Machine learning in the context of better healthcare in aging
Aging(2023)
摘要
The World Health Organization estimated that, in 2019, 1 billion people were aged 60 or older, being predicted to rise to 1.4 and 2.1 billions by 2030 and 2050, respectively. With aging and its related progressive accumulation of cellular and molecular damage, the organism suffers progressive physiological changes that lead to senescence, decline of biological functions, and reduced capacity to adapt to metabolic stress, increasing the risk for disease, and ultimately death. Moreover, aging increases the individual’s susceptibility to undergo simultaneous comorbidities, representing one of the major socioeconomic achievements and privileges of the last century, but also as a major social, economic, and medical challenge. In particular, an older world population creates a new distribution of health expenditure, with a significant change toward chronic diseases and other aging-related diseases (e.g., cardiovascular and neurological diseases). These changes cause unaffordable costs for health systems. At the same time, there is an unprecedented amount of age-related biological data being collected and made publicly available. This scenario, coupled with the renewed interest and advances observed in the area of machine learning (ML), creates a decisive opportunity for the development and application of effective computational methods, aiming at unveiling the real potential of the collected data and, therefore, to extract meaningful knowledge from it. This chapter explores potential biomarkers of biological aging and describes two examples where biological aging-related diseases can benefit from the application of ML-related techniques: the first example describes a home telemonitoring environment for the early detection of heart failure, whereas the second situation relies on the analysis of real data to early predict the potential development of multiple age-related diseases.
更多查看译文
关键词
machine learning,better healthcare,aging
AI 理解论文
溯源树
样例
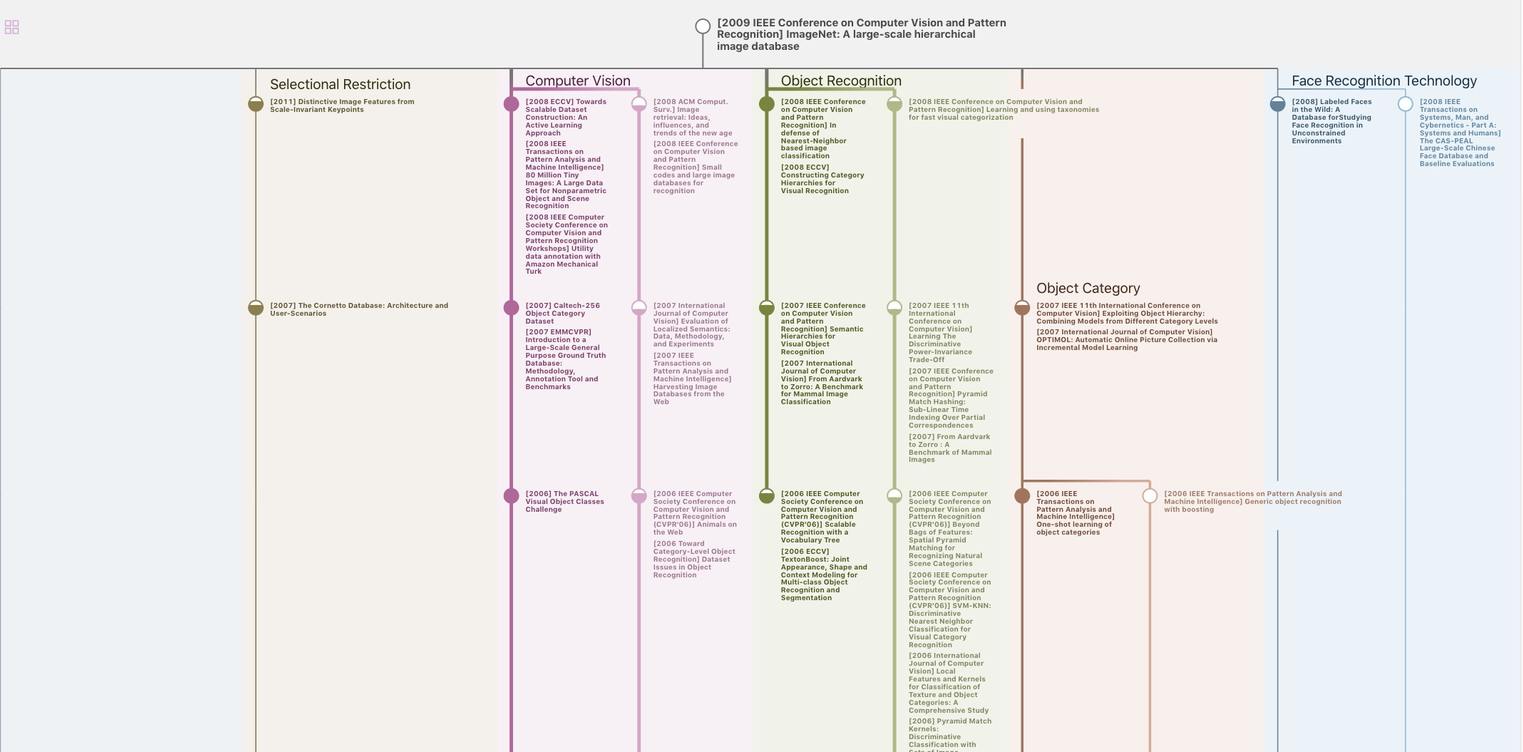
生成溯源树,研究论文发展脉络
Chat Paper
正在生成论文摘要