Frobenius norm-regularized robust graph learning for multi-view subspace clustering
Applied Intelligence(2022)
摘要
Graph learning methods have been widely used for multi-view clustering. However, such methods have the following challenges: (1) they usually perform simple fusion of fixed similarity graph matrices, ignoring its essential structure. (2) they are sensitive to noise and outliers because they usually learn the similarity matrix from the raw features. To solve these problems, we propose a novel multi-view subspace clustering method named Frobenius norm-regularized robust graph learning (RGL), which inherits desirable advantages (noise robustness and local information preservation) from the subspace clustering and manifold learning. Specifically, RGL uses Frobenius norm constraint and adjacency similarity learning to simultaneously explore the global information and local similarity of views. Furthermore, the l 2,1 norm is imposed on the error matrix to remove the disturbance of noise and outliers. An effectively iterative algorithm is designed to solve the RGL model by the alternation direction method of multipliers. Extensive experiments on nine benchmark databases show the clear advantage of the proposed method over fifteen state-of-the-art clustering methods.
更多查看译文
关键词
Multi-view clustering, Graph learning, Subspace clustering, Manifold learning
AI 理解论文
溯源树
样例
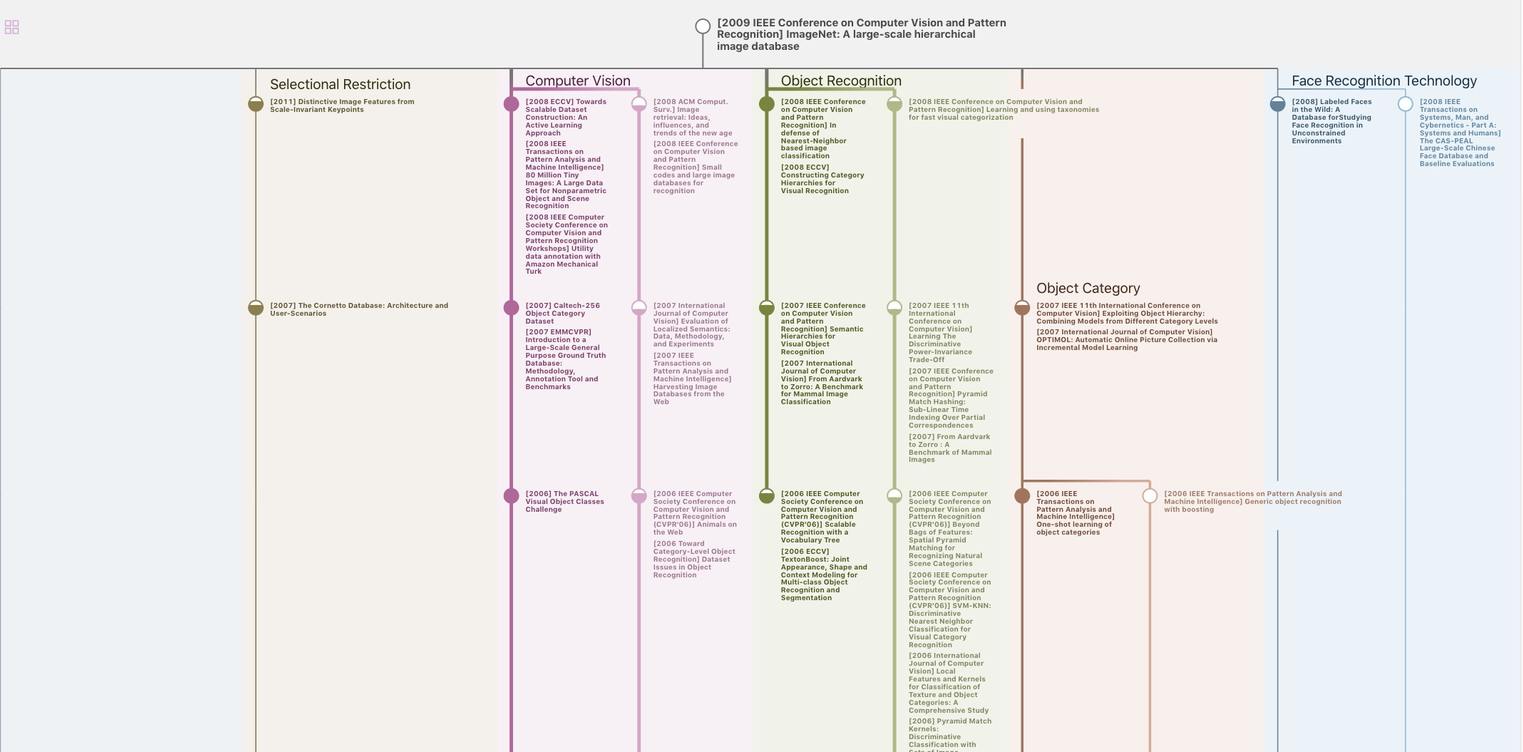
生成溯源树,研究论文发展脉络
Chat Paper
正在生成论文摘要