Atrial Fibrillation Classification and Prediction Explanation using Transformer Neural Network
IEEE International Joint Conference on Neural Network (IJCNN)(2022)
摘要
Deep learning models are ubiquitous for detecting cardiac abnormalities from Electrocardiogram (ECG) signals. Atrial Fibrillation (AFib) is one such abnormality that increases the risk of stroke, coronary mortality and is difficult to monitor in real-time due to its intermittent occurrence. This paper investigates Transformer Neural Network (TNN) for AFib detection and justifies the prediction by highlighting clinically relevant signal timestamps triggering the diagnosis. TNN captures local and global dependencies of ECG in a parameter-efficient way and achieves an average F1 score of 0.87 on the PhysioNet Computing in Cardiology Challenge 2017 database. For comparison, a baseline and attentive convolution neural network (ACNN), residual neural network are also investigated that achieve an F1 score of 0.76, 0.73, and 0.95, respectively, surpassing state-of-the-art methods. The effect of ECG segment length is also analyzed to precisely detect AFib rhythm in larger ECG segments. The ACNN and TNN explain the predictions by highlighting absent P-wave and Fibrillation waves in ECG, supporting the diagnosis. The visual explanations improve model assessment, increases transparency and the likelihood of model acceptance by the medical practitioners.
更多查看译文
关键词
Interpretation,Transformer Neural Network,Attention Mechanism,Convolutional Neural Networks,Atrial Fibrillation,Electrocardiogram,Residual Neural Network
AI 理解论文
溯源树
样例
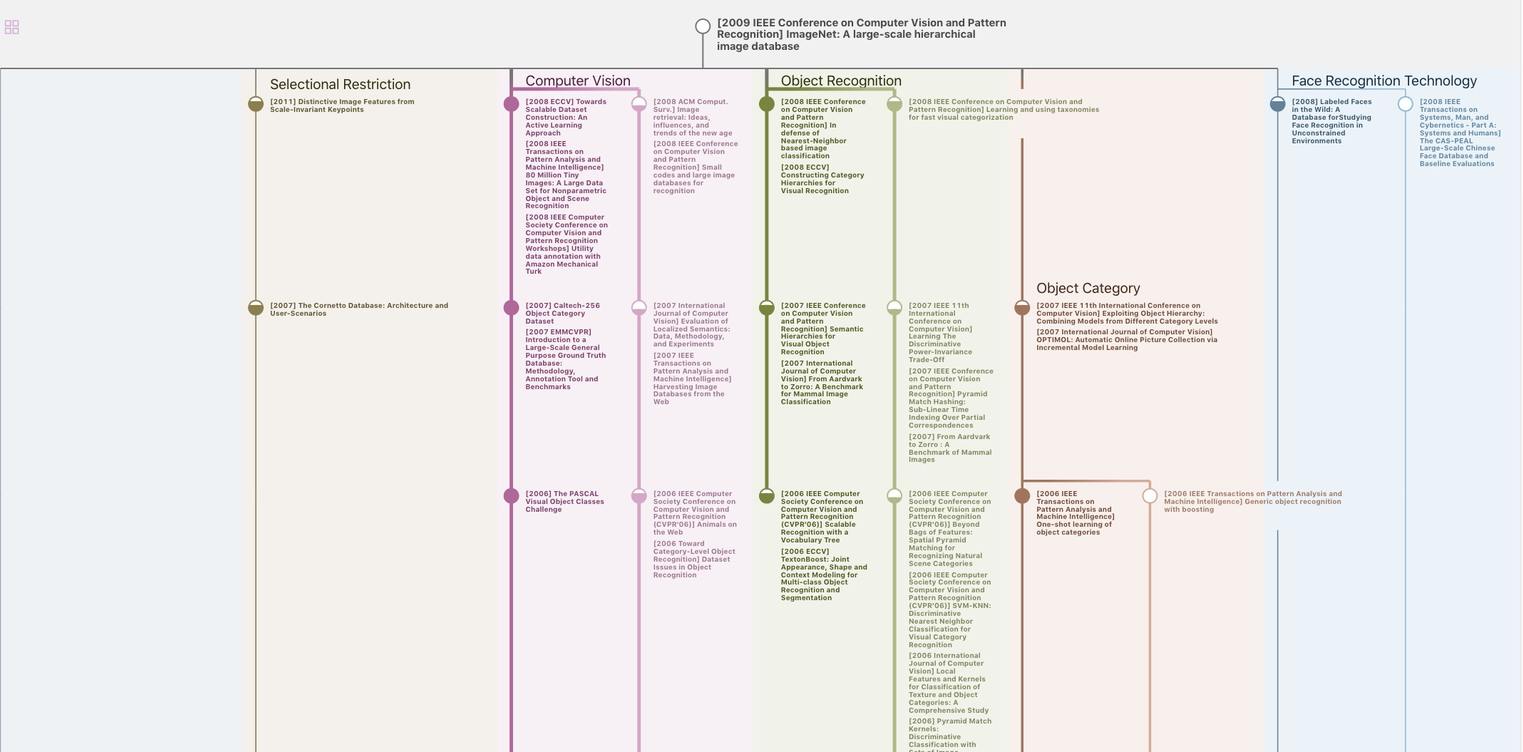
生成溯源树,研究论文发展脉络
Chat Paper
正在生成论文摘要