sEMG) signals are often incomplete due to interferences during data measurement, which can degrade sEMG-based applications. To address this issue, this paper proposes a novel adversarial autoencoder model, called the SGMD-AAE, which includes a self-mask generator and a multi-view discriminator. The generator’s binary mask is replaced with a self-mask mechanism, and an adversarial loss is added to promote the reconstruction performance. The multi-view discriminator extracts and fuses deep features of sEMG from time and frequency domains to enhance the generator’s reconstruction ability. The SGMD-AAE model is evaluated on the benchmark NinaPro DB2 database, and the experimental results show that it significantly outperforms incomplete sEMG signals, reducing NRMSE by 88.04% and increasing PSNR by 116.21%. The proposed model also achieves high recognition accuracy for hand gesture recognition even in extreme cases where 90% of the sEMG signals are missing, with an average accuracy exceeding 84%. The effectiveness of the SGMD-AAE model is further verified on a self-collected dataset, demonstrating similar recognition results.
更多查看译文
关键词
Adversarial autoencoder,Missing sEMG,Signal reconstruction,Self-mask,Multi-view discriminator
AI 理解论文
溯源树
样例
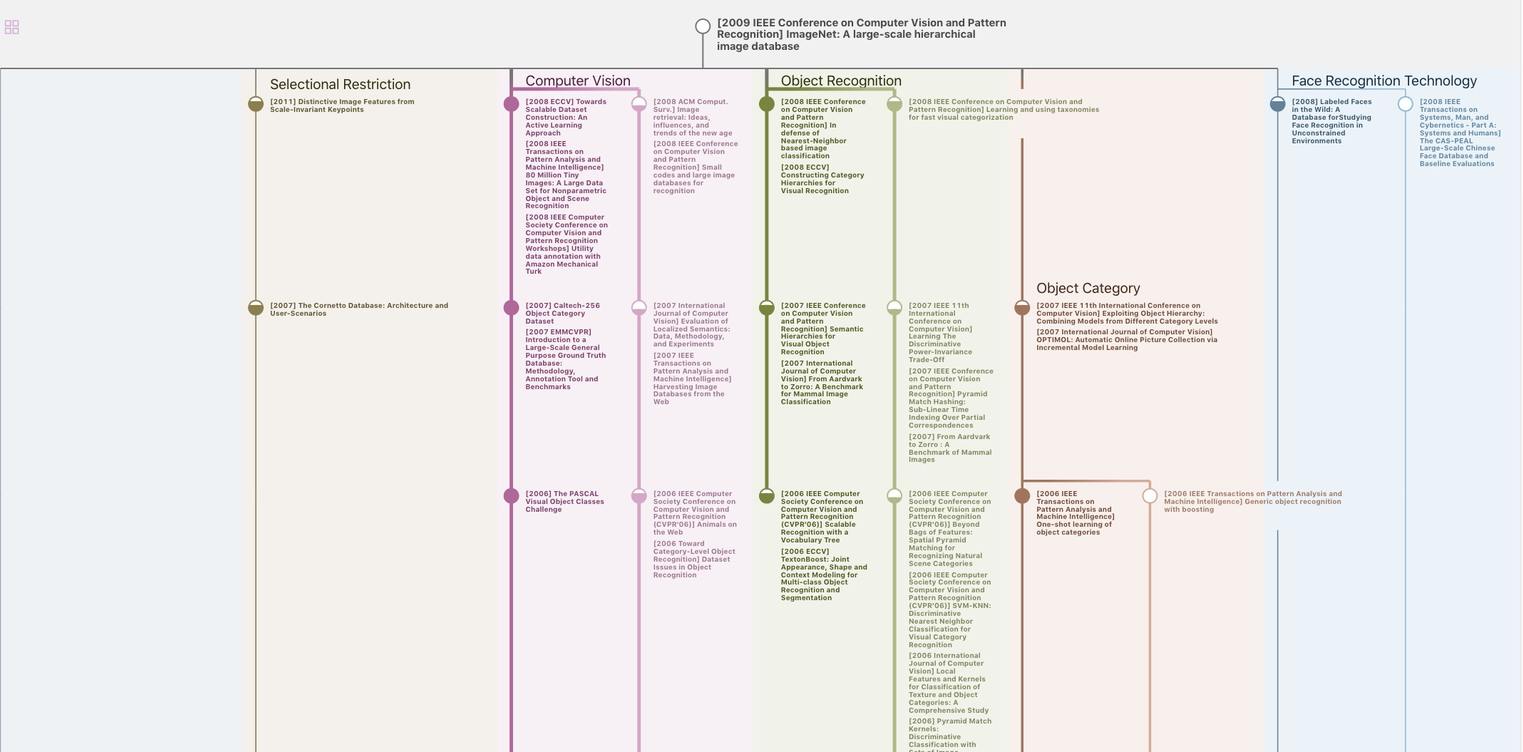
生成溯源树,研究论文发展脉络
Chat Paper
正在生成论文摘要