Wind Power Forecast Based on Transformers and Clustering of Wind Farms with Temporal and Spatial Interdependence
IEEE International Joint Conference on Neural Network (IJCNN)(2022)
摘要
Wind power forecasting remains a challenging area of research due to the complex relationships present in the conversion of wind into electrical power and the uncertainty associated with the physical modeling of the wind, which is highly dependent on the initial conditions. The need for a precise forecast is essential to meet the goals of high integration of renewable sources in the energy matrix to optimize non-renewable resources and improve the reliability in the operation of the electric system. In this work, we applied a wind power forecasting model based on Transformers that uses a self-attention encoder and a seq-2-seq decoder with global attention to capturing the nonlinear relationships of the power time series of wind farms. This evaluation considers the behavior of groups of wind farms with similar behavior. In terms of the similarity metric, five distant and close wind farms are clustered by the k-means method, using the autocorrelation features for time lags from 3 to 24 hours, mean and standard deviation, selected among 447 wind farms located in the NE region of Brazil. Compared to traditional linear autoregressive and LSTM models, the results show a lower prediction error. In this way, the model can capture temporal correlations arising from autoregressive and spatial behaviors. This shows the interdependence between hundreds of wind farms spread over a vast region with high wind potential, different altitudes, orography, distance from the coast, and wind regimes.
更多查看译文
关键词
Wind power,transformer,clustering,time series
AI 理解论文
溯源树
样例
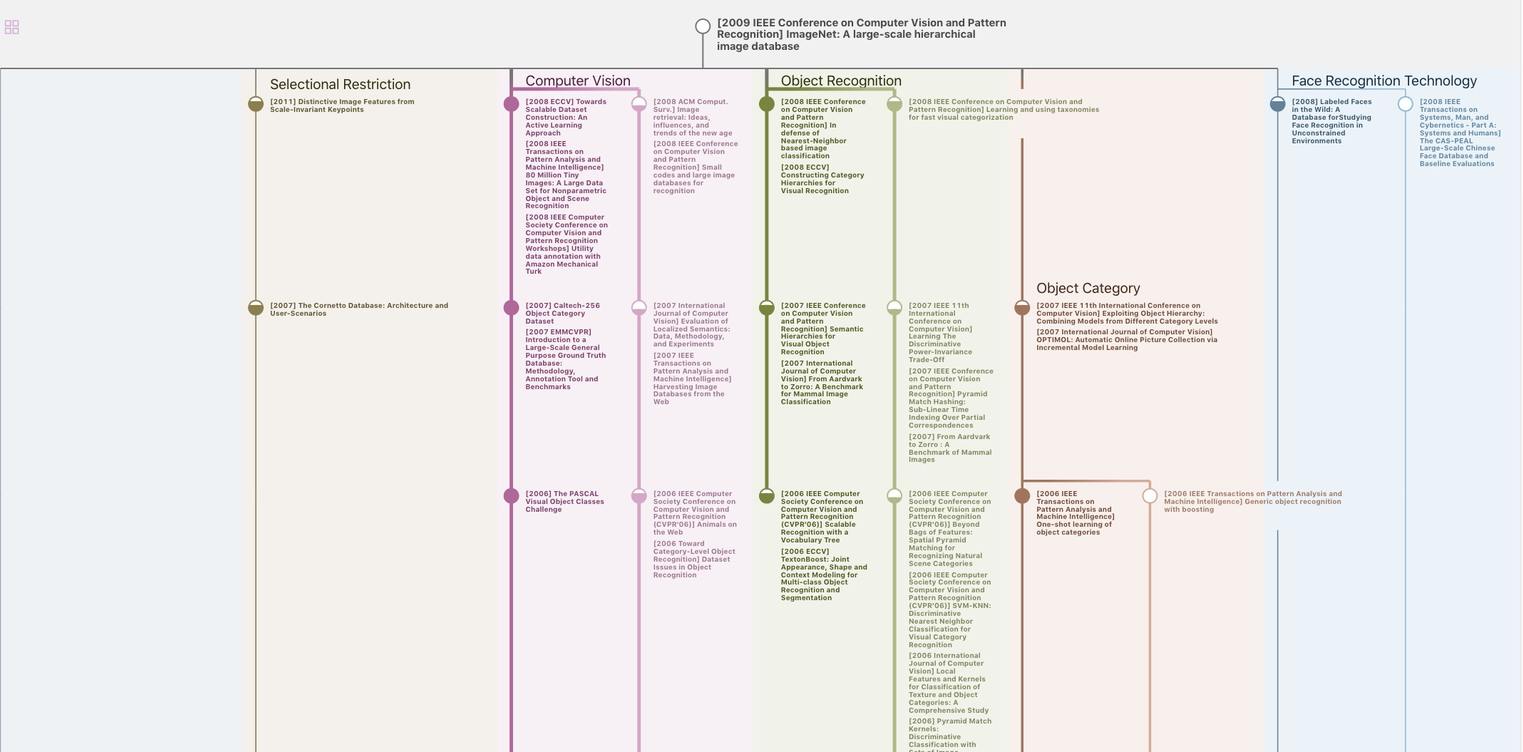
生成溯源树,研究论文发展脉络
Chat Paper
正在生成论文摘要