A Deep Learning Method for Floodwater Depth Prediction on Roadways from Side-View Real and Synthetic Images of Vehicles
Journal of Big Data Analytics in Transportation(2022)
摘要
This work proposes a novel deep learning method to predict depth of floodwater on streets based on side-view images of vehicles. Finding flood levels on roadways is useful for various applications including routing of emergency vehicles and safe traffic management and operations. The proposed approach consists of four main steps: semantic segmentation, object detection, segmentation refinement, and water depth calculation. The semantic segmentation step segments the flood scene to floodwater, wheels, and background classes; object detection step detects tires of the vehicles; and segmentation refinement step improves the initial segmentation result. Water depth is then calculated by fitting a line to the water edges on each tire and fitting an ellipse to each wheel on the vehicle. Finally, the water depth is estimated relative to the wheel size without knowing the actual wheel and tire dimensions which may vary from vehicle to vehicle. If the actual vehicle wheel dimensions are known, which can be inferred from the vehicle make/model, the proposed method is expected to offer more accurate water depth estimation. This method is trained using both real-world flooding images curated from web search and synthetic images from flooded city streets generated using the Unity software. We use only the real-world images in validation and test processes to tune the models and measure their performance with real-world examples. Overall, the proposed method achieves 0.038 mean absolute error (MAE) on the test set with 45 real-world images.
更多查看译文
关键词
Deep learning,Flooding,Floodwater depth prediction,Traffic management,Computer vision,Fully connected convolutional neural network
AI 理解论文
溯源树
样例
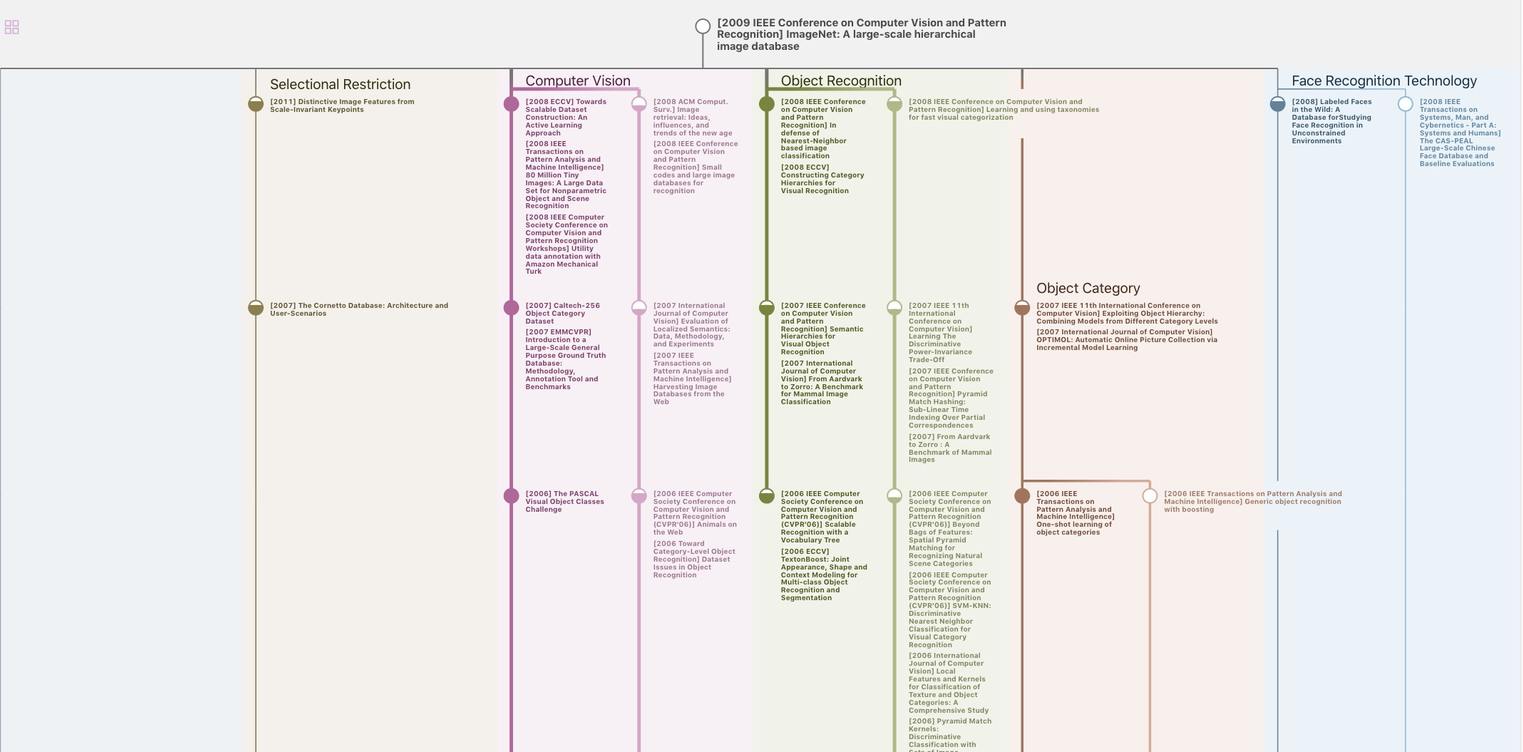
生成溯源树,研究论文发展脉络
Chat Paper
正在生成论文摘要