Analogy-Based Post-treatment of CNN Image Segmentations
Case-Based Reasoning Research and Development(2022)
摘要
Convolutional neural networks (CNNs) have proven to be efficient tools for image segmentation when a large number of segmented images are available. However, when the number of segmented images is not so large, the CNN segmentations are less accurate. It is the case for nephroblastoma (kidney cancer) in particular. When a new patient arrives, the expert can only manually segment a sample of scanned images since manual segmentation is a time-consuming process. As a consequence, the question of how to compute accurate segmentations using both the trained CNN and such a sample is raised. A CBR approach based on proportional analogy is proposed in this paper. For a source image segmented by the expert, let a be the CNN segmentation of this image, b be its expert segmentation and c be the CNN segmentation of a target image close to the source image. The proposed approach aims at solving the analogical equation “a is to b as c is to d” with unknown d: the solution d of this equation is proposed as a segmentation of the target image. This approach and some of its improvements are evaluated and show an accuracy increase of the segmentation with respect to the CNN segmentation.
更多查看译文
关键词
Analogical extrapolation, Case-based reasoning, Convolutional neural networks, Medical image segmentation, Kidney cancer
AI 理解论文
溯源树
样例
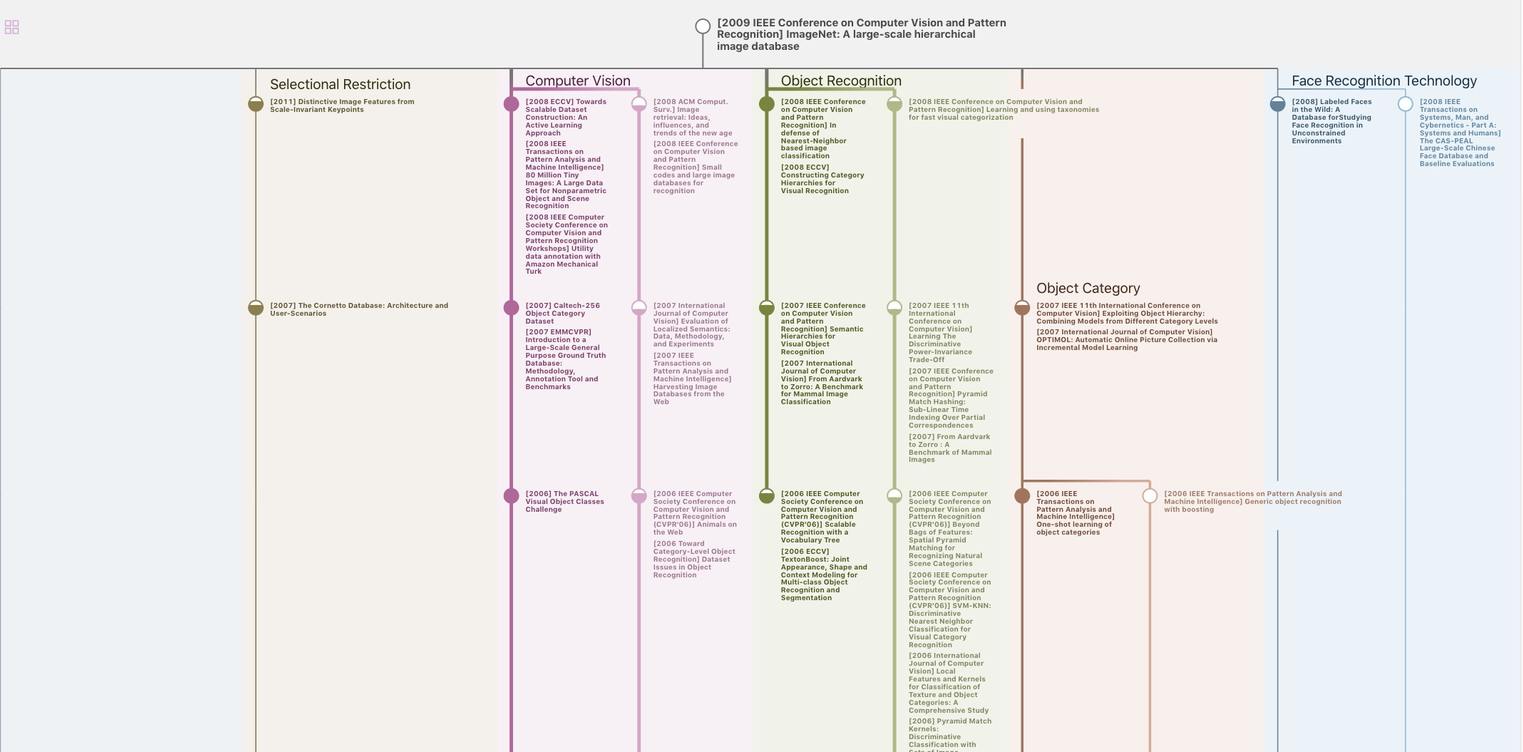
生成溯源树,研究论文发展脉络
Chat Paper
正在生成论文摘要