Language Bias-Driven Self-Knowledge Distillation with Generalization Uncertainty for Reducing Language Bias in Visual Question Answering
APPLIED SCIENCES-BASEL(2022)
摘要
To answer questions, visual question answering systems (VQA) rely on language bias but ignore the information of the images, which has negative information on its generalization. The mainstream debiased methods focus on removing language prior to inferring. However, the image samples are distributed unevenly in the dataset, so the feature sets acquired by the model often cannot cover the features (views) of the tail samples. Therefore, language bias occurs. This paper proposes a language bias-driven self-knowledge distillation framework to implicitly learn the feature sets of multi-views so as to reduce language bias. Moreover, to measure the performance of student models, the authors of this paper use a generalization uncertainty index to help student models learn unbiased visual knowledge and force them to focus more on the questions that cannot be answered based on language bias alone. In addition, the authors of this paper analyze the theory of the proposed method and verify the positive correlation between generalization uncertainty and expected test error. The authors of this paper validate the method's effectiveness on the VQA-CP v2, VQA-CP v1 and VQA v2 datasets through extensive ablation experiments.
更多查看译文
关键词
visual question answering, self-knowledge distillation, language bias, generalized uncertainty
AI 理解论文
溯源树
样例
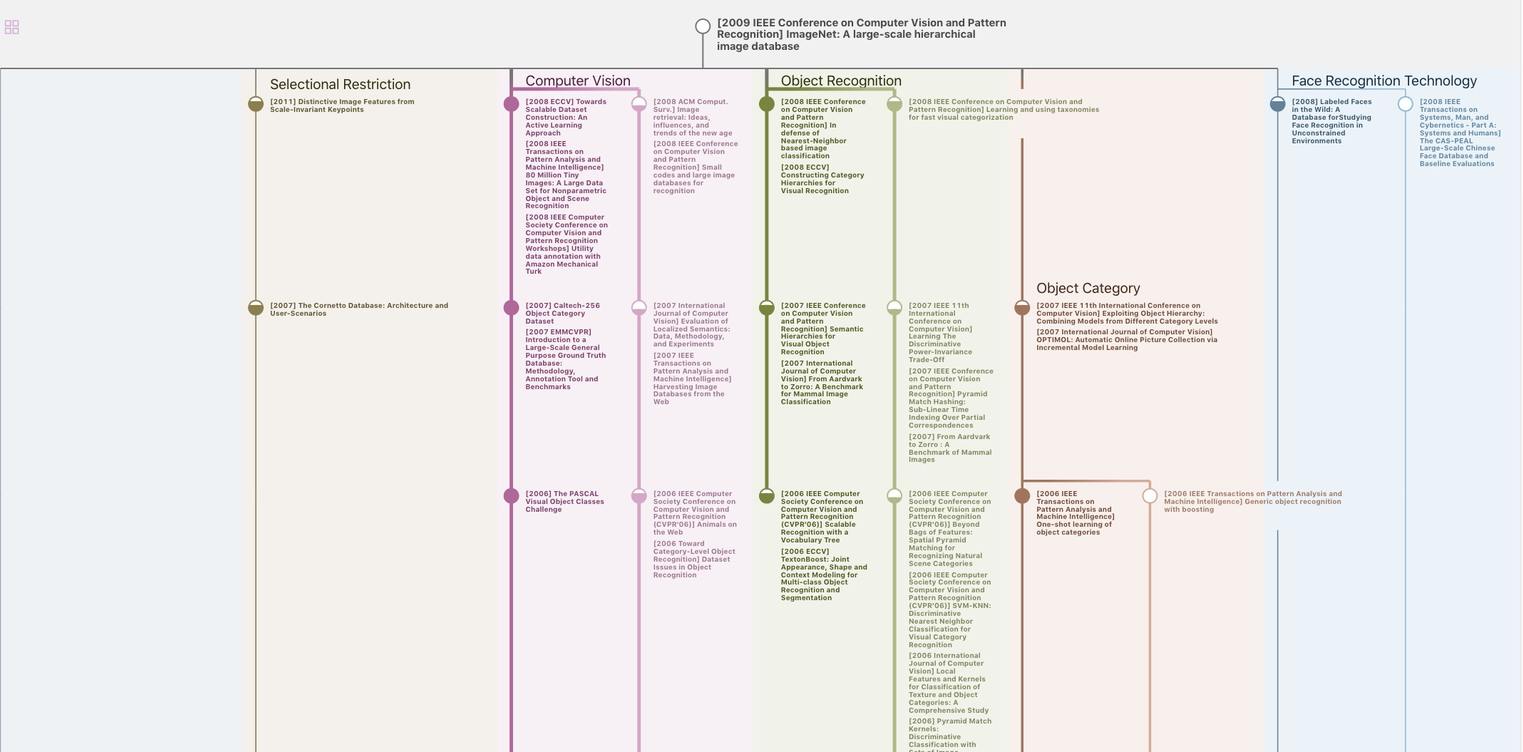
生成溯源树,研究论文发展脉络
Chat Paper
正在生成论文摘要