Dual-Anchor Metric Learning for Blind Image Quality Assessment of Screen Content Images
ELECTRONICS(2022)
摘要
The natural scene statistic is destroyed by the artificial portion in the screen content images (SCIs) and is also impractical for obtaining an accurate statistical model due to the variable composition of the artificial and natural parts in SCIs. To resolve this problem, this paper presents a dual-anchor metric learning (DAML) method that is inspired by metric learning to obtain discriminative statistical features and further identify complex distortions, as well as predict SCI image quality. First, two Gaussian mixed models with prior data are constructed as the target anchors of the statistical model from natural and artificial image databases, which can effectively enhance the metrical discrimination of the mapping relation between the feature representation and quality degradation by conditional probability analysis. Then, the distances of the high-order statistics are softly aggregated to conduct metric learning between the local features and clusters of each target statistical model. Through empirical analysis and experimental verification, only variance differences are used as quality-aware features to benefit the balance of complexity and effectiveness. Finally, the mapping model between the target distances and subjective quality can be obtained by support vector regression. To validate the performance of DAML, multiple experiments are carried out on three public databases: SIQAD, SCD, and SCID. Meanwhile, PLCC, SRCC, and the RMSE are then employed to compute the correlation between subjective and objective ratings, which can estimate the prediction of accuracy, monotonicity, and consistency, respectively. The PLCC and RMSE of the method achieved 0.9136 and 0.7993. The results confirm the good performance of the proposed method.
更多查看译文
关键词
blind image quality assessment, screen content image, metric learning, Gaussian mixture model
AI 理解论文
溯源树
样例
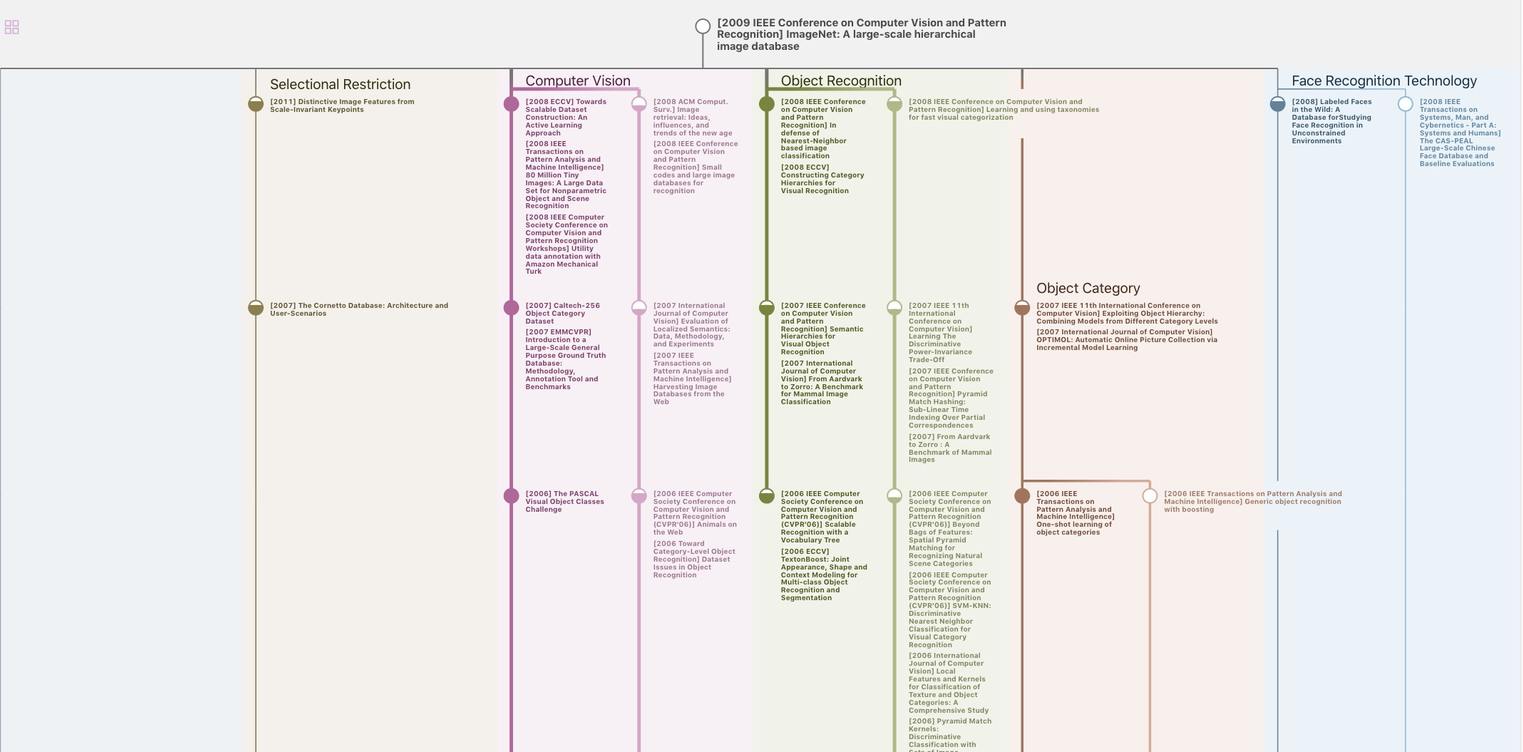
生成溯源树,研究论文发展脉络
Chat Paper
正在生成论文摘要