Deep-Reinforcement-Learning-based User-Preference-Aware Rate Adaptation for Video Streaming
2022 IEEE 23rd International Symposium on a World of Wireless, Mobile and Multimedia Networks (WoWMoM)(2022)
摘要
Online video is the most popular Internet application. As the throughput would frequently change under different network conditions, it is important to adaptively select the proper bitrate and improve user’s quality of experience. In this paper, we propose a new DRL-based rate adaption algorithm for video streaming, which holistically captures user’s preference of video contents, network throughput and buffer occupancy, and select the proper bitrate for video to improve the QoE. Specifically, we use 3D Convolutional neural (C3D) network to learn the spatio-temporal features, and implement the semantic analysis of videos. We also apply the Term Frequency-Inverse Document Frequency (TF-IDF) method to analyze the user’s preference of different scene types, according to its viewing history. The dynamic adaptive streaming is formulated as a Markov Decision Process (MDP) problem, and use the Actor-Critic (A3C) algorithm to dynamically choose the optimal bitrate. As corroborated by simulations, our algorithm can accurately obtain the user’s preference, keep the bitrate allocation consistent with the user’s preference, and maintain video quality. Compared with the state-of-the-art Pensieve algorithm, our algorithm improves the average QoE by at least 12.5%. It also has a significant improvement over other baseline methods.
更多查看译文
关键词
rate adaption,user preference,quality of experience (QoE),video analysis,deep reinforcement learning (DRL),Actor-Critic (A3C)
AI 理解论文
溯源树
样例
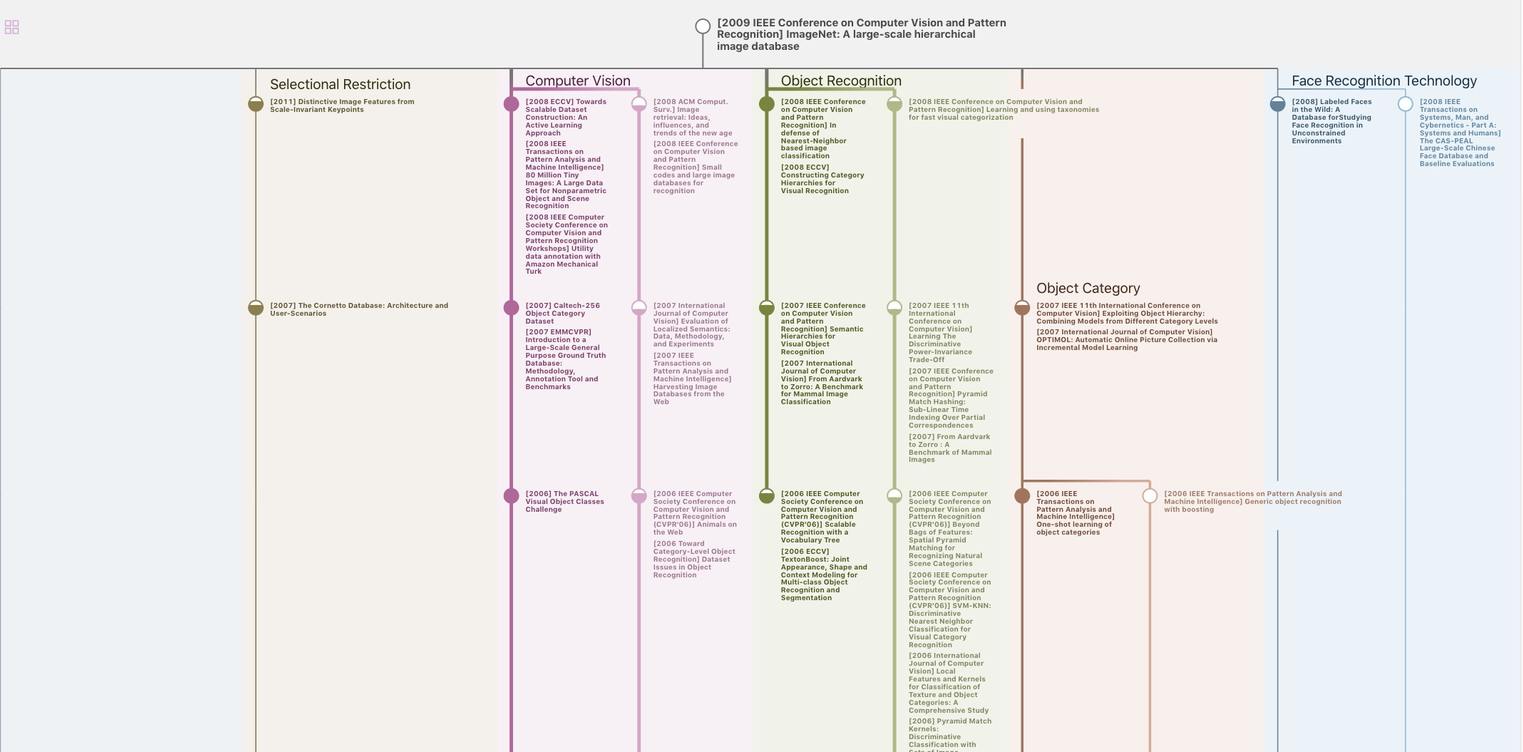
生成溯源树,研究论文发展脉络
Chat Paper
正在生成论文摘要