DiGress: Discrete Denoising diffusion for graph generation
ICLR 2023(2022)
摘要
This work introduces DiGress, a discrete denoising diffusion model for generating graphs with categorical node and edge attributes. Our model defines a diffusion process that progressively edits a graph with noise (adding or removing edges, changing the categories), and a graph transformer network that learns to revert this process. With these two ingredients in place, we reduce distribution learning over graphs to a simple sequence of classification tasks. We further improve sample quality by proposing a new Markovian noise model that preserves the marginal distribution of node and edge types during diffusion, and by adding auxiliary graph-theoretic features derived from the noisy graph at each diffusion step. Finally, we propose a guidance procedure for conditioning the generation on graph-level features. Overall, DiGress achieves state-of-the-art performance on both molecular and non-molecular datasets, with up to 3x validity improvement on a dataset of planar graphs. In particular, it is the first model that scales to the large GuacaMol dataset containing 1.3M drug-like molecules without using a molecule-specific representation such as SMILES or fragments.
更多查看译文
关键词
Graph generation,Denoising Diffusion Model,Molecule Generation,Permutation Equivariance,Discrete Diffusion
AI 理解论文
溯源树
样例
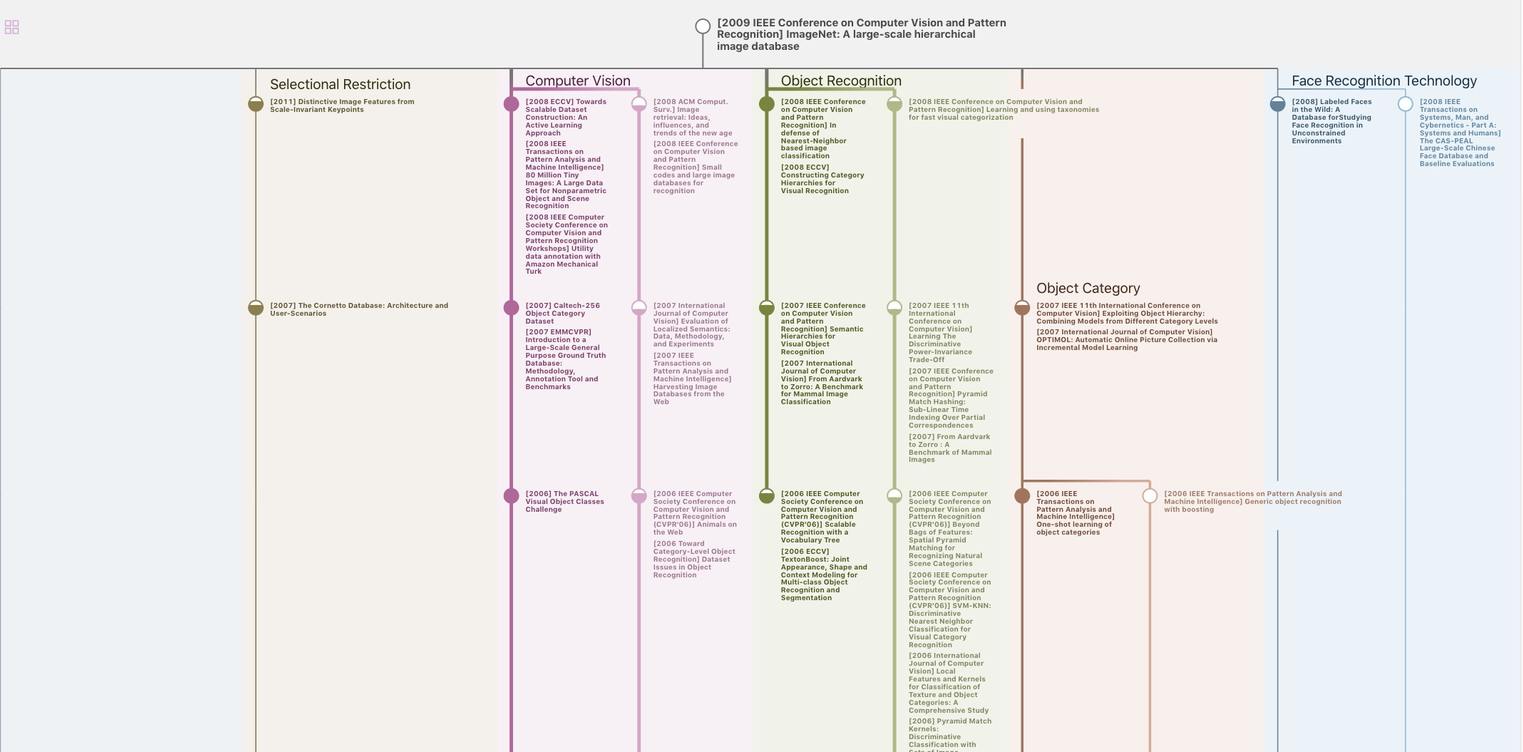
生成溯源树,研究论文发展脉络
Chat Paper
正在生成论文摘要