Data-driven optimization of sampling patterns for MR brain T-1 rho mapping
MAGNETIC RESONANCE IN MEDICINE(2023)
摘要
Purpose: The goal of this study was to apply a fast data-driven optimization algorithm, called bias-accelerated subset selection, for MR brain T-1 rho mapping to generate optimized sampling patterns (SPs) for compressed sensing reconstruction of brain 3D-T-1 rho MRI. Methods: Five healthy volunteers were recruited, and fully sampled Cartesian 3D-T-1 rho MRIs were obtained. Variable density (VD) and Poisson disc (PD) undersampling was used as the input to SP optimization process. The reconstruction used 3 compressed sensing methods: spatiotemporal finite differences, low-rank plus sparse with spatial finite differences, and low rank. The performance of images and T-1 rho maps using PD-SP and VD-SP and their optimized sampling patterns (PD-OSP and VD-OSP) were compared to the fully sampled reference using normalized root mean square error (NRMSE). Results: The VD-OSP with spatiotemporal finite differences reconstruction (NRMSE = 0.078) and the PD-OSP with spatiotemporal finite differences reconstruction (NRMSE = 0.079) at the highest acceleration factors (AF = 30) showed the largest improvement compared to the respective nonoptimized SPs (VD NRMSE = 0.087 and PD NRMSE = 0.149). Prospective undersampling was tested at AF = 4, with VD-OSP NRMSE = 0.057 versus PD-OSP NRMSE = 0.060, with optimized sampling performing better that input PD or VD sampling. For brain T-1 rho mapping, the VD-OSP with low rank reconstruction for AFs <10 and VD-OSP with spatiotemporal finite differences for AFs >10 perform better. Conclusions: The study demonstrated that the appropriate use of data-driven optimized sampling and suitable compressed sensing reconstruction technique can be employed to potentially accelerate 3D T-1 rho mapping for brain imaging applications.
更多查看译文
关键词
algorithms, brain MRI, data-driven sampling pattern, T-1 rho mapping
AI 理解论文
溯源树
样例
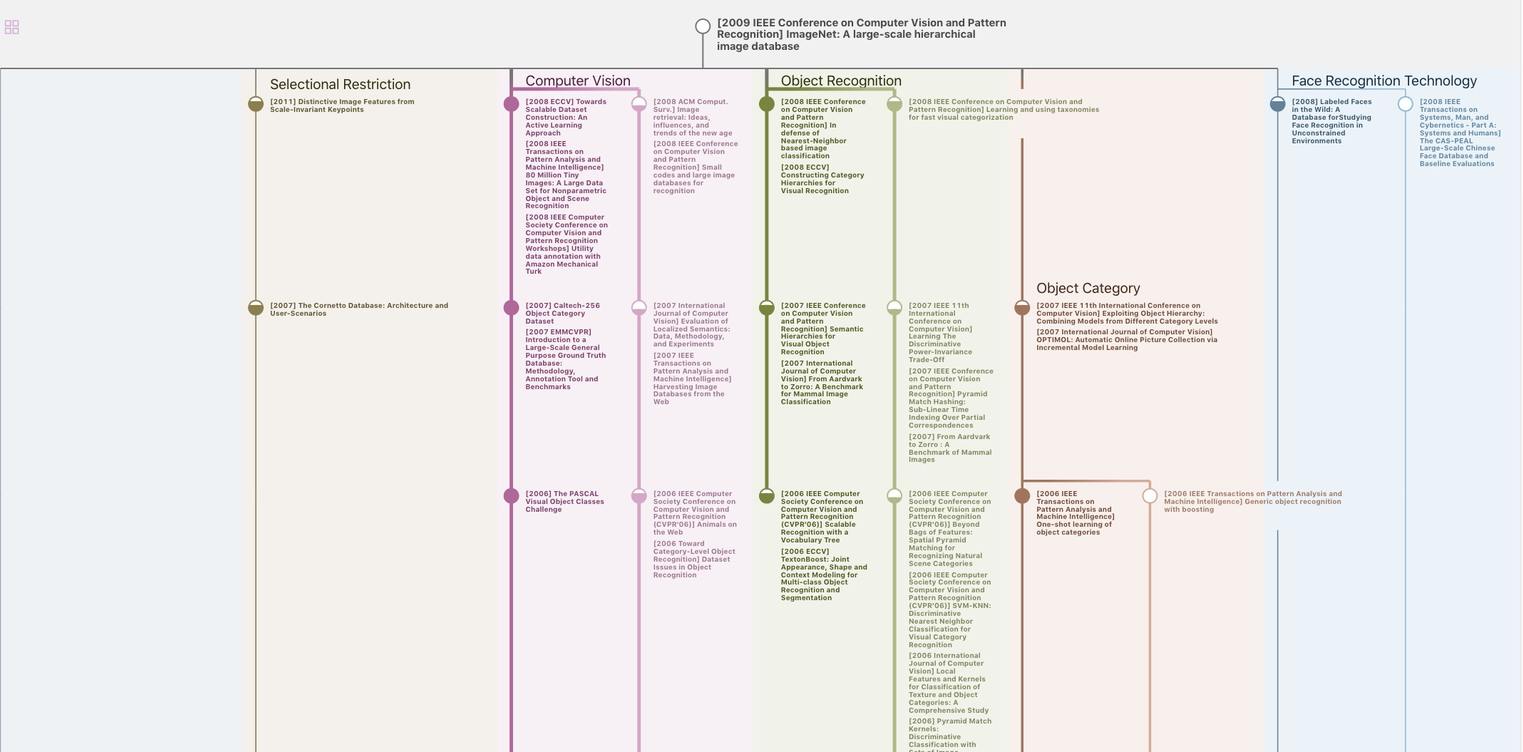
生成溯源树,研究论文发展脉络
Chat Paper
正在生成论文摘要