Interpretable Binaural Ratio for Visually Guided Binaural Audio Generation.
IEEE International Joint Conference on Neural Network (IJCNN)(2022)
摘要
Video and audio streams are essential and mutually complementary in multimedia immersive application scenarios. Recent studies have explored the field of deep neural network application on multimedia production, e.g., visually guided generation of binaural audio, where Difference Mask (DM) is the predominant strategy in the state-of-the-art (SOTA) work. However, this strategy is not interpretable and requires adding the ground truth output as the input, limiting applicability. Besides, the generated audio has a relatively low spatial sensation. This paper aims to develop an interpretable and robust approach to visually guided binaural audio generation. Specifically, we generalize a concept and new strategy from Difference Mask, named Binaural Ratio, to interpret its binaural property relevant to the Inter-aural Time Difference (ITD) and Inter-aural Level Difference (ILD). In the new strategy, the model input can be natural and arbitrary mono audio instead of the direct sum of left and right audio, i.e., ground truth output. Moreover, we identify that one reason for the low spatial sensation is the bias toward mono. Thus, we tackle it by designing new network variants to learn the Binaural Ratio robustly. Experiments show that our proposed approach significantly outperforms the SOTA methods in both objective and subjective evaluation metrics.
更多查看译文
关键词
spatial audio,self-supervised learning,cross-modality
AI 理解论文
溯源树
样例
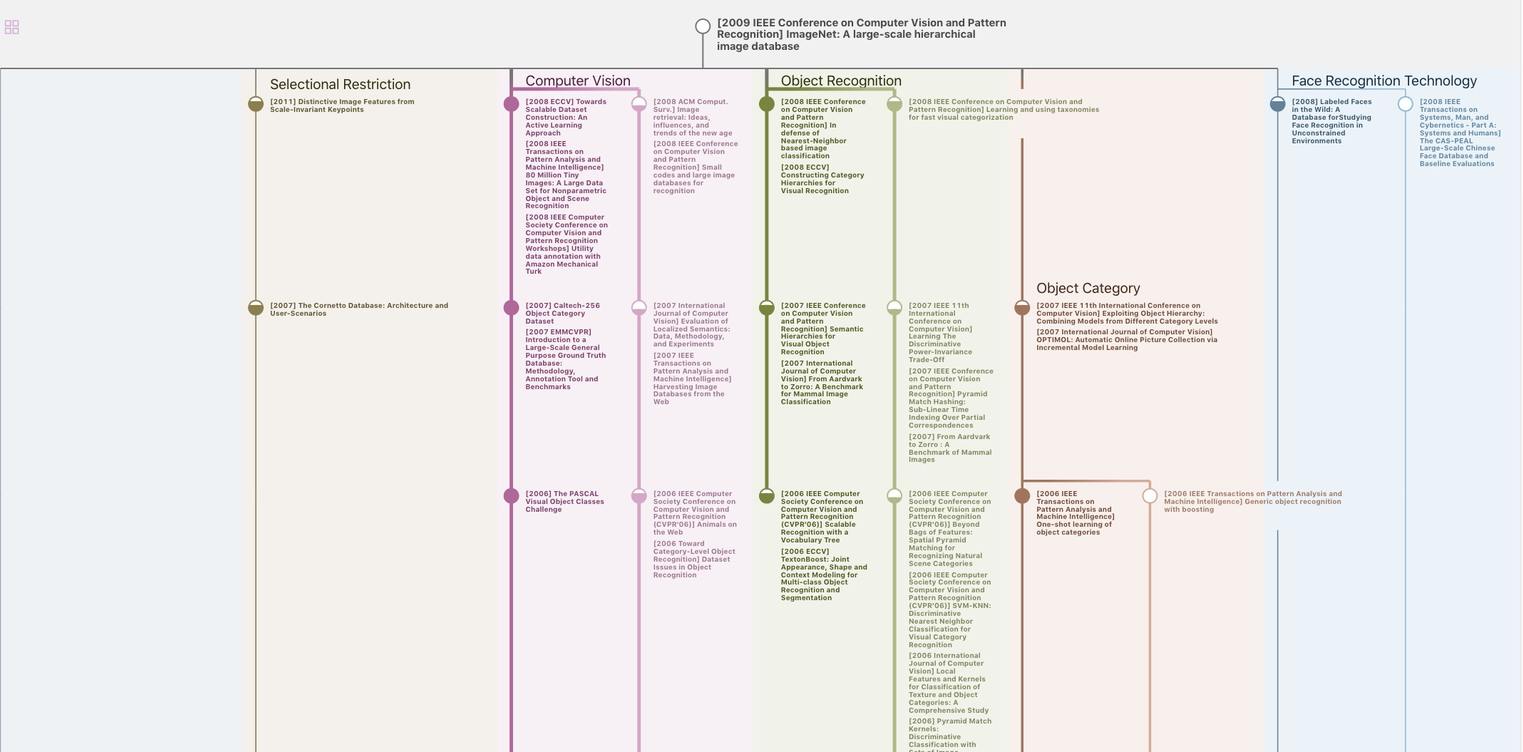
生成溯源树,研究论文发展脉络
Chat Paper
正在生成论文摘要