Topological Data Analysis of Time-Series as an Input Embedding for Deep Learning Models
Artificial Intelligence Applications and Innovations(2022)
摘要
An appreciable portion of medical data exist in the form of a time-series, e.g. electroencephalogram (EEG) and electrocardiogram (ECG) readings of the biopotentials related to the head and heart. Scarcity of labeled data and class imbalance pose challenges when training deep learning models. Topological data analysis (TDA) is an emerging area of research that can be applied to time-series data. In this paper we show that using TDA as a time-series embedding methodology for input to deep learning models offers advantages compared to direct training of such models on the raw data. In our work TDA acts as a generic, low-level feature extractor that is able to capture common signal patterns and thus improve performance with limited training data. Our experimental results on publicly available human physiological biosignal datasets show an improvement in accuracy, especially for imbalanced classes with only a few training instances compared to the full dataset.
更多查看译文
关键词
Topological data analysis, Time-series data, Embedding, Physiological signals
AI 理解论文
溯源树
样例
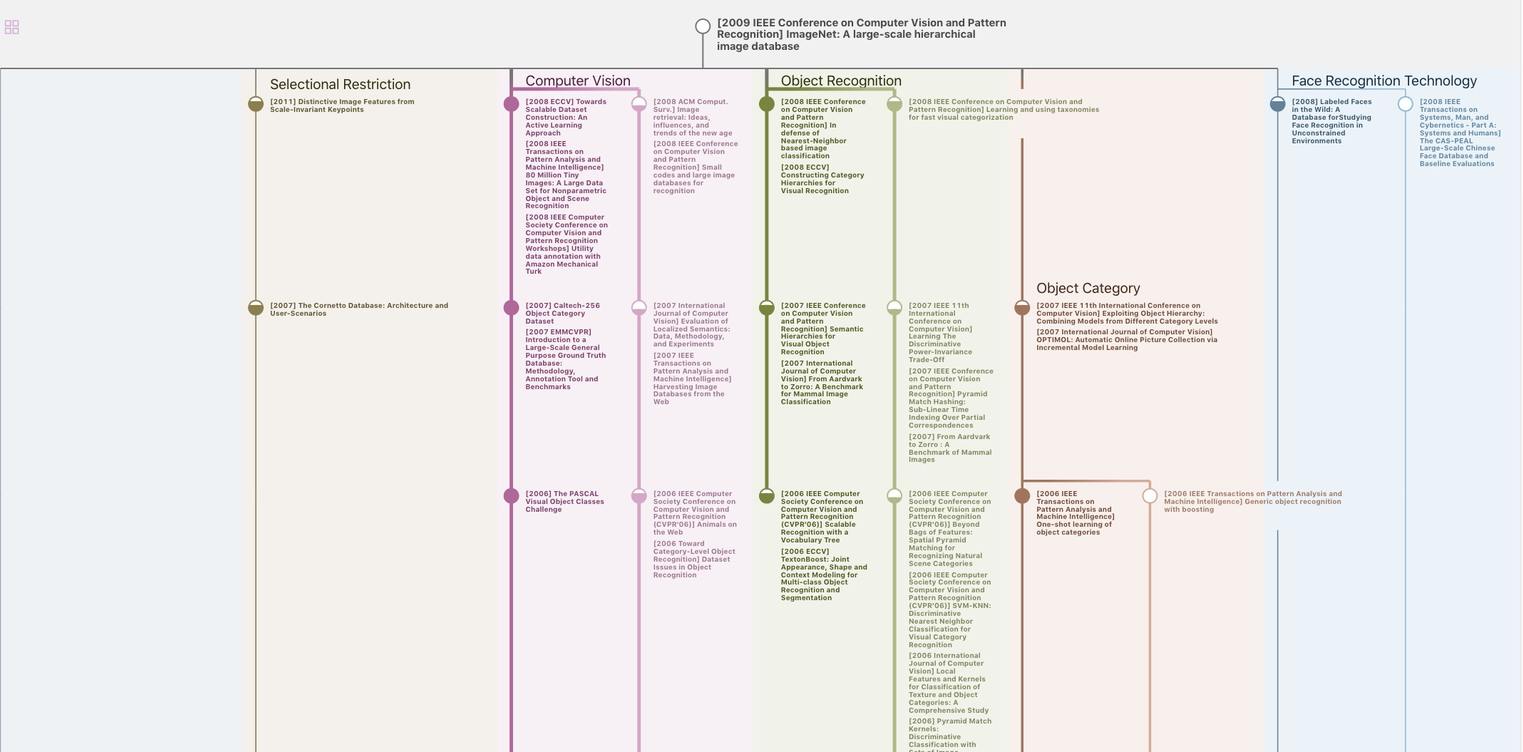
生成溯源树,研究论文发展脉络
Chat Paper
正在生成论文摘要