DISNet: A sequential learning framework to handle occlusion in human action recognition with video acquisition sensors.
Digit. Signal Process.(2022)
摘要
Human action recognition (HAR) requires a clear line of sight for the video acquisition system to properly collect the data. However, the data acquisition is hampered due to presence of the obstructions in front of the action happening in real time environment. In recently reported HAR techniques, the widely used multi-stream sequential networks are having two major limitations. First, the performance of the existing algorithms degrades comprehensively in the presence of partial loss of action data due to obstruction. Second, the performance of the existing networks is restricted by not exploiting the dependency relationship between different streams of a multi-stream network. To handle these problems, a novel double input sequential network (DISNet) is proposed which takes care the HAR algorithm in the presence of partial loss of action data. The DISNet, which learns inter-stream information, is jointly trained on the normal data and the artificially created obstructed data of a single video to provide immunity to the HAR network against obstructions. The proposed DISNet is evaluated on publicly available datasets like KTH, UCF-sports, JHMDB and UCF101, which provides performance accuracy of 83.57%, 82.27%, 50.24% and 54.96% respectively.(c) 2022 Elsevier Inc. All rights reserved.
更多查看译文
关键词
Action recognition,BiLSTM,Double input sequential network,Joint training
AI 理解论文
溯源树
样例
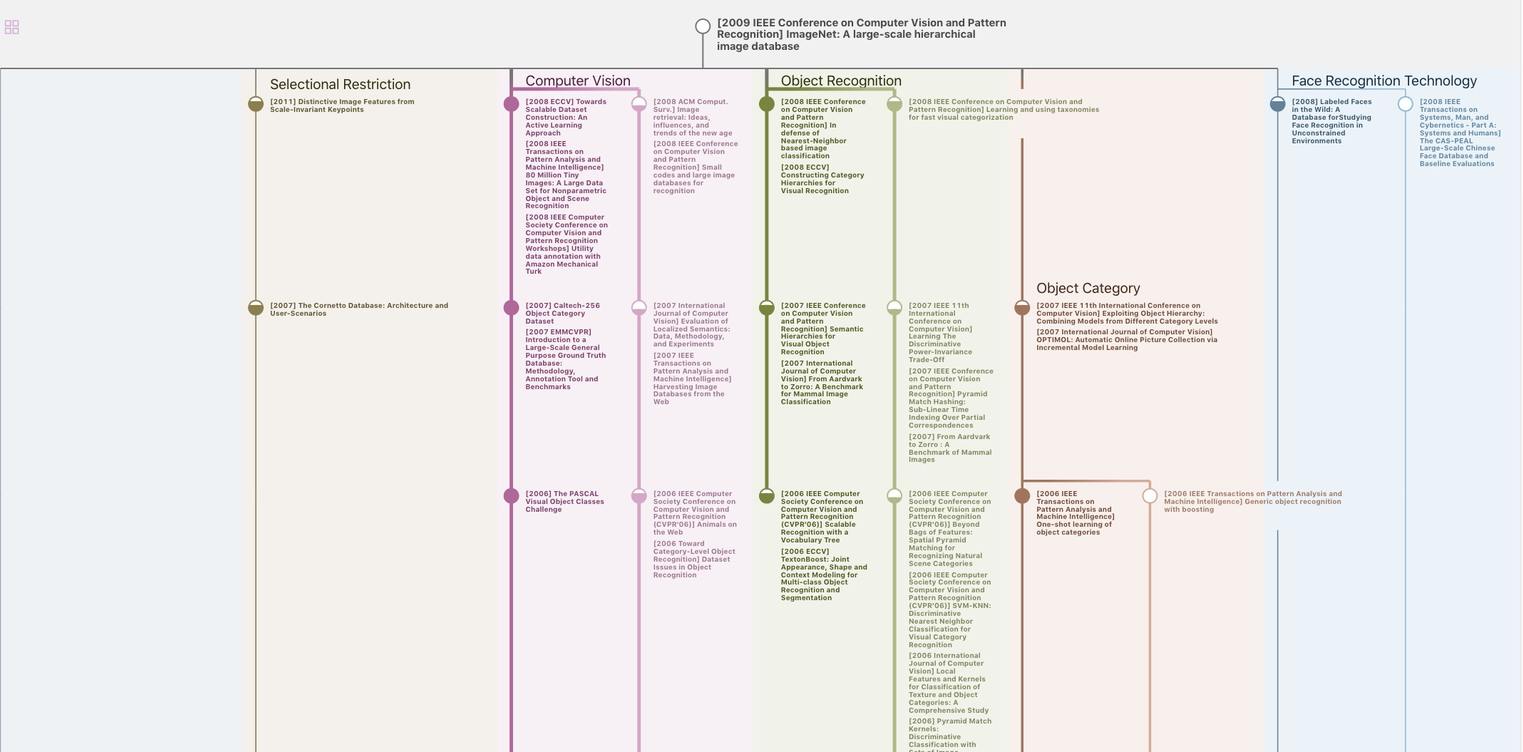
生成溯源树,研究论文发展脉络
Chat Paper
正在生成论文摘要