Unified Tumor Growth Mechanisms from Multimodel Inference and Dataset Integration
biorxiv(2022)
摘要
Systems approaches to elucidate biological processes that impact human health leverage mathematical models encoding mechanistic hypotheses suitable for experimental validation. However, building a single model fit to one dataset may miss alternate equally valid mathematical formulations, and available data may not be sufficient to fully elucidate mechanisms underlying system behavior. Here, we overcome these limitations via a Bayesian multimodel inference (Bayesian-MMI) approach, which estimates how multiple mechanistic hypotheses explain experimental datasets, concurrently quantifying how each dataset informs each hypothesis. We apply this approach to unanswered questions about heterogeneity, lineage plasticity, and cell-cell interaction dynamics in small cell lung cancer (SCLC) tumor growth. Through available dataset integration, we find that Bayesian-MMI predictions support tumor evolution promoted by high lineage plasticity, rather than through expanding rare stem-like populations. These results highlight that given available data, any SCLC cellular subtype can contribute to tumor repopulation post-treatment, suggesting a mechanistic interpretation for tumor recalcitrance.
### Competing Interest Statement
The authors have declared no competing interest.
更多查看译文
AI 理解论文
溯源树
样例
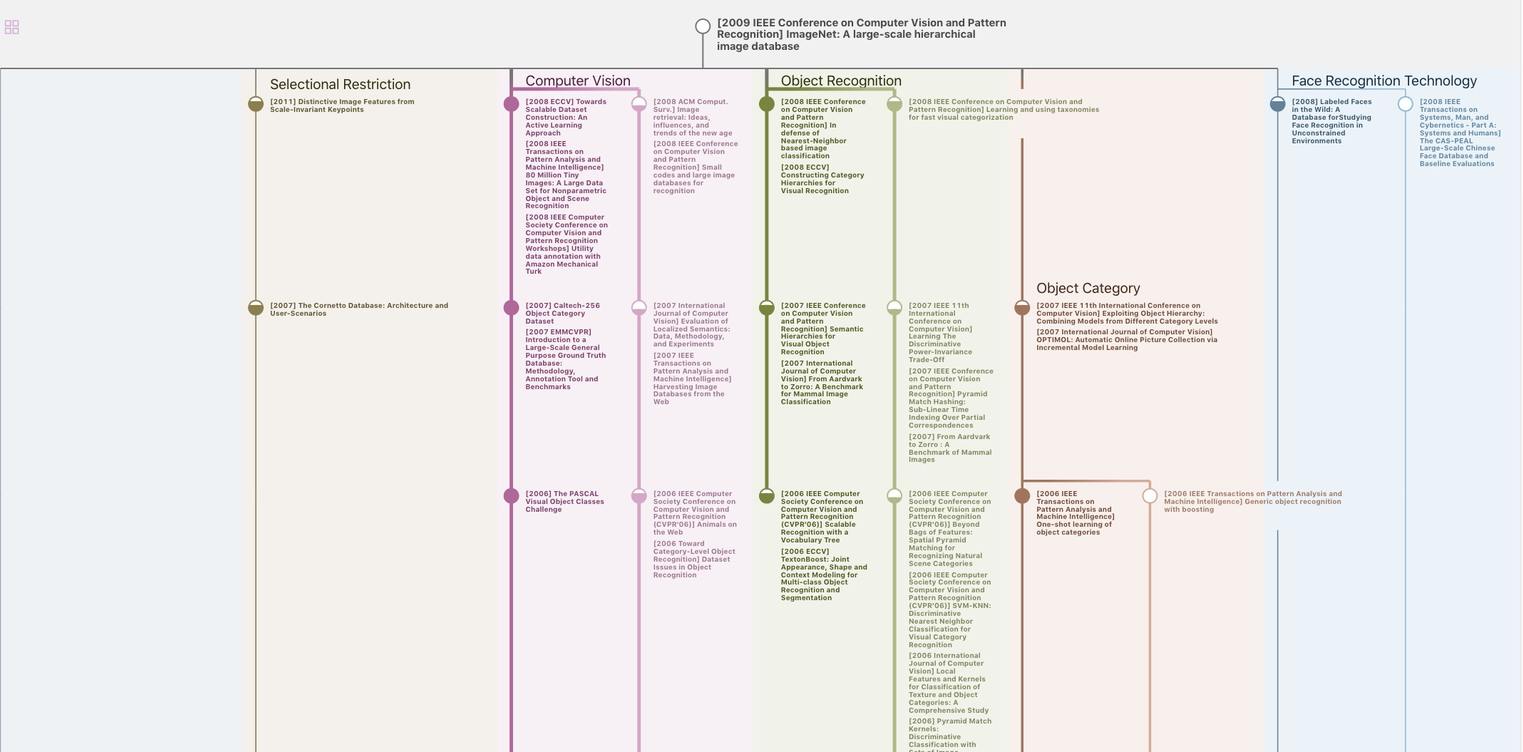
生成溯源树,研究论文发展脉络
Chat Paper
正在生成论文摘要