An Extreme Machine Learning Model for Evaluating Landslide Hazard Zonation in Nilgiris District, Causative Factors and Risk Assessment Using Earth Observation Techniques
Computational Methods and Data Engineering(2022)
摘要
In Nilgiris mountain regions, landslide is one of the most severe hazards, damages natural resources, and disturbs the ecosystem and environment. It creates a substantial loss to agriculture, forest, wealth and scratches major architectural constructions in the mountainous region. This proposed work aims to distinguish the range of the spatial vulnerability of landslide using ensembled machine learning models, including AdaBoost, random forest algorithms, and gradient boosting decision tree algorithms. Seven conditioning factors namely slope angle, slope aspect, land use, geomorphic features, distance from road, drainage density, and lineament density and a total of 272 landslide historical locations with a ratio of 70/30 were used to construct the spatial database. Estimate the efficacy of the ensembled models using evaluation metrics like precision, recall, F1-score, MAE, MCE, MSE, Kappa and AUC score. Results concluded that the land-use factor, vegetable crop area, is prone to high vulnerability zone in the study area. Additionally, results show that among all the ensembled machine learning models, the AdaBoost algorithm’s classification gives the highest accuracy value of 88.96%. However, the gradient boost decision classifier also outperformed with an accuracy value of 78.43%, and the random forest algorithm gives 72.61%.
更多查看译文
关键词
Landslide susceptibility mapping, Machine learning, AdaBoost algorithms, Receiver operating characteristics, Nilgiris
AI 理解论文
溯源树
样例
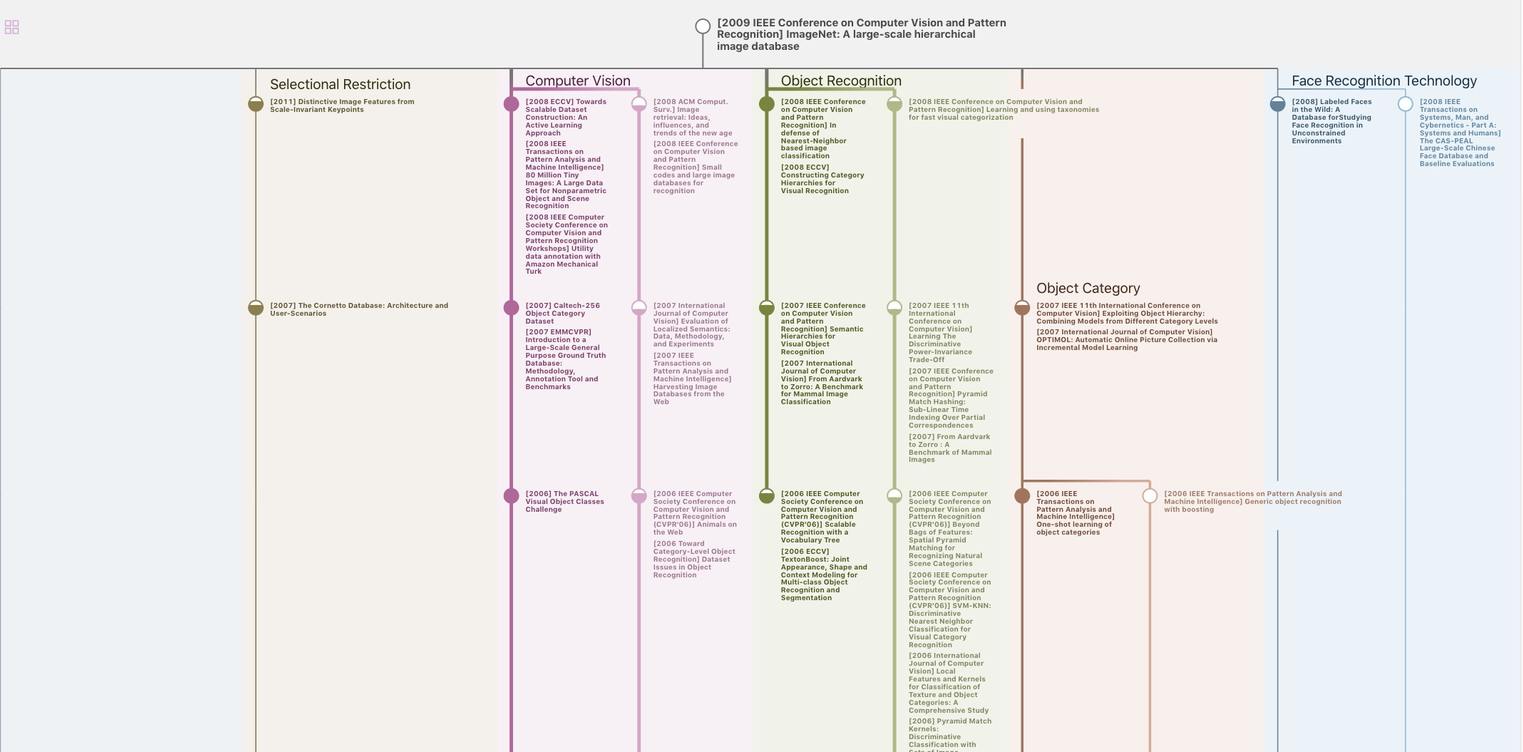
生成溯源树,研究论文发展脉络
Chat Paper
正在生成论文摘要