Fault Diagnosis of Wheeled Robot Based on Prior Knowledge and Spatial-Temporal Difference Graph Convolutional Network
IEEE Transactions on Industrial Informatics(2023)
摘要
The critical issue of wheeled robot fault diagnosis is to comprehensively evaluate its health condition using multisensor data, but traditional deep learning-based methods are hard to model the relationships among sensor measurements. Unlike these methods, the graph convolutional network (GCN), which uses the graph-structured data along with the association graph as input, is more efficient for relationship modeling. However, existing GCN-based fault diagnosis methods suffer from the following weaknesses: the association graphs are obtained according to the similarity of data samples or their features, which cannot guarantee accuracy; and these models are focused on spatial correlations and neglect temporal correlations. To address these problems, we propose to construct the association graph based on prior knowledge, i.e., a simplified mathematical model of the wheeled robot. Moreover, we develop a spatial-temporal difference graph convolutional network (STDGCN) for wheeled robot fault diagnosis. This network contains a difference layer that utilizes localized difference properties for feature enhancement, and the spatial-temporal graph convolutional modules are introduced to jointly capture the spatial-temporal correlations. To verify the effectiveness of the STDGCN for fault diagnosis, experiments are carried out, and the results show that the STDGCN achieves superior performance.
更多查看译文
关键词
Robot sensing systems,Mobile robots,Robots,Convolution,Fault diagnosis,Feature extraction,Robot kinematics,Deep learning,graph convolutional network,intelligent fault diagnosis,wheeled robot
AI 理解论文
溯源树
样例
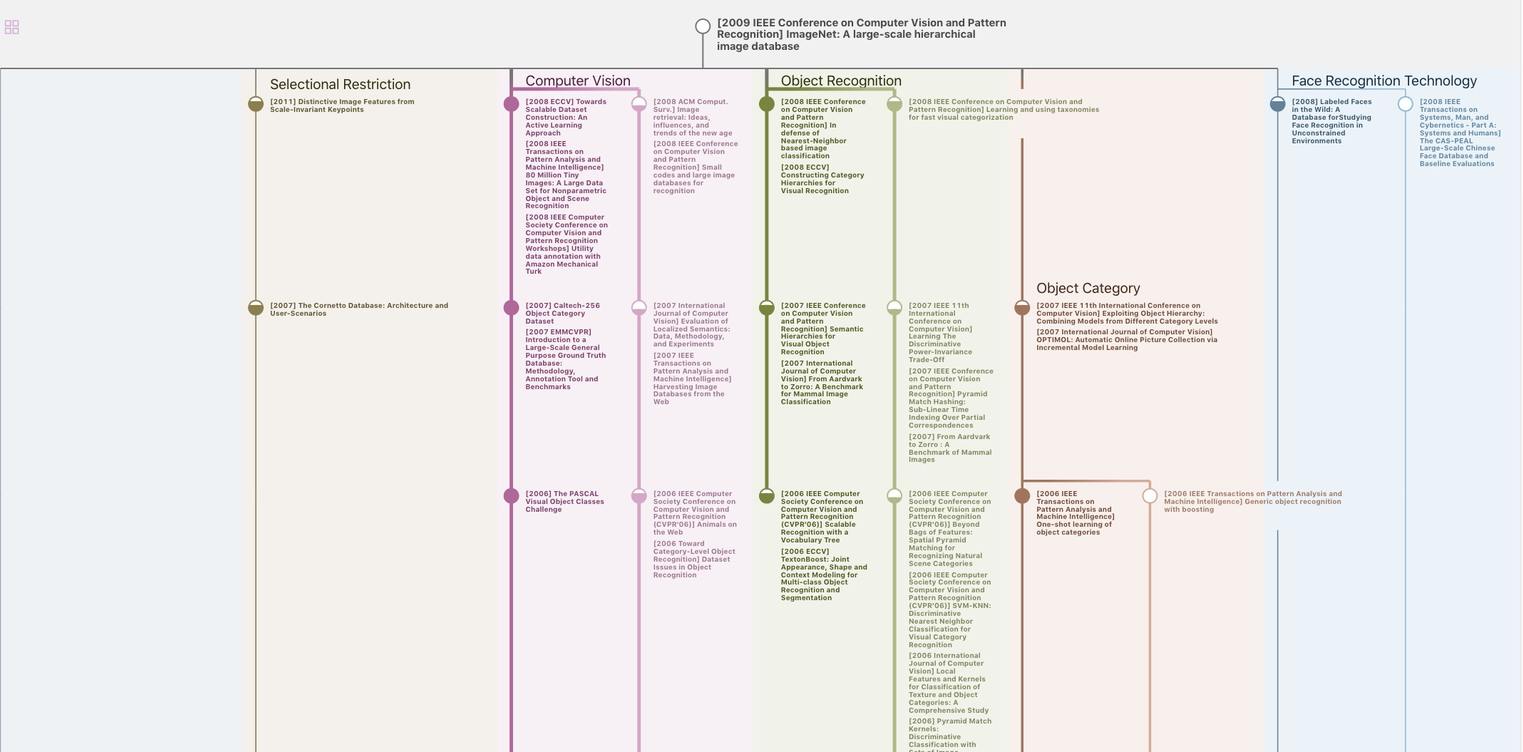
生成溯源树,研究论文发展脉络
Chat Paper
正在生成论文摘要