Multi-label causal feature selection based on neighbourhood mutual information
International Journal of Machine Learning and Cybernetics(2022)
摘要
Multi-label feature selection has gained significant attention over the past decades. However, most existing algorithms are lack of interpretability and uncover the causal mechanisms. As we know, Markov blanket (MB) is a key concept in Bayesian network, which can be used to represent the local causal structure of a variable and the selected optimal features for multi-label feature selection. To select casual features for multi-label learning, in this paper, Parents and Children ( PC ) of each label are discovered via the Hiton method. Then, we distinguish P & C and search Spouses ( SP ) of each label based on neighborhood conditional mutual information. Moreover, the equivalent information phenomenon brought by multi-label datasets will cause some features to be ignored. A metric of conditional independence test is designed, which can be used to retrieve ignored features. In addition, we search common features between relevant labels and label-specific features for a single label. Finally, we propose a M ulti-label C ausal F eature S election with N eighbourhood M utual I nformation algorithm, called MCFS-NMI. To verify the performance of MCFS-NMI, we compare it with five well-established multi-label feature selection algorithms on six datasets. Experiment results show that the proposed algorithm achieves highly competitive performance against all comparing algorithms.
更多查看译文
关键词
Causal relationship,Feature selection,Markov blanket discovery,Multi-label learning,Neighborhood conditional mutual information
AI 理解论文
溯源树
样例
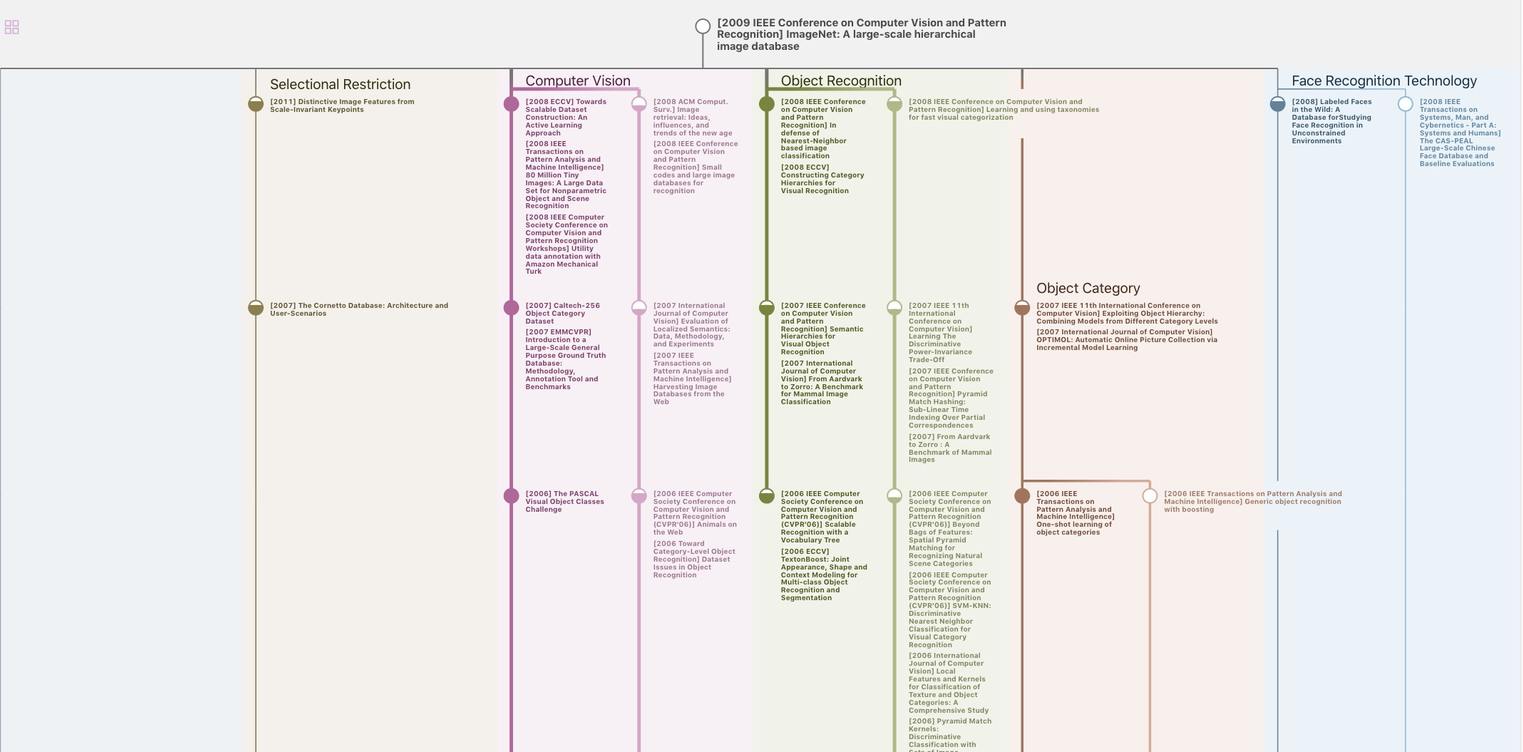
生成溯源树,研究论文发展脉络
Chat Paper
正在生成论文摘要