Open-vocabulary Queryable Scene Representations for Real World Planning
arxiv(2022)
摘要
Large language models (LLMs) have unlocked new capabilities of task planning from human instructions. However, prior attempts to apply LLMs to real-world robotic tasks are limited by the lack of grounding in the surrounding scene. In this paper, we develop NLMap, an open-vocabulary and queryable scene representation to address this problem. NLMap serves as a framework to gather and integrate contextual information into LLM planners, allowing them to see and query available objects in the scene before generating a context-conditioned plan. NLMap first establishes a natural language queryable scene representation with Visual Language models (VLMs). An LLM based object proposal module parses instructions and proposes involved objects to query the scene representation for object availability and location. An LLM planner then plans with such information about the scene. NLMap allows robots to operate without a fixed list of objects nor executable options, enabling real robot operation unachievable by previous methods. Project website: https://nlmap-saycan.github.io
更多查看译文
关键词
real world planning,representations,open-vocabulary
AI 理解论文
溯源树
样例
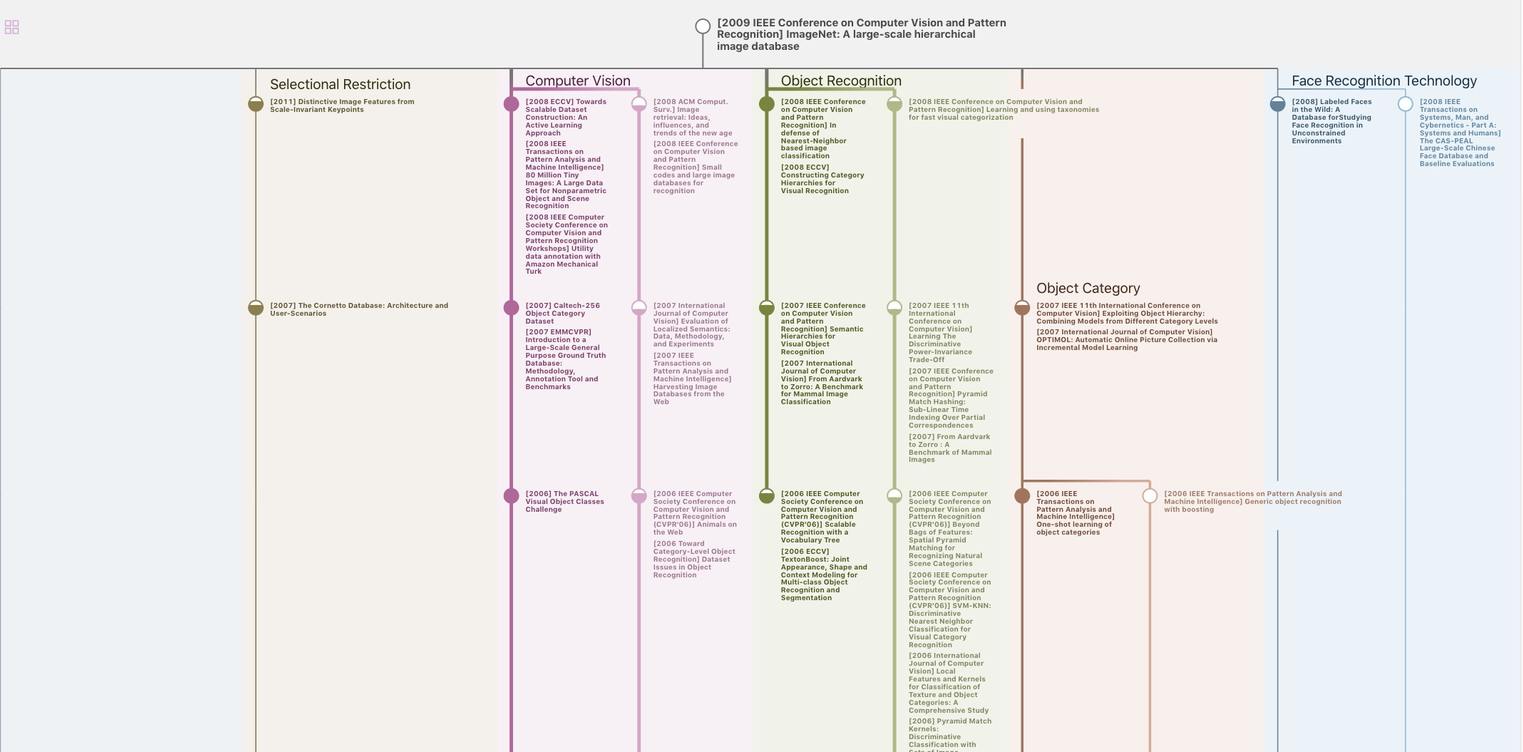
生成溯源树,研究论文发展脉络
Chat Paper
正在生成论文摘要