ARISE: A Multitask Weak Supervision Framework for Network Measurements
IEEE Journal on Selected Areas in Communications(2022)
摘要
The application of machine learning (ML) to mitigate network-related problems poses significant challenges for researchers and operators alike. For one, there is a general lack of labeled training data in networking, and labeling techniques popular in other domains are ill-suited due to the scarcity of operators’ domain expertise. Second, network problems are typically multi-tasked in nature, requiring multiple ML models (one per task) and resulting in multiplicative increases in training times as the number of tasks increases. Third, the adoption of ML by network operators hinges on the models’ ability to provide basic reasoning about their decision-making procedures. To address these challenges, we propose ARISE, a multi-task weak supervision framework for network measurements. ARISE uses weak supervision-based data programming to label network data at scale and applies learning paradigms such as multi-task learning (MTL) and meta-learning to facilitate information sharing between tasks as well as reduce overall training time. Using community datasets, we show that ARISE can generate MTL models with improved classification accuracy compared to multiple single-task learning (STL) models. We also report findings that show the promise of MTL models for providing a means for reasoning about their decision-making process, at least at the level of individual tasks.
更多查看译文
关键词
Multitask weak supervision,network measurements,internet data science
AI 理解论文
溯源树
样例
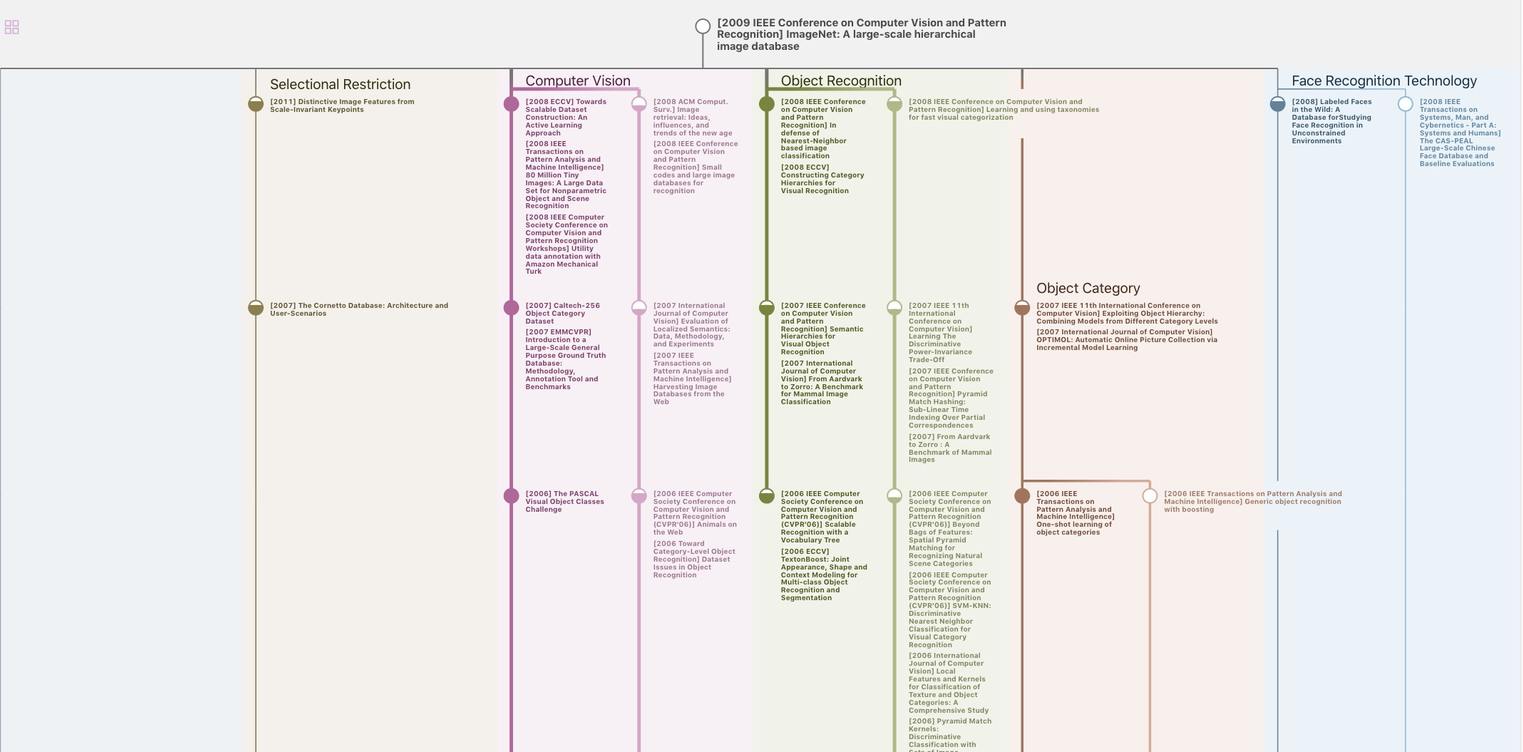
生成溯源树,研究论文发展脉络
Chat Paper
正在生成论文摘要