A data-centric approach for predicting individual outcomes in a multi-party legislative system
IEEE International Joint Conference on Neural Network (IJCNN)(2022)
摘要
Congressional roll-call voting is highly predictable in strong two-party systems, and simple spatial voting models are sufficient to explain most of individual voting choices. Such spatial predictability is mainly due to the high degree of ideological stability exhibited by members of the same party. In multi-party legislative systems even if we also find a high degree of party discipline, individuals can be more susceptible to defection. This is a more complex and harder to monitor environment, and as such, models that predict "yea" or "nay" votes are the need of the hour for advocacy groups. In order to learn effective prediction models, the training data must entail diverse aspects of the modus-operandi of the congress. In this paper we discuss a data-centric approach for the problem, with emphasis on the features we built. Each congressperson is represented by a diverse set of features which are computed using linguistic models, causal models, and structured information. Finally, we show that meta-features derived from feature importance information lead to highly effective prediction models.
更多查看译文
关键词
advocacy,prediction models,data-centric approaches
AI 理解论文
溯源树
样例
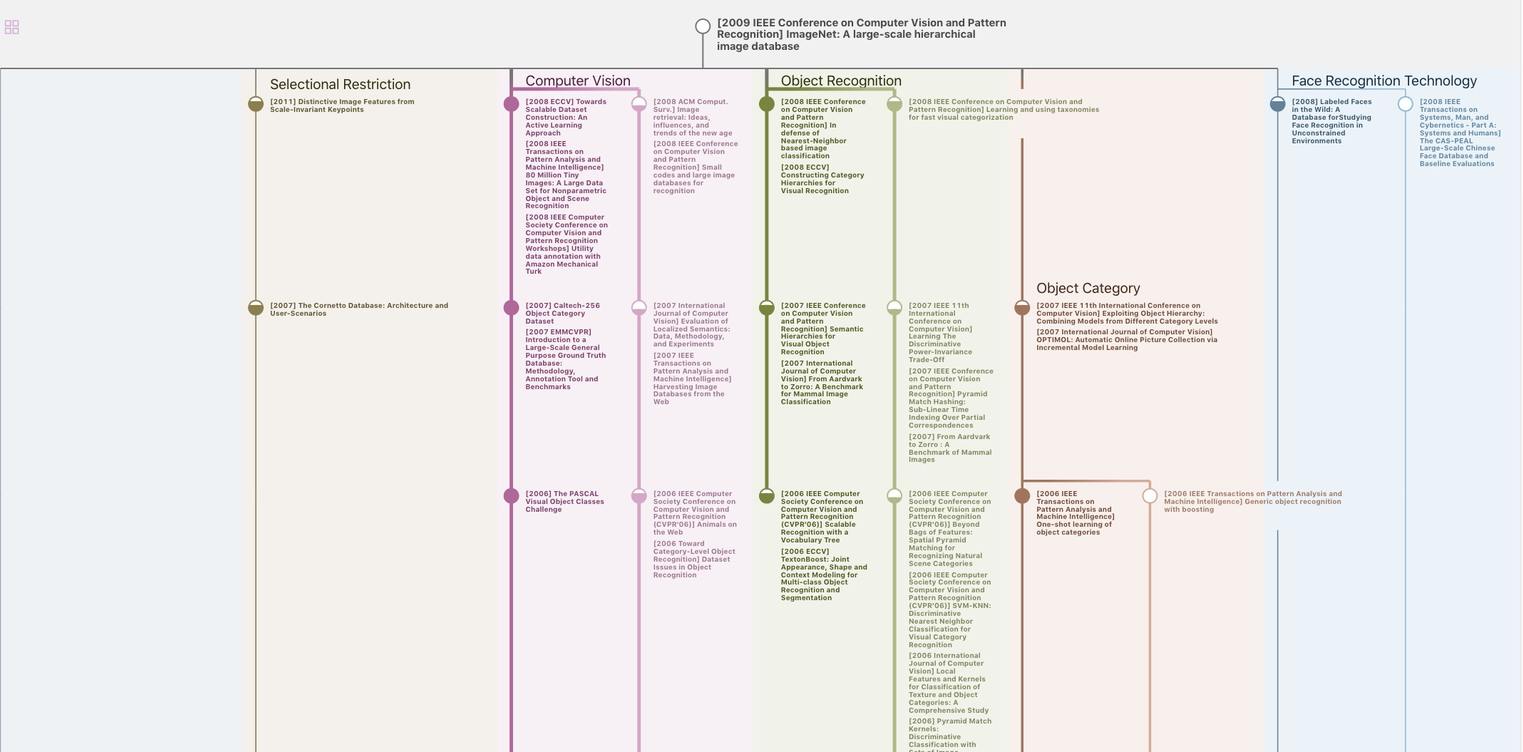
生成溯源树,研究论文发展脉络
Chat Paper
正在生成论文摘要