Information Diffusion for Few-Shot Learning in Robotic Residual Errors Compensation
Intelligent Robotics and Applications(2022)
摘要
In this work, a novel model-free robotic residual errors compensation method is proposed based on the information-diffusion-based dataset enhancement (ID-DE) and the Gradient-Boosted Decision Trees (GBDT). Firstly, the dataset enhancement method is developed by utilizing the normal membership function based on the information diffusion technology. Then, merging it with multiple GBDTs, the multi-output residual errors learning model (ID-GBDTs) is constructed, and the grid search is used to determine the optimal hyper-parameters to accomplish the accurate prediction of residual errors. Finally, the compensation of robotic residual errors is realized by using the calibrated kinematic model. Experiments show that ID-DE can significantly improve the generalization ability of various learning models on the few-shot dataset. The R-squared of ID-GBDTs is improved from 0.58 to 0.77 along with the MAE decreased from 0.23 to 0.16, compared to original GBDT. Through the compensation of the residual errors, the mean/maximum absolute positioning error of the UR10 robot are optimized from 4.51/9.42 mm to 0.81/2.65 mm, with an accuracy improvement of 82.03%.
更多查看译文
关键词
Robot calibration, Residual errors, Dataset enhancement
AI 理解论文
溯源树
样例
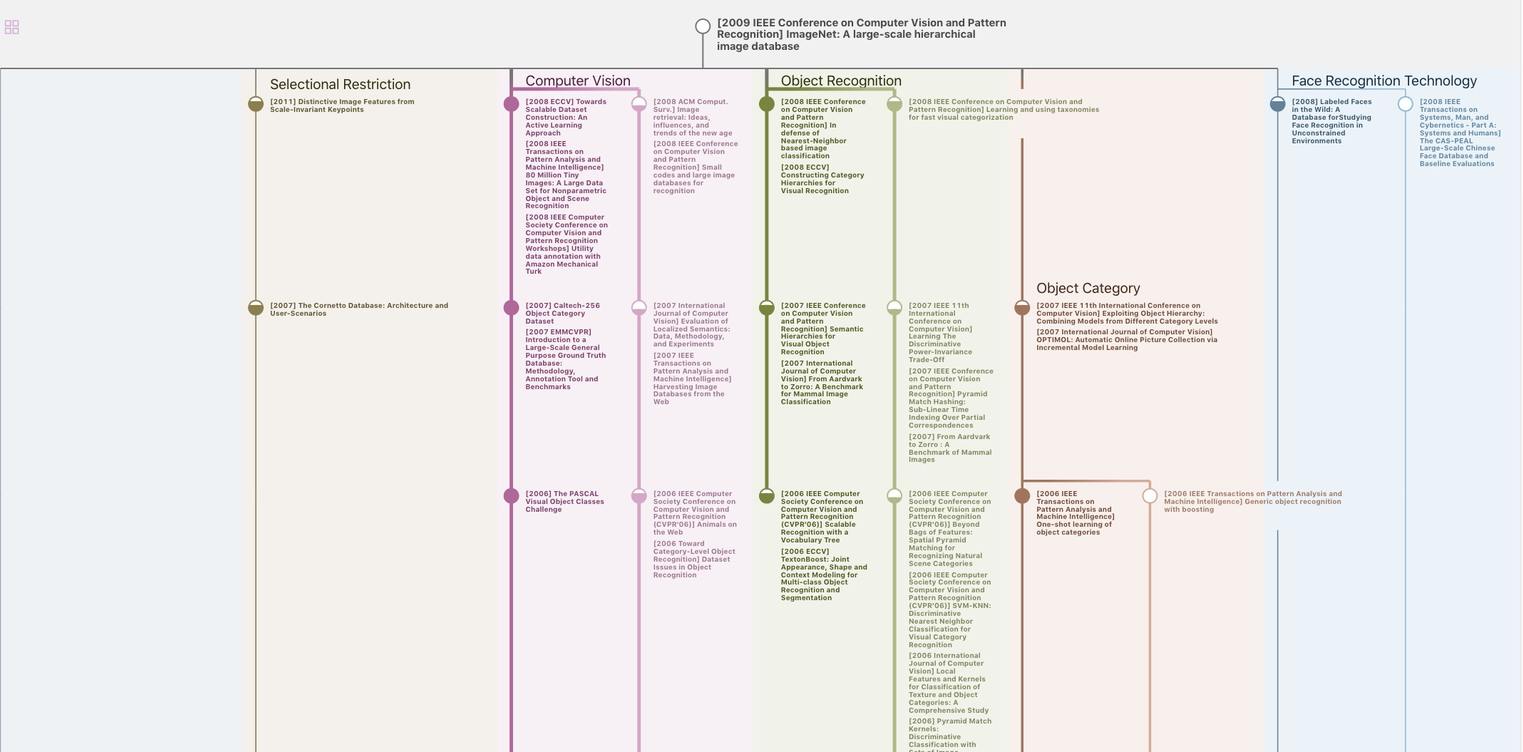
生成溯源树,研究论文发展脉络
Chat Paper
正在生成论文摘要