HAPTR2: Improved Haptic Transformer for legged robots’ terrain classification
Robotics and Autonomous Systems(2022)
摘要
The haptic terrain classification is an essential component of a mobile walking robot control system, ensuring proper gait adaptation to the changing environmental conditions. In practice, such components are a part of an autonomous system and thus have to be lightweight, provide fast inference time, and guarantee robustness to minor changes in recorded sensory data. We propose transformer-based HAPTR and HAPTR2 terrain classification methods that use force and torque measurements from feet to meet these requirements. For reliable comparison of the proposed solutions, we adapt two classical machine learning algorithms (DTW-KNN and ROCKET), one temporal convolution network (TCN), and use the state-of-the-art CNN-RNN. The experiments are performed on publicly available PUTany and QCAT datasets. We show that the proposed HAPTR and HAPTR2 methods achieve accuracy on par or better than state-of-the-art approaches with a lower number of parameters, faster inference time, and improved robustness to input signal distortions. These features make HAPTR and HAPTR2 excel in terrain recognition tasks when considering real-world requirements.
更多查看译文
关键词
Legged robots,Deep learning methods,Data sets for robot learning
AI 理解论文
溯源树
样例
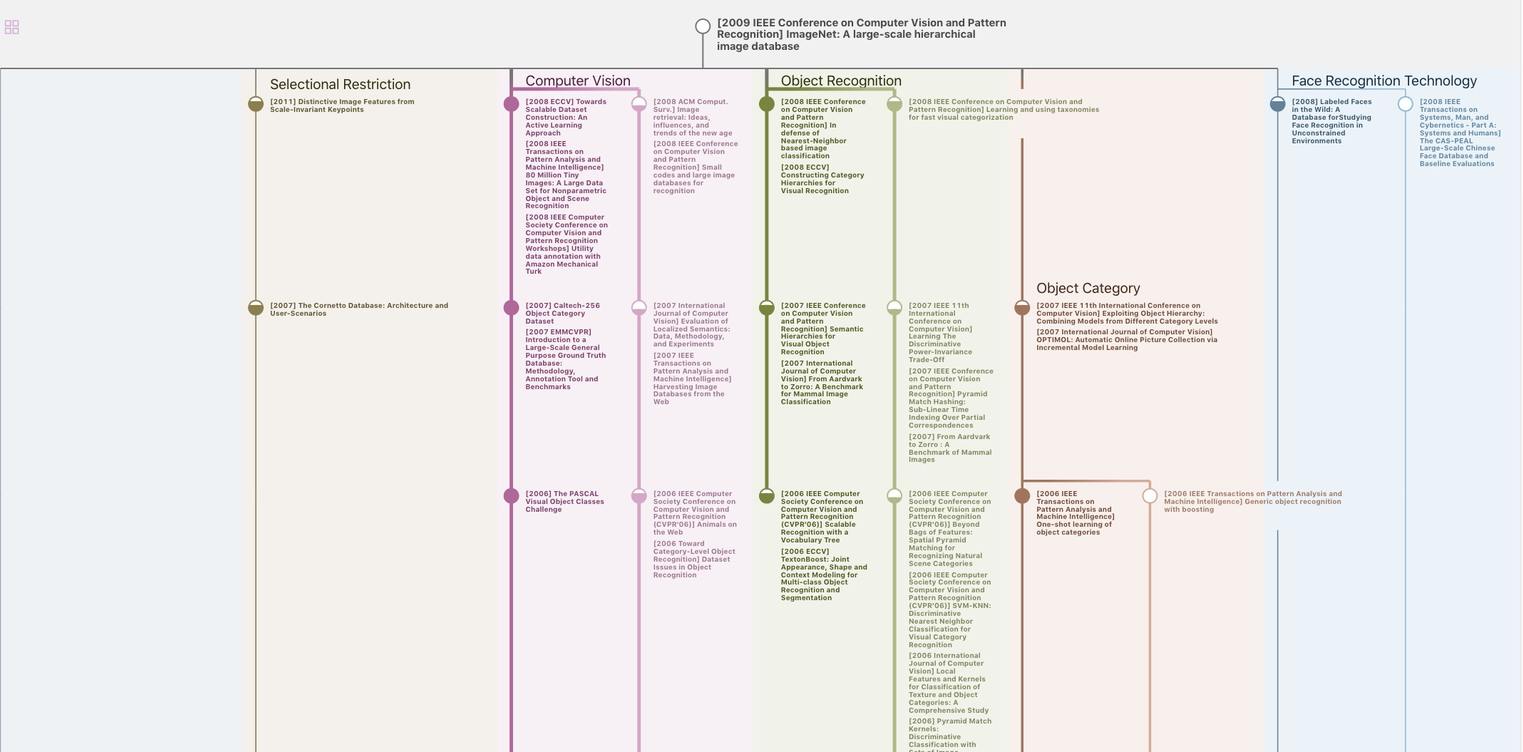
生成溯源树,研究论文发展脉络
Chat Paper
正在生成论文摘要