Logistic Regression With Tangent Space-Based Cross-Subject Learning for Enhancing Motor Imagery Classification
IEEE Transactions on Cognitive and Developmental Systems(2022)
摘要
Brain-computer interface (BCI) performance is often impacted due to the inherent nonstationarity in the recorded EEG signals coupled with a high variability across subjects. This study proposes a novel method using logistic regression with tangent space-based transfer learning (LR-TSTL) for motor imagery (MI)-based BCI classification problems. The single-trial covariance matrix (CM) features computed from the EEG signals are transformed into a Riemannian geometry frame and tangent space features are computed by considering the lower triangular matrix. These are then further classified using the logistic regression model to improve classification accuracy. The performance of LR-TSTL is tested on healthy subjects’ data set as well as on stroke patients’ data set. As compared to existing within-subject learning approaches the proposed method gave an equivalent or better performance in terms of average classification accuracy (78.95 ± 11.68%), while applied as leave-one-out cross-subject learning for healthy subjects. Interestingly, for the patient data set LR-TSTL significantly (
$p< 0.05$
) outperformed the current benchmark performance by achieving an average classification accuracy of 81.75 ± 6.88%. The results show that the proposed method for cross-subject learning has the potential to realize the next generation of calibration-free BCI technologies with enhanced practical usability especially in the case of neurorehabilitative BCI designs for stroke patients.
更多查看译文
关键词
Brain-computer interface (BCI),electroencephalogram (EEG),logistic regression,motor-imagery,Riemannian geometry (RG),tangent space,transfer learning
AI 理解论文
溯源树
样例
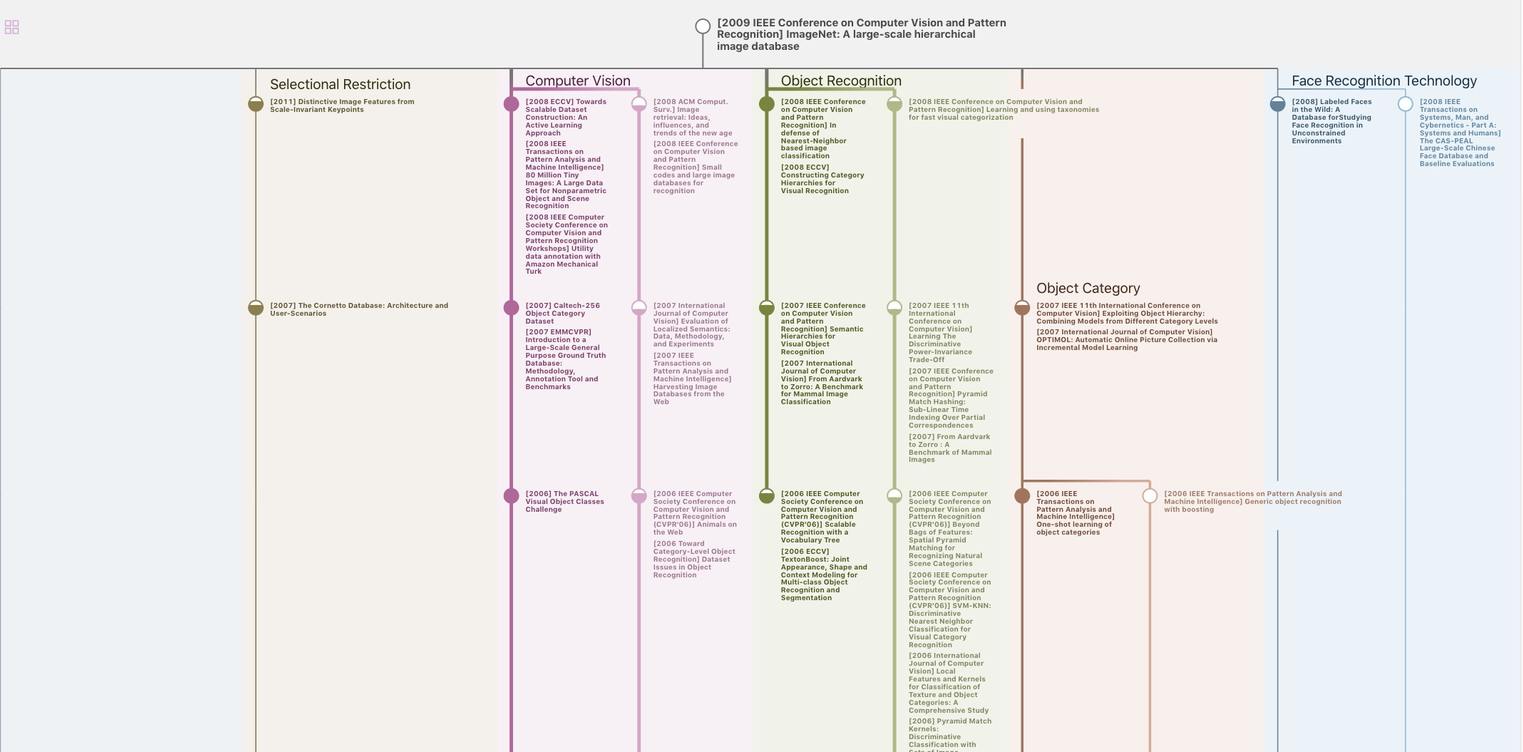
生成溯源树,研究论文发展脉络
Chat Paper
正在生成论文摘要