Articulatory Analysis and Classification of Pathological Speech
Proceedings of the 9th Conference on Sound and Music Technology(2022)
摘要
Unlike clinical practices, data analysis and speech technology (signal processing, and machine learning) have manifested many advantages to diagnose pathological speech. Due to this reason, this work has presented different methods of data analysis to compare dysarthria severity and healthy speech followed by the classification. The comparisons have been done based on the articulatory part of TORGO, a publicly dysarthric speech dataset. This study mainly compared the three-dimensional space, the tongue pronunciation range skewness, and the articulatory pronunciation time between dysarthria speech severity and control speech. In machine learning, the classification of the pathological database is important to diagnose the disease. However, the misclassification of the traditional algorithm on the data with more than two classes, worst when the sample numbers between classes are imbalanced is high. Due to that problem, a method is proposed in this paper by adopting both principal component analysis (PCA) and cost-sensitive (CS) with the J48 decision tree to reduce the misclassification and solve imbalanced data. The result of the proposed method both in overall accuracy and recall outperformed the original J48. This method is also compared against the two other imbalanced classification methods where it showed the best accuracy.
更多查看译文
关键词
Dysarthria, Pronunciation position, Time analysis, PCA, Cost-sensitive
AI 理解论文
溯源树
样例
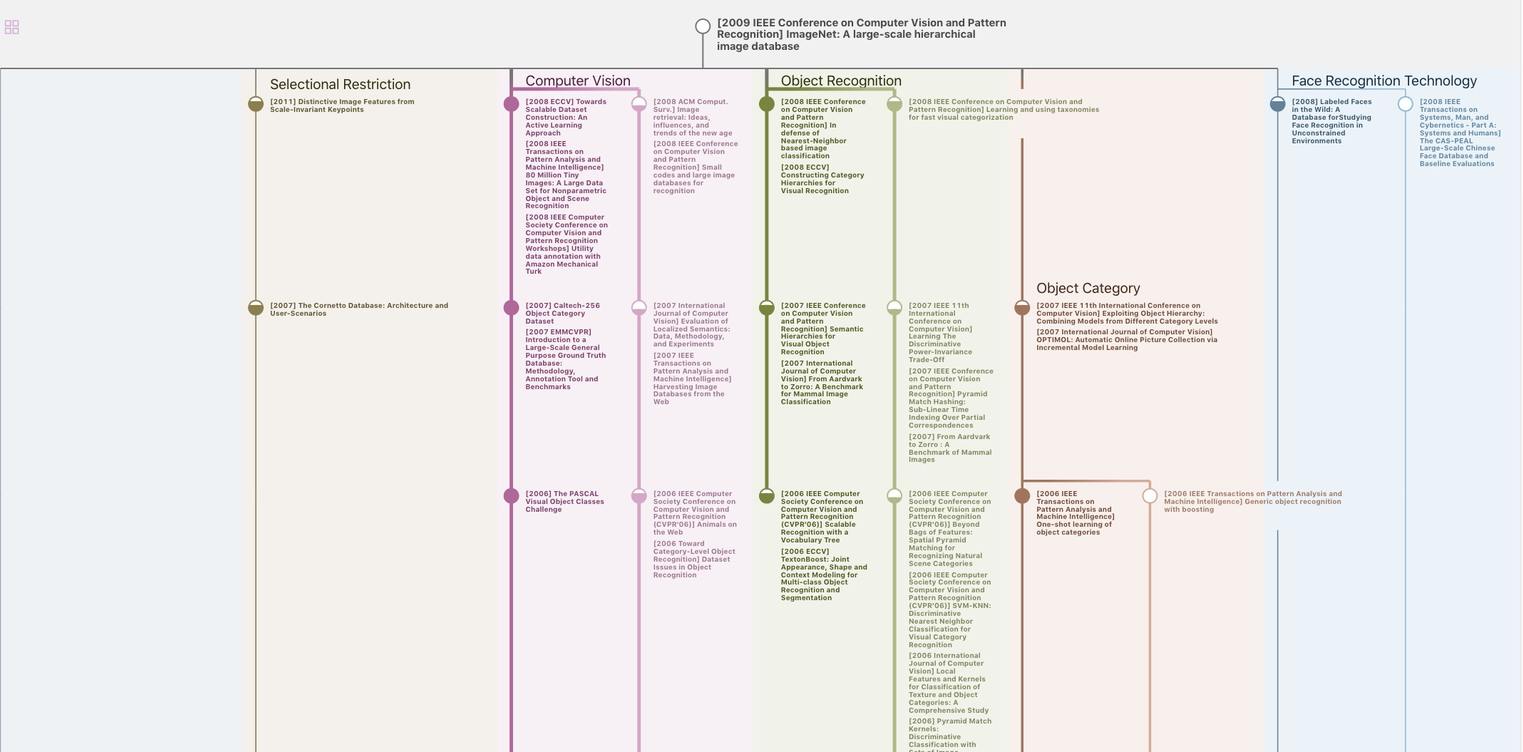
生成溯源树,研究论文发展脉络
Chat Paper
正在生成论文摘要