3DCNN Backed Conv-LSTM Auto Encoder for Micro Facial Expression Video Recognition
Machine Learning and Intelligent Communications(2022)
摘要
Facial Micro-Expression recognition in the field of emotional information processing has become an inexorable necessity for its exotic attributes. It is a non-verbal, spontaneous, and involuntary leakage of true emotion in disguise of most expressive intentional prototypical facial expressions. However, it persists only for a split-second duration and possesses fainted facial muscle movements that make the recognition task more difficult with naked eyes. Besides, there are a limited number of video samples and wide-span domain shifting among datasets. Considering these challenges, several video-based works have been done to improve the classification accuracy but still lack high accuracy. This works addresses these issues and presents an approach with a deep 3D Convolutional Residual Neural Network as a backbone followed by a Long-Short-Term-Memory auto-encoder with 2D convolutions model for automatic Spatio-temporal feature extractions, fine-tuning, and classifications from videos. Also, we have done transfer learning on three standard macro-expression datasets to reduce over-fitting. Our work has shown a significant accuracy gain with extensive experiments on composite video samples from five publicly available micro-expression benchmark datasets, CASME, CASMEII, CAS(ME)2, SMIC, and SAMM. This outweighs the state-of-the-art accuracy. It is the first attempt to work with five datasets and rational implication of LSTM auto-encoder for micro-expression recognition.
更多查看译文
关键词
Micro-expression, Recognition, Deep learning, Transfer learning, Spatio-temporal
AI 理解论文
溯源树
样例
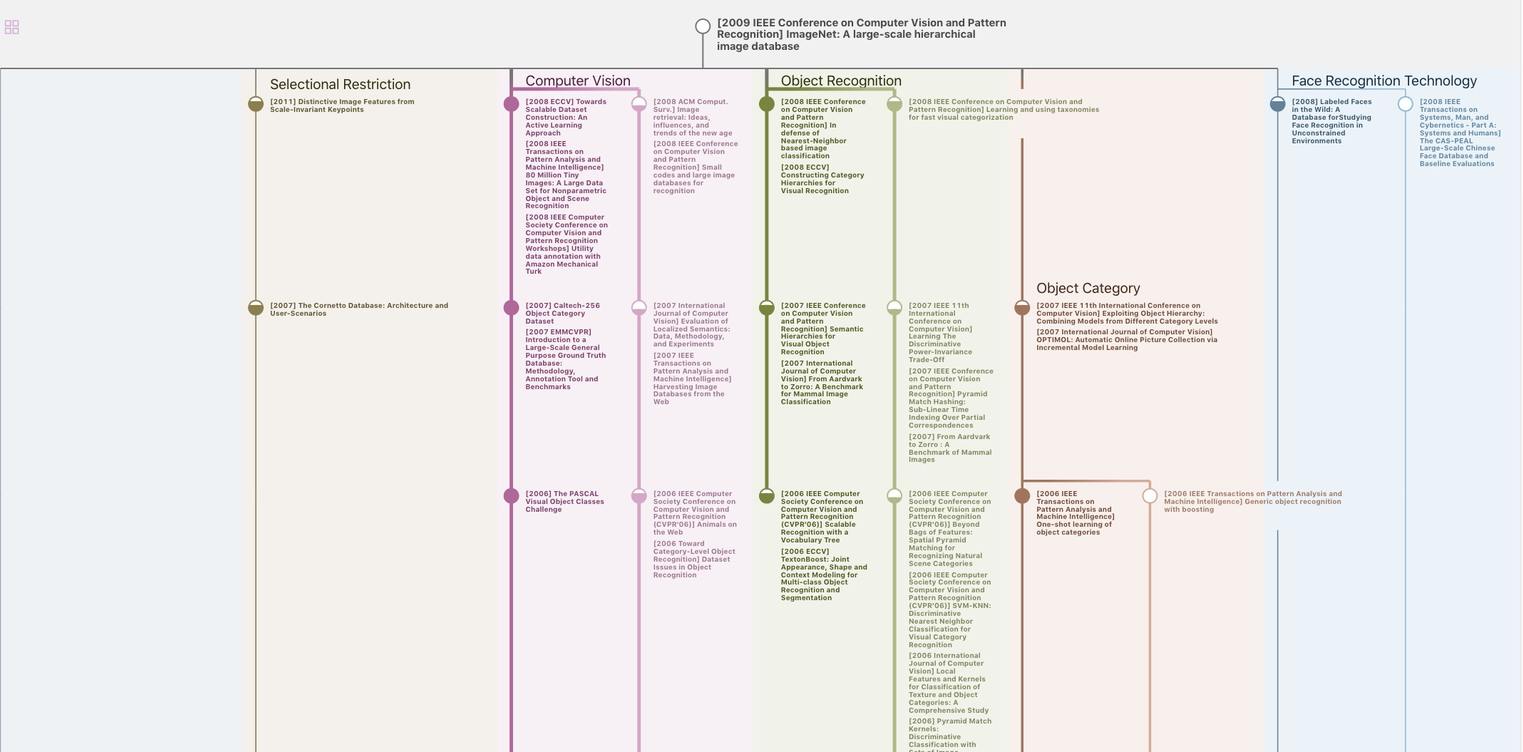
生成溯源树,研究论文发展脉络
Chat Paper
正在生成论文摘要