Graph Convolution over the Semantic-syntactic Hybrid Graph Enhanced by Affective Knowledge for Aspect-level Sentiment Classification
2022 International Joint Conference on Neural Networks (IJCNN)(2022)
摘要
Aspect-level sentiment classification (ASC), detecting and predicting the sentiment polarity of the given aspects, has attracted increasing attention in the field of Natural Language Processing (NLP). Recent studies in ASC leveraged the graph based on the dependency tree of the context to incorporate the syntactic information and structure of a sentence for better relation extraction. Some researchers noted that existing methods ignored semantic relations or failed to consider affective dependency information, and then proposed several state-of-art methods tackling the above two limitations. However, these approaches failed to consider both informative relations simultaneously. Therefore, we explore and propose a novel solution based on semantic latent graph and SenticNet to leverage semantic and affective information. Specifically, we build a latent semantic graph based on self-attention networks to parse semantic relations within the contexts. In addition, we utilize affective knowledge from SenticNet to enhance the dependency graphs of sentences. Moreover, we use the gate mechanism to dynamically combine information from both the enhanced dependency graphs and latent semantic graphs. Experimental results on three benchmark datasets illustrate the effectiveness and state-of-the-art performance of our model.
更多查看译文
关键词
Aspect-level sentiment classification,semantic relations,affective knowledge,Graph convolutional networks
AI 理解论文
溯源树
样例
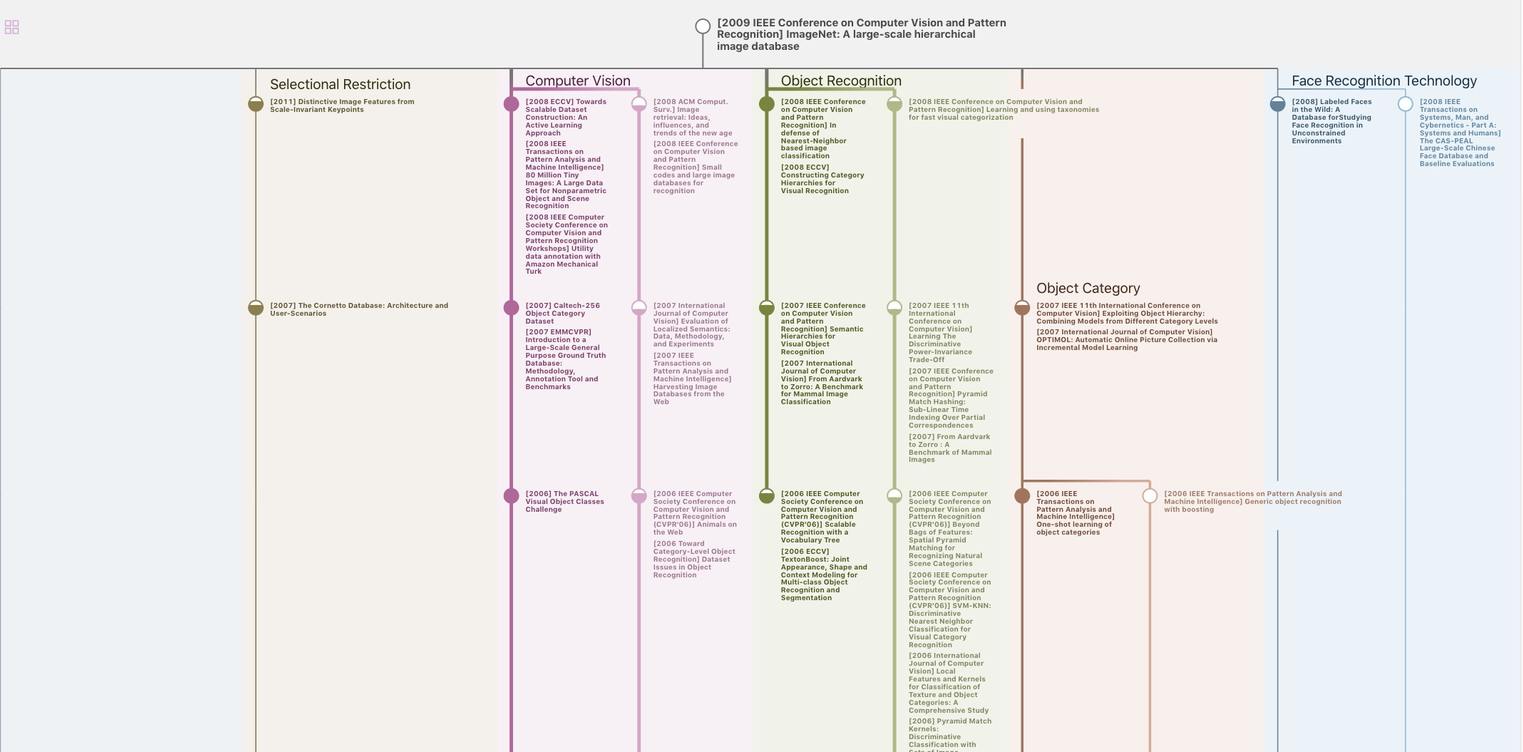
生成溯源树,研究论文发展脉络
Chat Paper
正在生成论文摘要