High-Efficiency Data Conversion Interface for Reconfigurable Function-in-Memory Computing
IEEE Transactions on Very Large Scale Integration (VLSI) Systems(2022)
摘要
Recently, analog in-memory computing (IMC) systems exhibit the considerable potential to break through the inherent high computational latency and energy cost of Von Neumann’s computer architecture. However, inefficient data convertor will inhibit the performance improvement of this system. The tradeoff between different data conversion circuit technologies has turned into one of the major driving forces for the analog IMC system-level improvements. The primary contribution is in two aspects. First, this article shows a digital-to-time-to-analog converter (DTAC) with the tradeoff of latency, area, and power consumption compared to a digital-to-time converter (DTC) and digital-to-analog converter (DAC). Second, we develop an innovative reconfigurable joint-quantization nonlinear analog-to-digital convertor (JQNL-ADC) architecture with lower quantization error by merging the two paradigms of uniform input quantization and uniform output quantization. Compared to conventional DAC, DTAC can reduce power and area by
$50\times $
and
$3\times $
, respectively. Compared to SAR-ADC, our JQNL-ADC can reduce area and power by
$1.6\times $
and
$2\times $
, respectively. In an example of ReRAM-based reconfigurable function-IMC (RFIMC) macro with 256-kb memory, our design can reach 112.9 TOPS/W@8bIN-8bW-8bO under the 28-nm process conditions.
更多查看译文
关键词
AI accelerator,analog in-memory computing (IMC),DAC,DTC,nonlinear activate function,nonlinear ADC (NL-ADC)
AI 理解论文
溯源树
样例
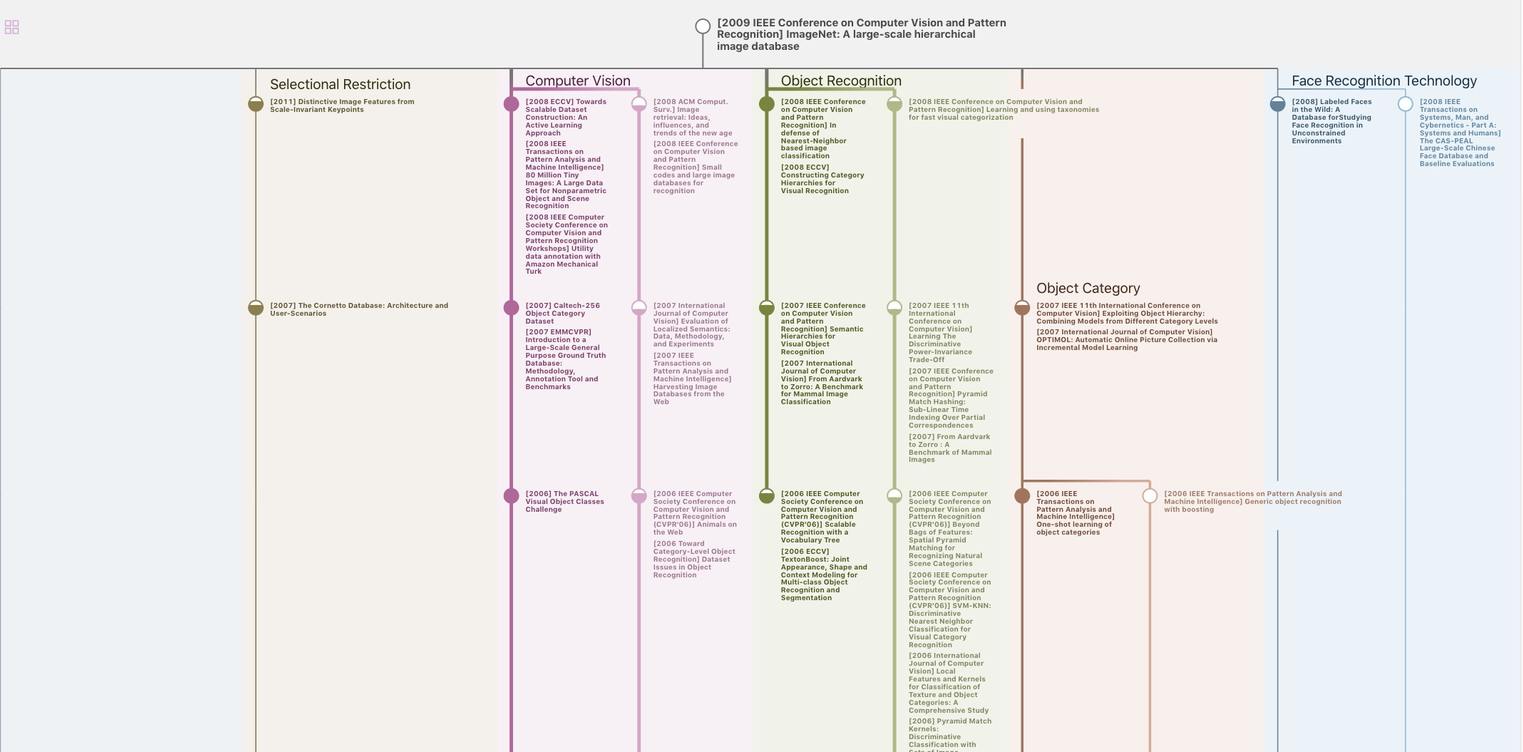
生成溯源树,研究论文发展脉络
Chat Paper
正在生成论文摘要