DeepRetroMoCo: Deep neural network-based Retrospective Motion Correction Algorithm for Spinal Cord functional MRI
biorxiv(2022)
摘要
There are unique challenges in the preprocessing of spinal cord fMRI data, particularly voluntary or involuntary movement artifacts during image acquisition. Despite advances in data processing techniques for movement detection and correction, there are challenges in extrapolating motion correction algorithm developments in the brain cortex to the brainstem and spinal cord. We trained a Deep Learning-based convolutional neural network (CNN) via an unsupervised learning algorithm, called DeepRetroMoCo, to detect and correct motions in axial T2*-weighted spinal cord data. Spinal cord fMRI data from 27 participants were used for training of the network (135 runs for training and 81 runs for testing). We used average temporal signal-to-noise-ratio (tSNR) and Delta Variation Signal (DVARS) of raw and motion-corrected images to compare the outcome of DeepRetroMoco with sct\_fmri\_moco implemented in the spinal cord toolbox. The average tSNR in the cervical cord was significantly higher when DeepRetroMoco was used for motion correction compared to sct\_fmri\_moco method. Average DVARS was lower in images corrected by DeepRetroMoco than those corrected by sct\_fmri\_moco. The average processing time for DeepRetroMoco was also significantly shorter than sct\_fmri\_moco. Our results suggest that DeepRetroMoCo improves motion correction procedures in fMRI data acquired from the cervical spinal cord.
### Competing Interest Statement
The authors have declared no competing interest.
更多查看译文
关键词
retrospective motion correction algorithm,mri,spinal cord,network-based
AI 理解论文
溯源树
样例
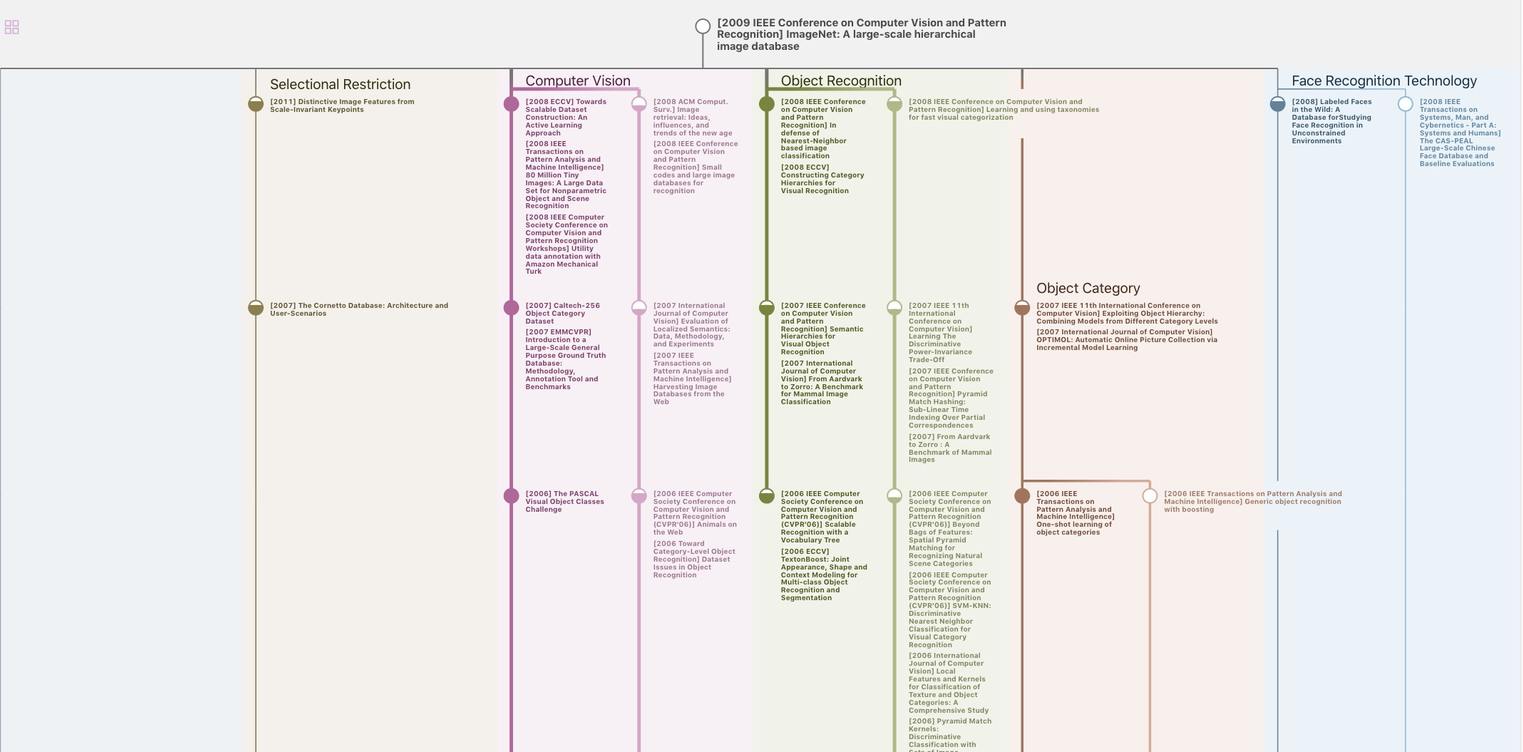
生成溯源树,研究论文发展脉络
Chat Paper
正在生成论文摘要