Hierarchical Multi-scale Architecture Search for Self-supervised Monocular Depth Estimation
Pattern Recognition(2022)
摘要
Self-supervised learning has shown great promising in monocular depth estimation task, using images as the only source of supervision. Most existing methods design the network architecture in a handcrafted manner, which is usually time-consuming and not specific to depth estimation task. To reduce the human efforts in network design, we propose a hierarchical multi-scale Neural Architecture Search framework for self-supervised monocular depth estimation, named Auto-Depth. Specially, our method consists of a two-level search space: cell level and network level. In the cell level, we explore the possible hierarchical connections within one single cell to enhance the ability of multi-scale information representation. In the network level, we search the depth and width of network to balance the accuracy and computational complexity. Furthermore, the two-level path binarization is adopted to reduce the computational cost in searching stage, which only activate one operation path among several candidate operations. Extensive experiments on the KITTI and Make3D datasets show that our searched network yields better performance than previous state-of-the-art monocular depth estimation methods.
更多查看译文
关键词
Neural Architecture Search, Monocular depth estimation, Deep learning
AI 理解论文
溯源树
样例
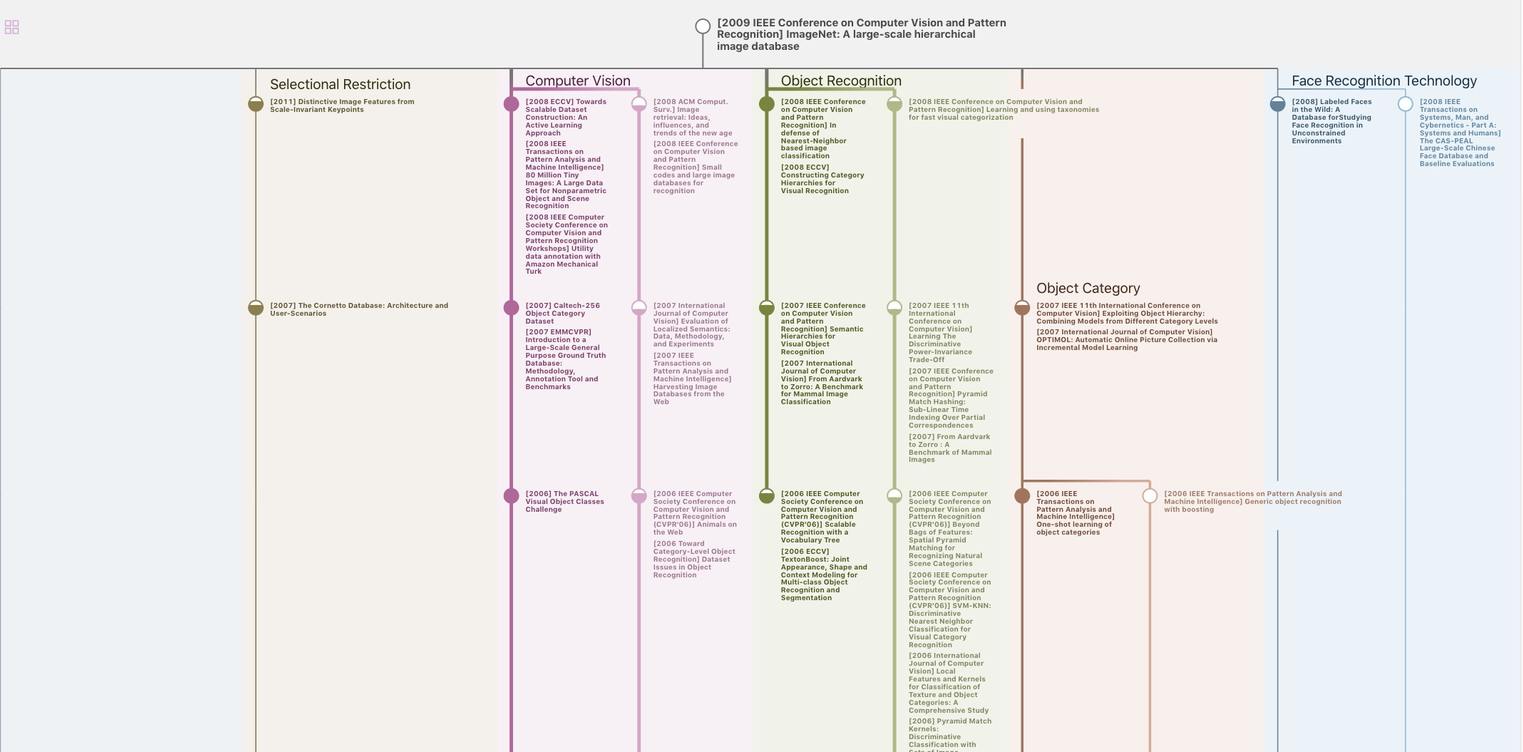
生成溯源树,研究论文发展脉络
Chat Paper
正在生成论文摘要