Deep Autoencoder-Based Anomaly Detection of Electricity Theft Cyberattacks in Smart Grids
IEEE Systems Journal(2022)
摘要
Designing an electricity theft cyberattack detector for the advanced metering infrastructures (AMIs) is challenging due to the limited availability of electricity theft datasets (i.e., malicious datasets). Anomaly detectors, which are trained solely on honest customers’ energy consumption profiles (i.e., benign datasets), could potentially overcome this challenge. Unfortunately, existing anomaly detectors in AMIs present shallow architectures and are incapable of capturing the temporal correlations as well as the sophisticated patterns present in the electricity consumption data, which impact their detection performance negatively. This article proposes the adoption of deep (stacked) autoencoders with a long-short-term-memory (LSTM)-based sequence-to-sequence (seq2seq) structure. The depth of the autoencoders’ structure helps capture the sophisticated patterns of the data and the seq2seq LSTM model enables exploitation of the time-series nature of data. We investigate the performance of simple autoencoder, variational autoencoder, and autoencoder with attention (AEA), in which improved detection performance is observed when seq2seq structures are adopted compared to fully connected ones. Based on the simulation results, the AEA detector offers an enhancement of
$4\!-\!21\%$
and
$4\!-\!13\%$
in terms of detection rate and false alarm rate, respectively, compared to existing state-of-the-art detectors
更多查看译文
关键词
Autoencoders,deep machine learning,electricity stealth,hyperparameter optimization,sequence to sequence (seq2seq)
AI 理解论文
溯源树
样例
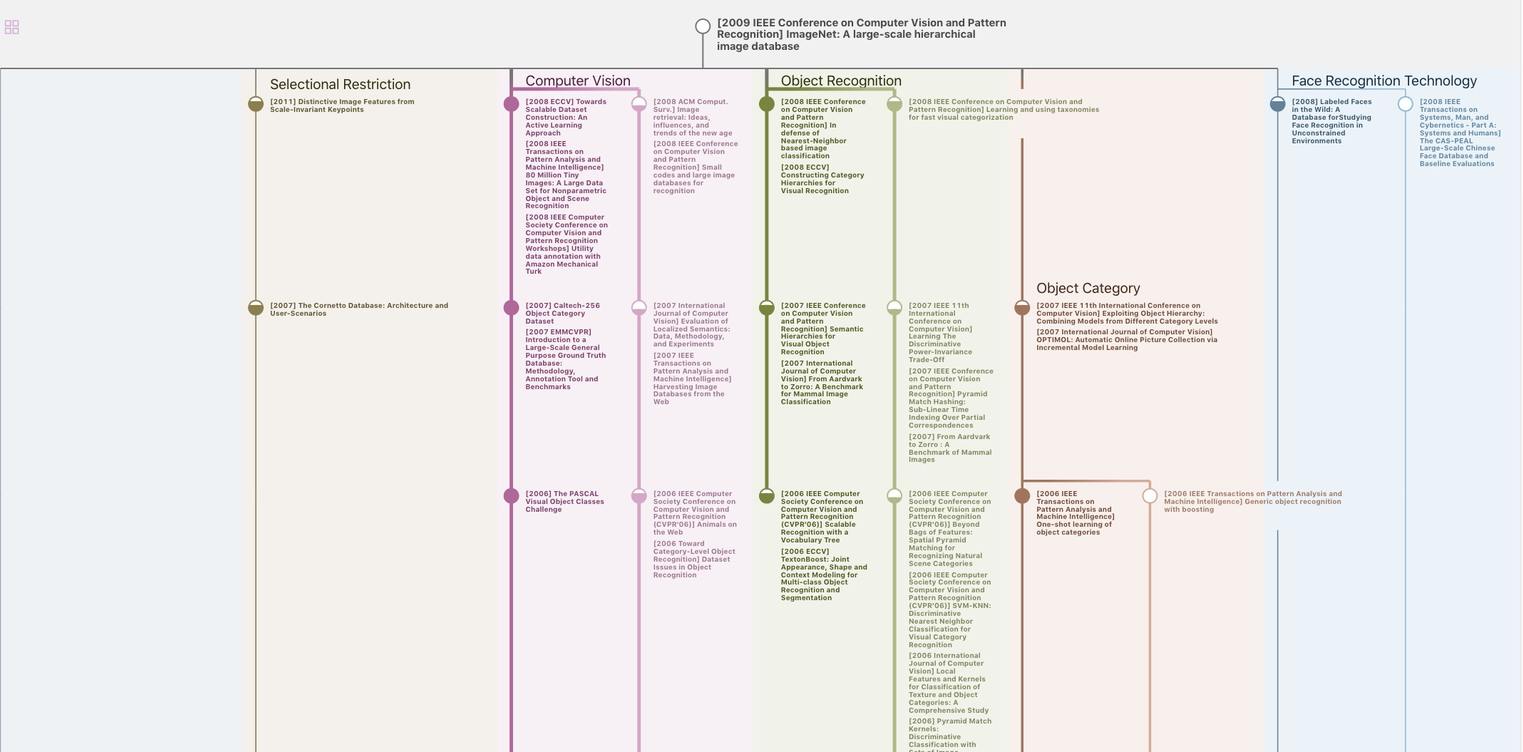
生成溯源树,研究论文发展脉络
Chat Paper
正在生成论文摘要