Convolutional neural network-based homogenization for constructing a long-term global surface solar radiation dataset
Renewable and Sustainable Energy Reviews(2022)
摘要
Rapid development of photovoltaics industry emphasizes the importance of a long-term reliable dataset of surface solar radiation (Rs). However, long-term satellite-retrievals usually suffer from inhomogeneity due to the inhomogeneous input data from different satellites. Atmospheric reanalysis Rs data have stable quality but relatively lower accuracy. In this study, a convolutional neural network-based homogenization method is developed to use a reanalysis dataset (ERA5) as a bridge to homogenize a 36-year (1983–2018) satellite-based Rs dataset (so-called ISCCP-ITP) with 10-km spatial resolution and 3-hr interval, which has high accuracy after 2000 (monthly RMSE of 16.9 W/m2 and MBE of 0.2 W/m2) but relatively lower accuracy before 2000 (monthly RMSE of 18.2 W/m2 and MBE of 7.2 W/m2). The method is based on U-Net algorithm, which contains encoder, decoder and skipped connection parts. Monthly Rs of ISCCP-ITP and ERA5 after 2000 are used as label data and input data for the training, respectively, and monthly Rs of ERA5 before 2000 is fed into the trained model to generate homogenization results. After homogenization, the RMSE and MBE of the monthly ISCCP-ITP data are reduced to 15.8 W/m2 and -1.6 W/m2, respectively, before 2000, which are comparable to those after 2000, making the accuracy of the new dataset stable throughout the whole period. Moreover, the variations and trends of Rs derived from the new data are consistent with in-situ observations at global and regional scales. Therefore, the homogenization can contribute to the re-construction of a high-quality long-term Rs dataset suitable for photovoltaics industry.
更多查看译文
关键词
Satellite-based solar radiation,Atmospheric reanalysis dataset,Inhomogeneity,Homogenization,Convolutional neural network,Radiation trend
AI 理解论文
溯源树
样例
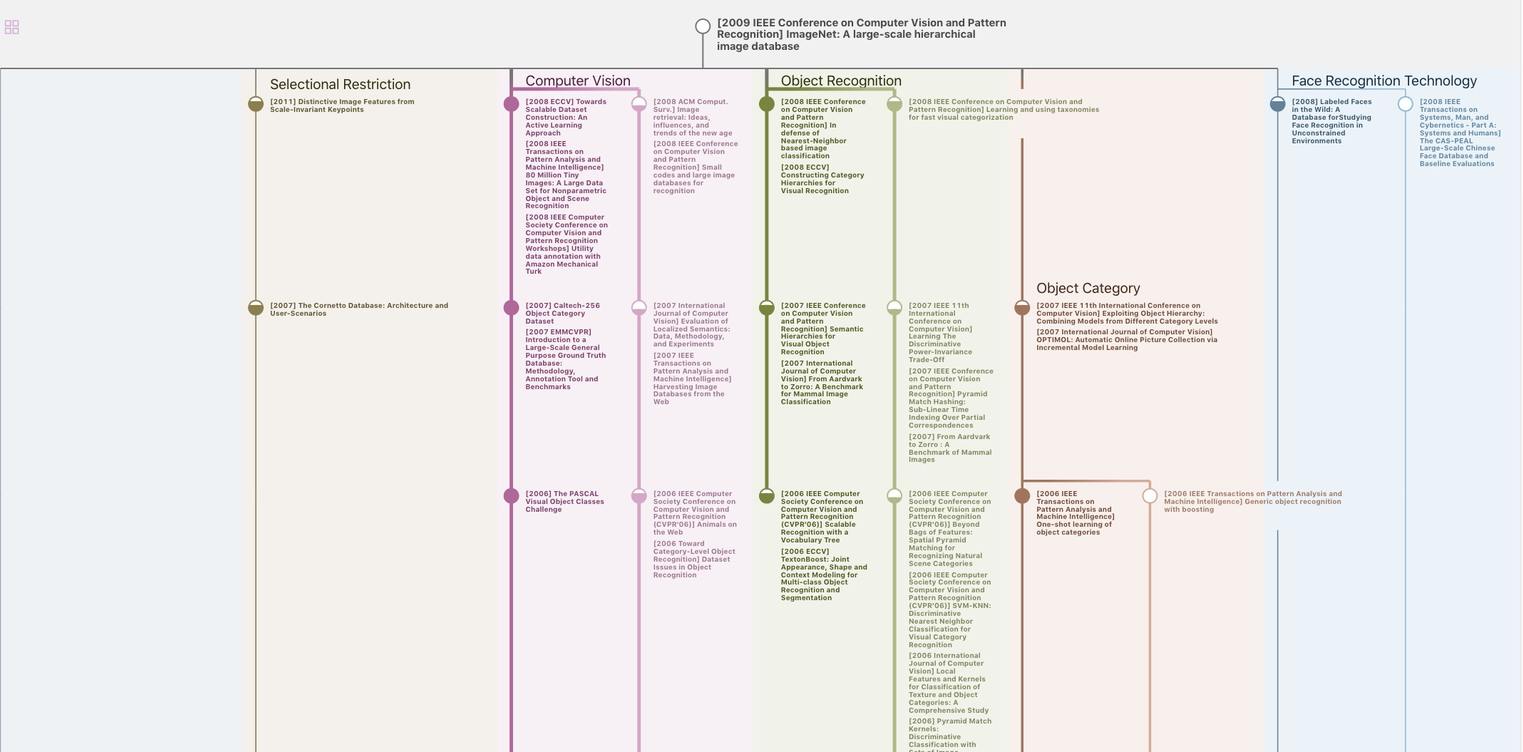
生成溯源树,研究论文发展脉络
Chat Paper
正在生成论文摘要