Vegetation vulnerability to hydrometeorological stresses in water-scarce areas using machine learning and remote sensing techniques
Ecological Informatics(2023)
摘要
The quantitative understanding of vegetation vulnerability as a major example of terrestrial ecosystems under hydrometeorological stress is essential for environmental risk preparedness and mitigation strategies. The aim of this study was to develop a new quantitative vegetation vulnerability map using benchmark and standalone machine learning (ML) algorithms (e.g., RF, SVM and Maxent), as well as influencing variables (evaporation, rainfall, maximum temperature, slope degree, elevation, topographic wetness index, distance from river, aspect, land use), in the South Baluchistan basin, Iran. An ensemble model was developed based on selected standalone ML algorithms. A vegetation vulnerability index (VVI), based on remote sensing indices (NDVI, VCI, LST, and TCI), was used to monitor vegetation conditions and changes. Five evaluation metrics for the confusion matrix (accuracy, precision, bias, Probability of Detection (POD), False Alarm Ratio (FAR)) and ROC-AUC were used to measure the predictive performance of the ensemble model and VVI. The optimum values for accuracy, precision, bias, POD, FAR, and ROC-AUC were obtained as 0.89, 0.88, 1.02, 0.91, 0.11, and 0.946, respectively for the ensemble model. Based on remote sensing data, VVI achieved a 0.923 prediction rate in vegetation vulnerability mapping (the efficiency of the ensembled model was somewhat better than VVI). Based on the results obtained from the ensemble model, precipitation (PRD = 20.61), maximum temperature (PRD = 12.31), evaporation (PRD = 5.53), and distance from the river (PRD = 2.62) were found to be the most important variables. The methodology as presented in this study provides valuable information in a large area and can be easily modified for other case studies by adding different influencing variables.
更多查看译文
关键词
Vegetation vulnerability,Ensemble model,Probability of detection,False alarm ratio
AI 理解论文
溯源树
样例
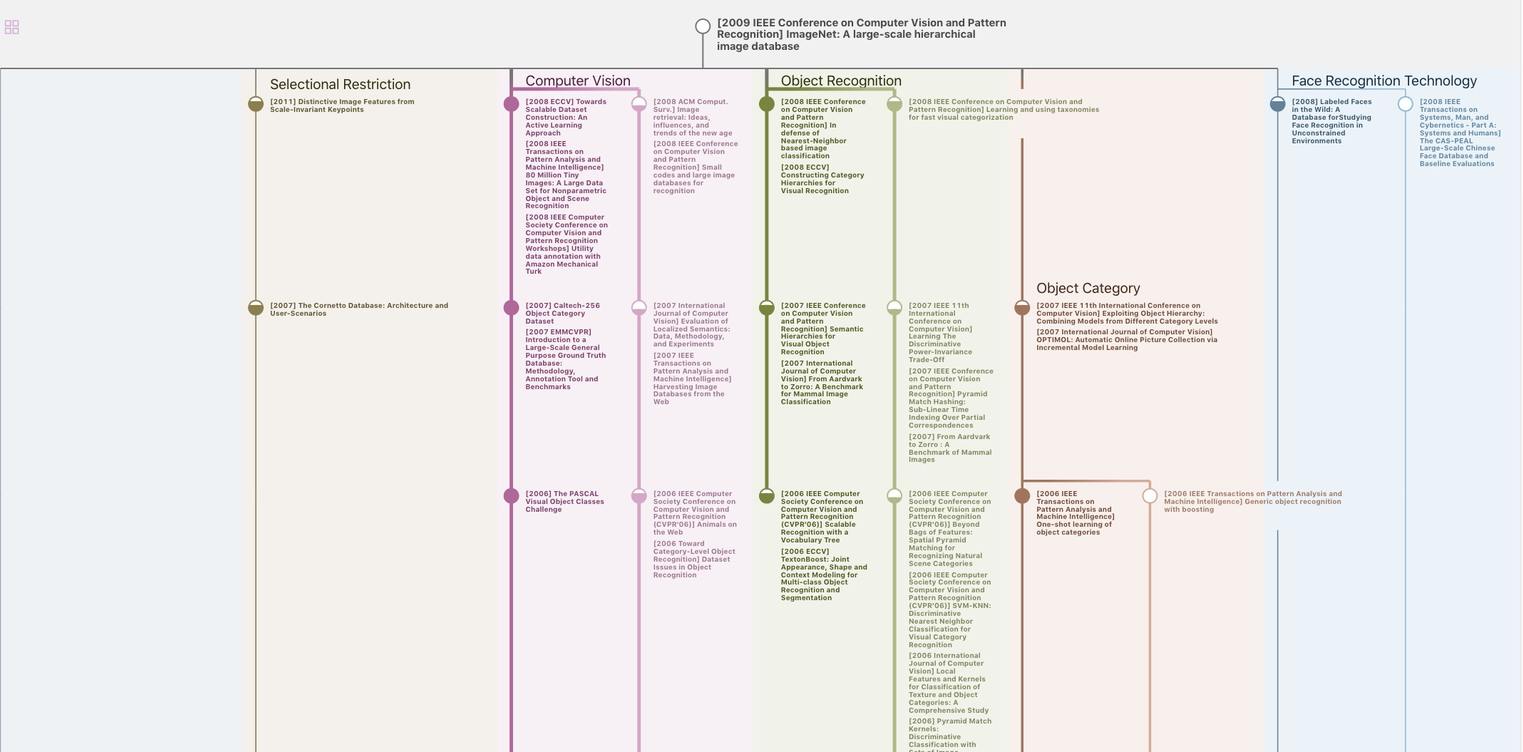
生成溯源树,研究论文发展脉络
Chat Paper
正在生成论文摘要