A multi-head self-attention deep learning approach for detection and recommendation of neuromagnetic high frequency oscillations in epilepsy
FRONTIERS IN NEUROINFORMATICS(2022)
摘要
Magnetoencephalography is a noninvasive neuromagnetic technology to record epileptic activities for the pre-operative localization of epileptogenic zones, which has received increasing attention in the diagnosis and surgery of epilepsy. As reported by recent studies, pathological high frequency oscillations (HFOs), when utilized as a biomarker to localize the epileptogenic zones, result in a significant reduction in seizure frequency, even seizure elimination in around 80% of cases. Thus, objective, rapid, and automatic detection and recommendation of HFOs are highly desirable for clinicians to alleviate the burden of reviewing a large amount of MEG data from a given patient. Despite the advantage, the performance of existing HFOs rarely satisfies the clinical requirement. Consequently, no HFOs have been successfully applied to real clinical applications so far. In this work, we propose a multi-head self-attention-based detector for recommendation, termed MSADR, to detect and recommend HFO signals. Taking advantage of the state-of-the-art multi-head self-attention mechanism in deep learning, the proposed MSADR achieves a more superior accuracy of 88.6% than peer machine learning models in both detection and recommendation tasks. In addition, the robustness of MSADR is also extensively assessed with various ablation tests, results of which further demonstrate the effectiveness and generalizability of the proposed approach.
更多查看译文
关键词
high frequency oscillations (HFOs), magnetoencephalography, MEG, deep learning, multi-head self-attention, HFOs detection, HFOs recommendation
AI 理解论文
溯源树
样例
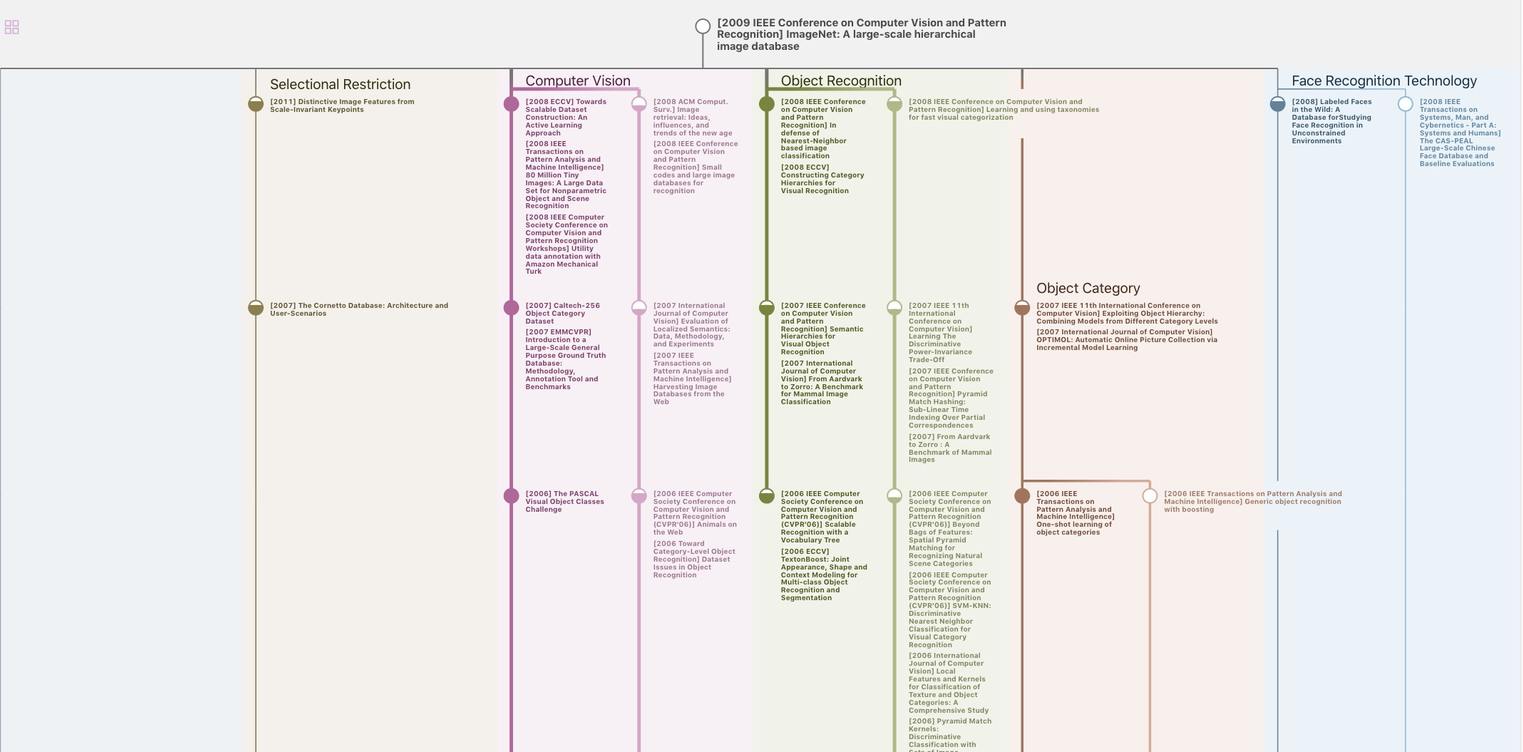
生成溯源树,研究论文发展脉络
Chat Paper
正在生成论文摘要