Joint Speech Activity and Overlap Detection with Multi-Exit Architecture
arxiv(2022)
摘要
Overlapped speech detection (OSD) is critical for speech applications in scenario of multi-party conversion. Despite numerous research efforts and progresses, comparing with speech activity detection (VAD), OSD remains an open challenge and its overall performance is far from satisfactory. The majority of prior research typically formulates the OSD problem as a standard classification problem, to identify speech with binary (OSD) or three-class label (joint VAD and OSD) at frame level. In contrast to the mainstream, this study investigates the joint VAD and OSD task from a new perspective. In particular, we propose to extend traditional classification network with multi-exit architecture. Such an architecture empowers our system with unique capability to identify class using either low-level features from early exits or high-level features from last exit. In addition, two training schemes, knowledge distillation and dense connection, are adopted to further boost our system performance. Experimental results on benchmark datasets (AMI and DIHARD-III) validated the effectiveness and generality of our proposed system. Our ablations further reveal the complementary contribution of proposed schemes. With $F_1$ score of 0.792 on AMI and 0.625 on DIHARD-III, our proposed system outperforms several top performing models on these datasets, but also surpasses the current state-of-the-art by large margins across both datasets. Besides the performance benefit, our proposed system offers another appealing potential for quality-complexity trade-offs, which is highly preferred for efficient OSD deployment.
更多查看译文
关键词
AMI dataset,dense connection,DIHARD-III dataset,frame level,high-level features,joint speech activity-overlap detection,joint VAD,knowledge distillation,low-level features,multiexit architecture,multiparty conversion,OSD problem,standard classification problem,three-class label
AI 理解论文
溯源树
样例
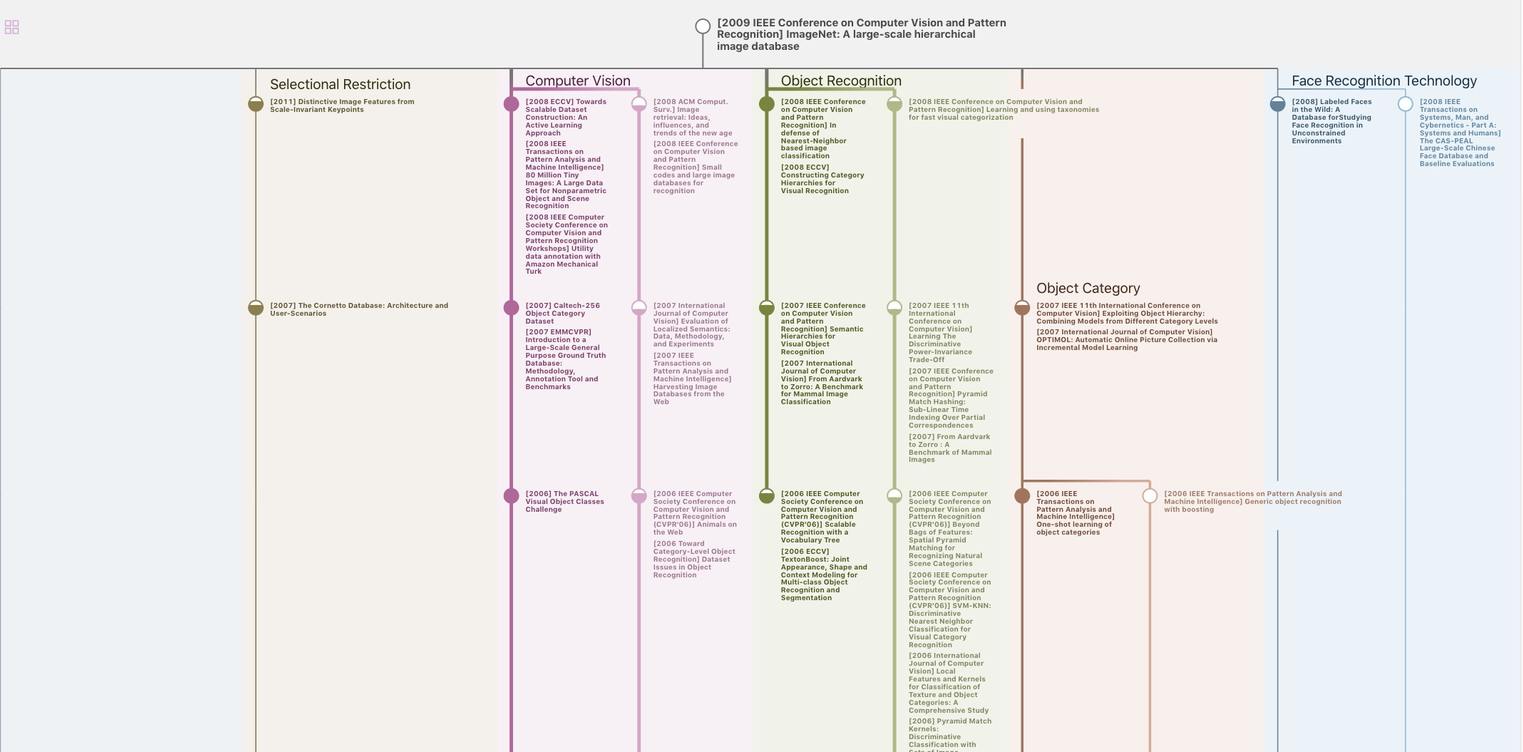
生成溯源树,研究论文发展脉络
Chat Paper
正在生成论文摘要