Document Layout Analysis with Variational Autoencoders: An Industrial Application
Foundations of Intelligent Systems(2022)
摘要
We present a novel method for Document Layout Analysis that detects documents that are not compliant with a given template. The major challenge we solve is dealing with a highly unbalanced dataset with only a few, hard-to-distinguish, non-compliant documents. Our model learns to detect inadequate documents based on localised non-compliant characteristics, including stamps, handwritten text, and misplaced signatures. Nevertheless, the model must not report documents containing other artefacts such as amendments or notes, which we deem acceptable. We address these challenges via generative modelling, using anomaly detection techniques to validate document layout. In particular, we first let the model learn the compliant document distribution. Then, we detect and report out-of-distribution samples for their automated rejection. In the paper, we investigate and compare two major approaches to anomaly detection: 1) classifying anomalies as those samples that cannot be accurately generated by the model; and 2) detecting samples whose mapping to a known proxy distribution is not possible. Both methods can be trained without annotations and obtain a classification accuracy of
$$\sim $$
90% on real-world documents, outperforming alternative supervised solutions.
更多查看译文
关键词
Document validation, VAE, Anomaly detection, Unbalanced dataset, Document layout analysis, Deep learning
AI 理解论文
溯源树
样例
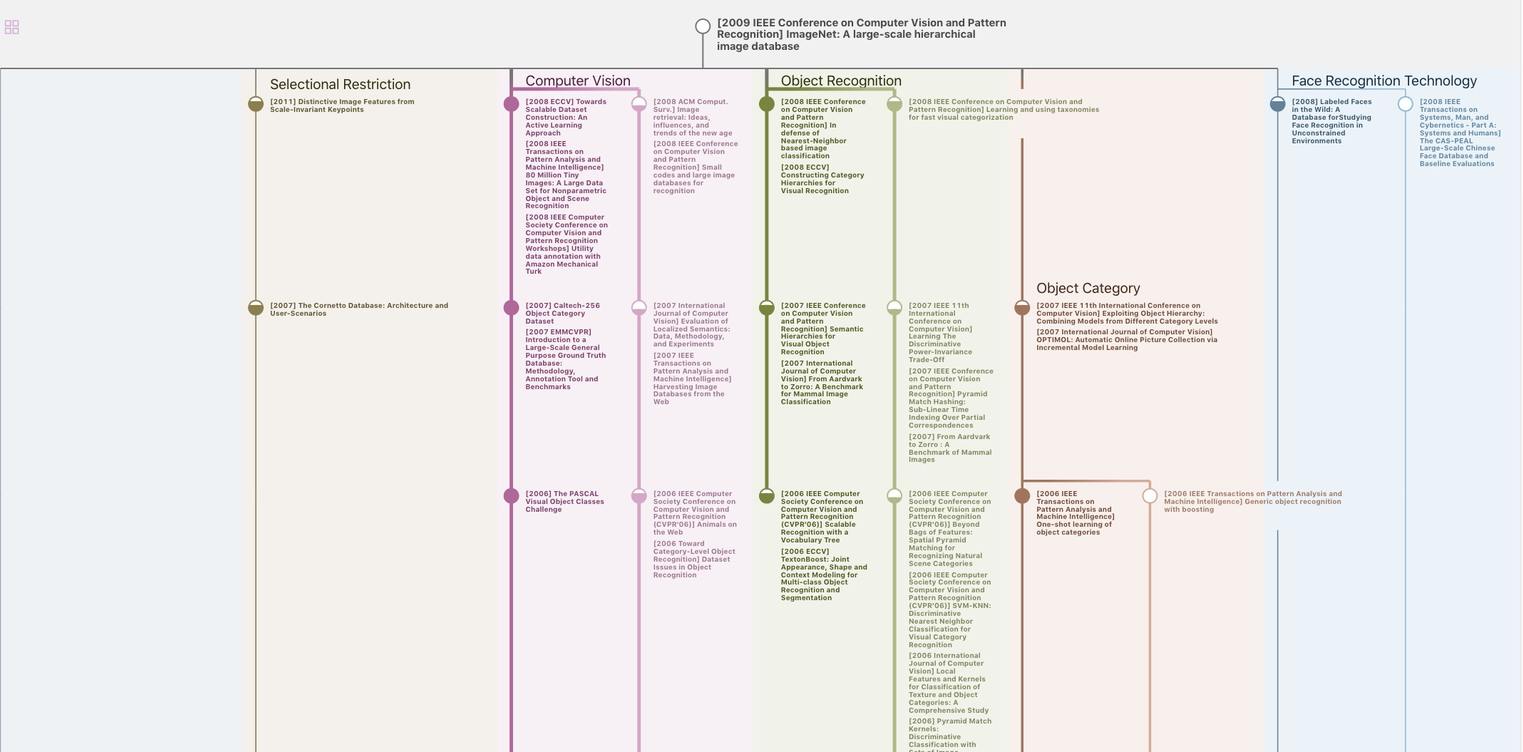
生成溯源树,研究论文发展脉络
Chat Paper
正在生成论文摘要