Intrusion detection system using hybrid classifiers with meta-heuristic algorithms for the optimization and feature selection by genetic algorithm
Computers and Electrical Engineering(2022)
摘要
An intrusion detection system (IDS) is considered critical for detecting threats, intrusions, and unauthorized access. IDS monitors massive network traffic that includes irrelevant and extravagant features that profoundly impact the system’s efficiency and slow down the classification process for accurate decisions. Its effectiveness is tested over the various techniques that comprise an enormous volume of data and heavy network traffic. Many approaches, such as machine learning algorithms, data mining, swarm intelligence, and artificial neural networks (ANN), have been implemented for adequate and improved IDSs. This paper recommends a novel feature selection method using a genetic algorithm (GA) that determines the optimal feature subsets from the NSL-KDD dataset. Further, hybrid classification has been performed using logistic regression (LR) and decision tree (DT) to achieve a better detection rate (DR) and accuracy (ACC). This research applied and compared several meta-heuristic algorithms’ performance to optimize the selected optimal features. The experimental results show that the grey wolf optimization (GWO) algorithm gives the best accuracy of 99.44% and DR of 99.36% with the reduction of features (=20) out of (=41). The results of the proposed work are compared with the existing feature selection methods to verify improved performance.
更多查看译文
关键词
Machine learning,Feature selection,Classification,Hybrid approach,Meta-heuristic,Optimization
AI 理解论文
溯源树
样例
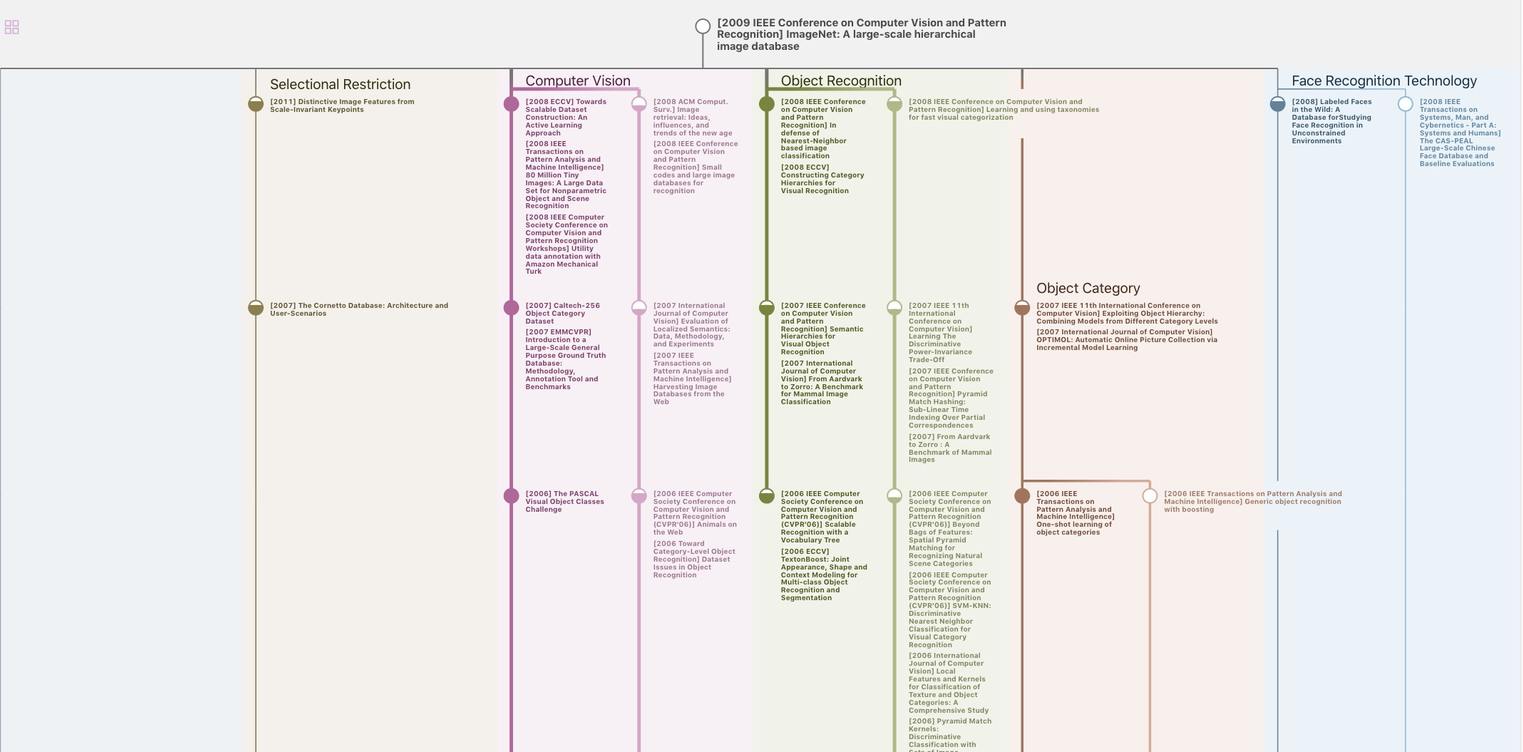
生成溯源树,研究论文发展脉络
Chat Paper
正在生成论文摘要