A Deep Learning and Digital Archaeology Approach for Mosquito Repellent Discovery
openalex(2022)
Key words
Insect Farming
AI Read Science
Must-Reading Tree
Example
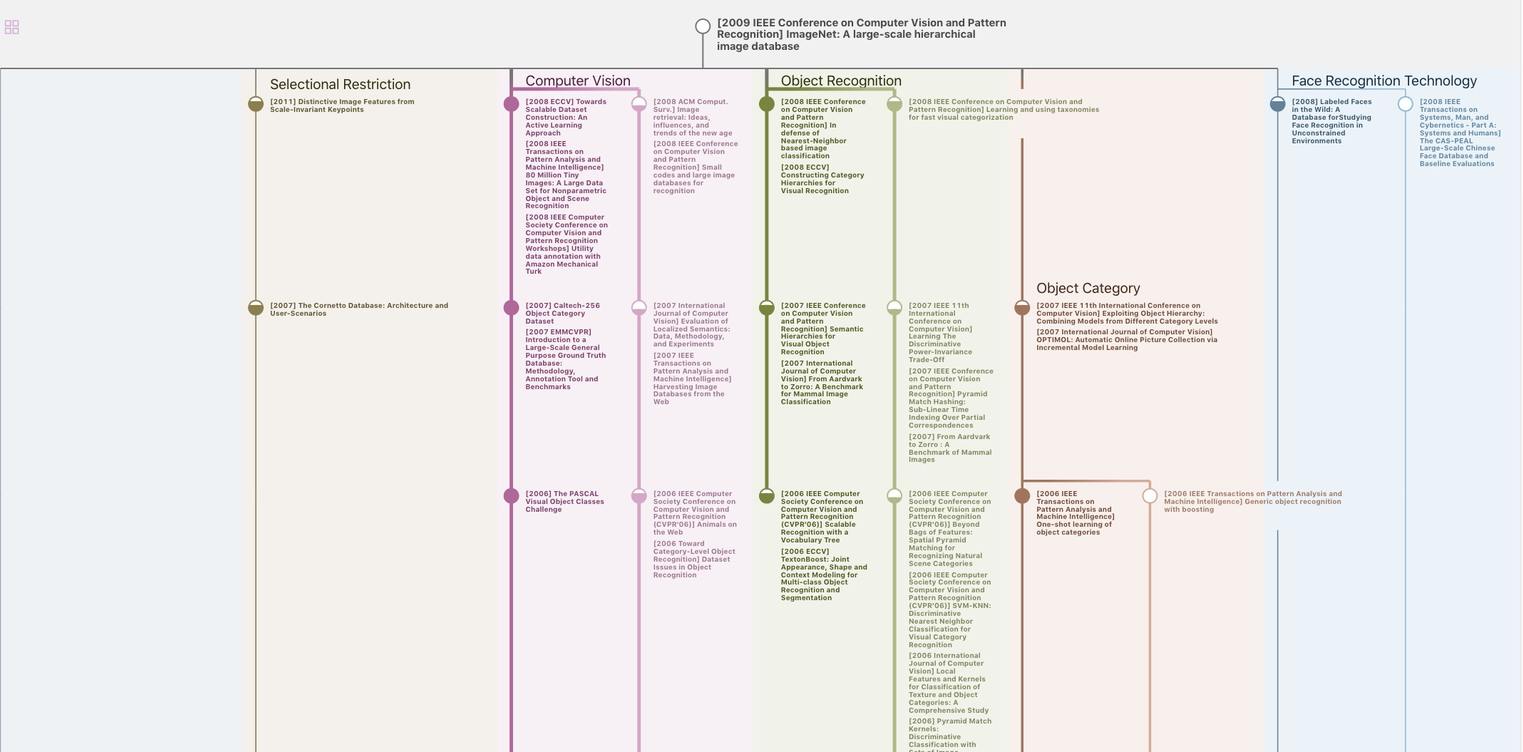
Generate MRT to find the research sequence of this paper
Chat Paper
Summary is being generated by the instructions you defined