System Identification of an Actuated Inclined Ball Mechanism via Causation Entropy
Journal of Dynamic Systems, Measurement, and Control(2022)
摘要
Abstract Grey-box and black-box dynamic models are routinely used to model the behavior of real-world dynamic systems. When creating such models, the identification of an accurate model structure (often referred to as covariate selection, feature selection, or sparsity identification) is a critical step required to achieve suitable predictive performance by minimizing the effects of overfitting. Recently, causation entropy has been shown to be quite useful in data-driven covariate selection as it provides a mechanism to measure the causal relationships between the set of covariates and the state dynamics. This work extends previous results by applying the causation entropy covariate selection technique to data from an experimental nonlinear system consisting of a ball rolling on an actuated inclined ramp. Data collected from the system is processed by the causation entropy-based algorithm and covariate selection is performed on a black-box dynamic model. The resulting optimized model is shown to provide better predictive performance than an optimized black-box model which includes extraneous covariates. This study represents the first application of causation entropy-based covariate selection to real-world experimental data, illustrating its use as a practical system identification method.
更多查看译文
关键词
mechanism,ball
AI 理解论文
溯源树
样例
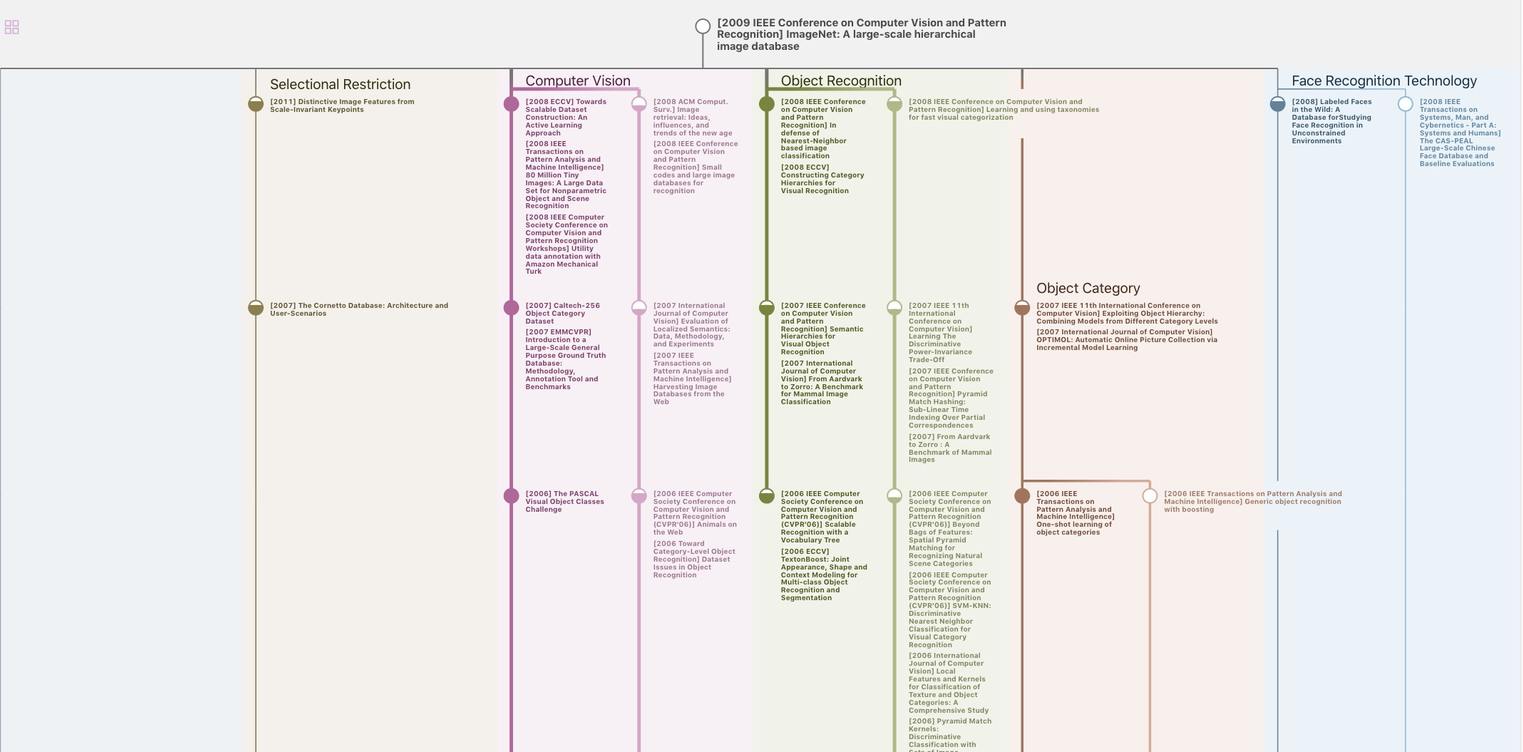
生成溯源树,研究论文发展脉络
Chat Paper
正在生成论文摘要