PyPose: A Library for Robot Learning With Physics-Based Optimization
CVPR 2023(2023)
摘要
Deep learning has had remarkable success in robotic perception, but its data-centric nature suffers when it comes to generalizing to ever-changing environments. By contrast, physics-based optimization generalizes better, but it does not perform as well in complicated tasks due to the lack of high-level semantic information and reliance on manual parametric tuning. To take advantage of these two complementary worlds, we present PyPose: a robotics-oriented, PyTorch-based library that combines deep perceptual models with physics-based optimization. PyPose's architecture is tidy and well-organized, it has an imperative style interface and is efficient and user-friendly, making it easy to integrate into real-world robotic applications. Besides, it supports parallel computing of any order gradients of Lie groups and Lie algebras and 2nd-order optimizers, such as trust region methods. Experiments show that PyPose achieves more than 10x speedup in computation compared to the state-of-the-art libraries. To boost future research, we provide concrete examples for several fields of robot learning, including SLAM, planning, control, and inertial navigation.
更多查看译文
AI 理解论文
溯源树
样例
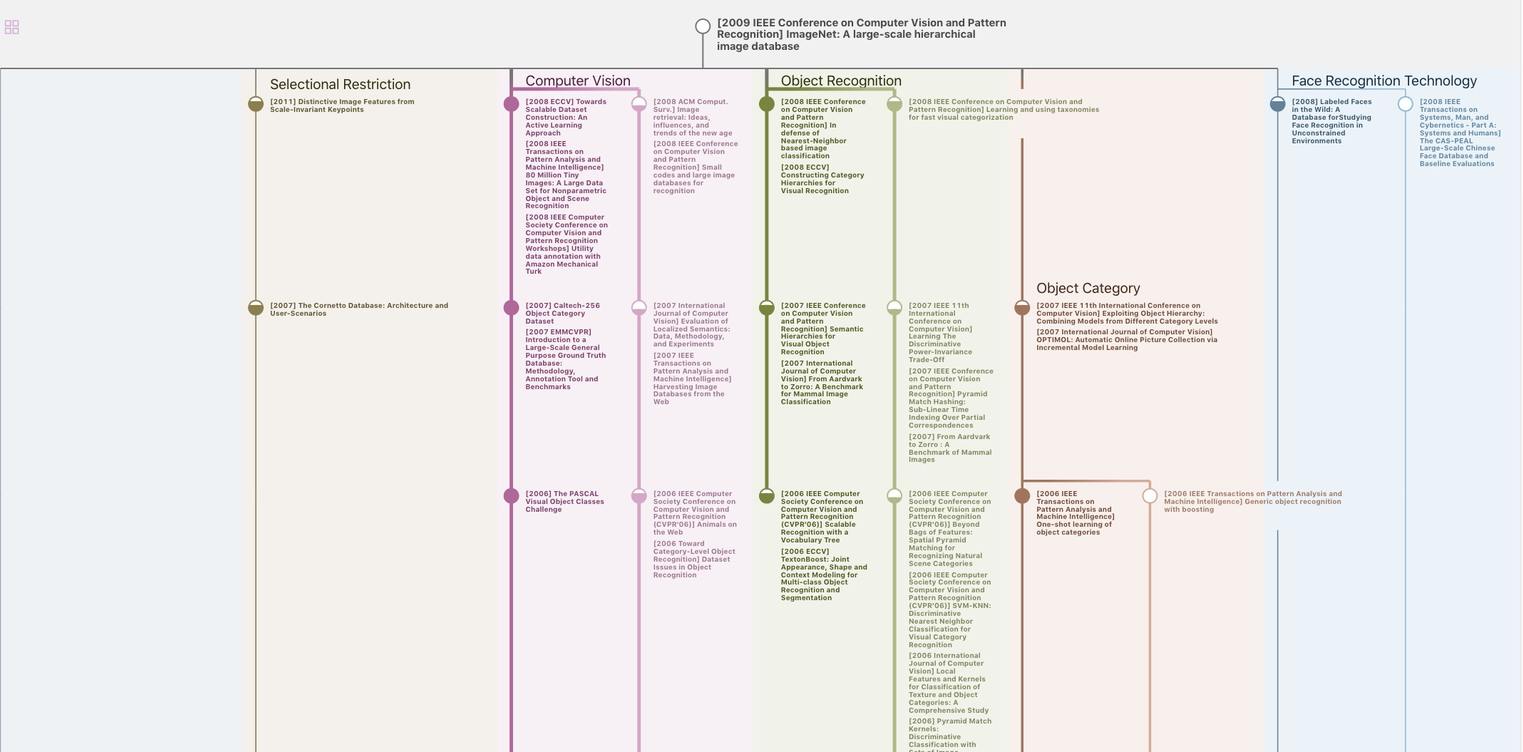
生成溯源树,研究论文发展脉络
Chat Paper
正在生成论文摘要