Bayesian nonnegative matrix factorization in an incremental manner for data representation
Applied Intelligence(2022)
摘要
Nonnegative matrix factorization (NMF) is a novel paradigm for feature representation and dimensionality reduction. However, the performance of the NMF model is affected by two critical and challenging problems. One is that the original NMF does not consider the distribution information of data and parameters, resulting in inaccurate representations. The other is the high computational complexity in online processing. Bayesian approaches are proposed to address the former problem of NMF. However, most existing Bayesian-based NMF models utilize an exponential prior, which only guarantees the nonnegativity of parameters without fully considering the prior information of the parameters. Thus, a new Bayesian-based NMF model is constructed based on the Gaussian likelihood and a truncated Gaussian prior, called the truncated Gaussian-based NMF (TG-NMF) model, in which a truncated Gaussian prior can prevent overfitting while ensuring nonnegativity. Furthermore, Bayesian inference-based incremental learning is introduced to reduce the high computational complexity of TG-NMF; this model is called TG-INMF. We adopt variational Bayesian to estimate all parameters of TG-NMF and TG-INMF. Experiments on genetic data-based tumor recognition demonstrate that our models are competitive with other existing methods for classification problems.
更多查看译文
关键词
Nonnegative matrix factorization, Incremental learning, Truncated Gaussian prior, Inverse space sparse representation based classification, Tumor recognition
AI 理解论文
溯源树
样例
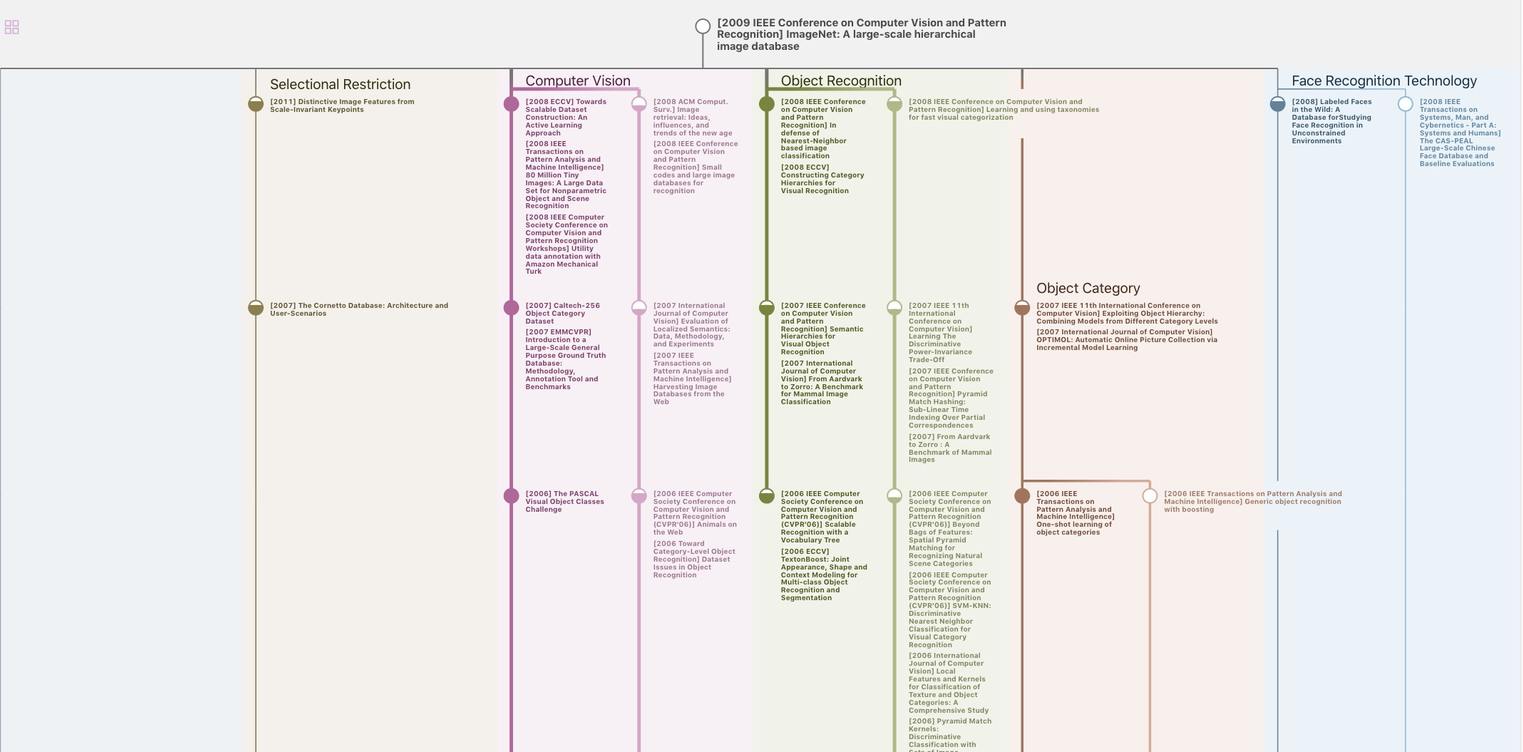
生成溯源树,研究论文发展脉络
Chat Paper
正在生成论文摘要