Learning based speech compressive subsampling
Multimedia Tools and Applications(2022)
摘要
In this paper, we present a learning-based approach to speech compressive subsampling. Prior work in the field has mainly used random or deterministic matrices, which are unaffected by the structure and nature of the signal to be sampled. As a result, recovery algorithms require more computational and power resources to recover the original signal from a small number of samples. The framework presented in this paper aims to propose an efficient methodology for optimised speech sensing matrix design using standard speech datasets via offline training. The effectiveness of the suggested method is assessed using the signal-to-noise ratio that is obtained after reconstructing the original speech signal from samples supplied by the developed matrix. The baseline sensing matrices chosen for this work are widely used random and deterministic matrices, including the Gaussian random matrix, the Bernoulli random matrix, the Toeplitz matrix, and the Hadamard matrix. Convex relaxation and linear reconstruction algorithms were used to perform the signal reconstruction, and the key performance indicators for the relevant sensing matrix are chosen to be reconstruction accuracy and speed. In this work, we observe almost 4% on average improvement in reconstruction accuracy when the proposed method is compared with one of the efficient sensing matrices.
更多查看译文
关键词
Compressive sensing,Sensing matrix,Speech signal recovery,Gaussian random matrix,Deterministic sensing matrix,Learning based compressive subsampling
AI 理解论文
溯源树
样例
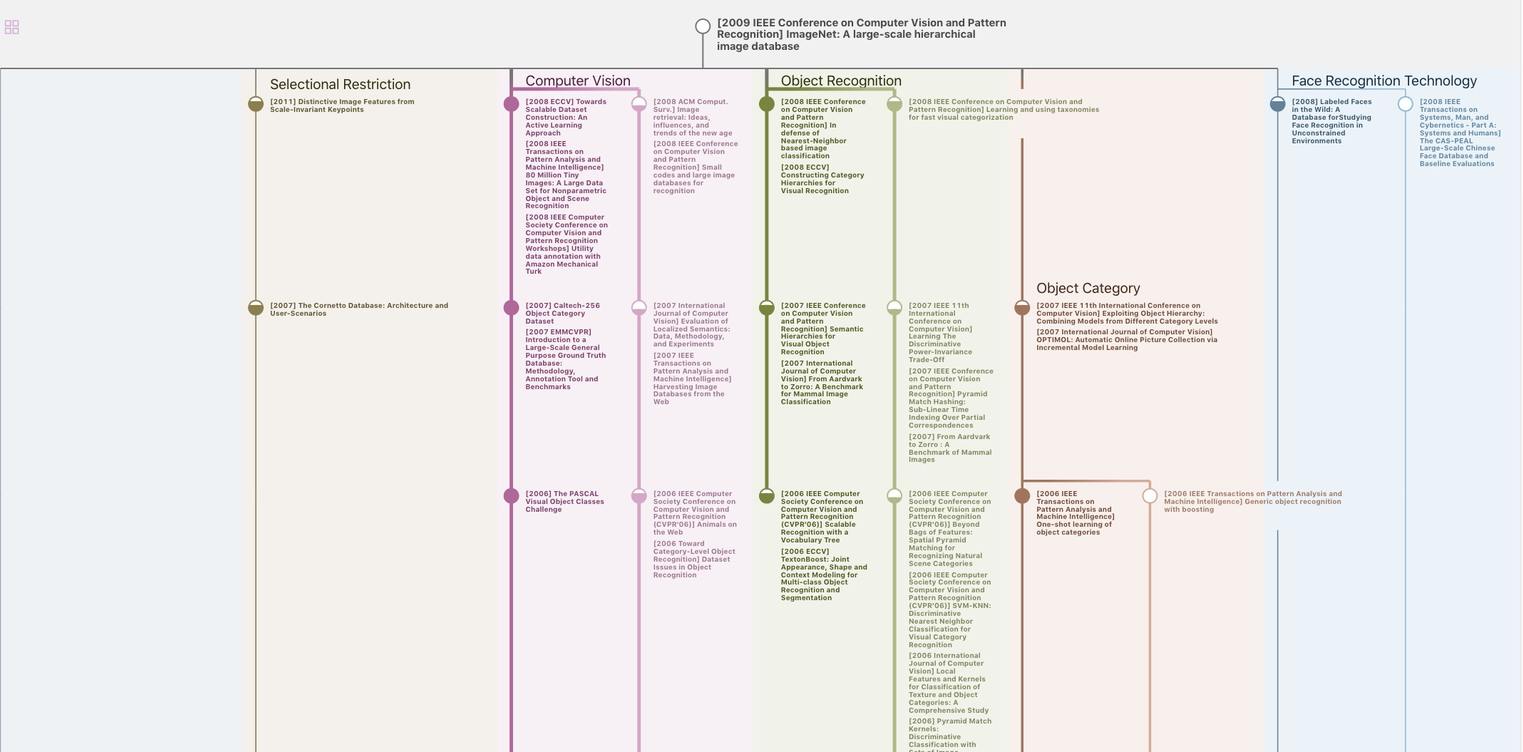
生成溯源树,研究论文发展脉络
Chat Paper
正在生成论文摘要