Traffic flow prediction in inland waterways of Assam region using uncertain spatiotemporal correlative features
ACTA GEOPHYSICA(2022)
摘要
Modern civilization has reported a significant rise in the volume of traffic on inland rivers all over the globe. Traffic flow prediction is essential for a good travel experience, but adequate computer processes for processing unpredictable spatiotemporal data (timestamp, weather, vessel_ID, water level, vessel_position, vessel_speed) in the inland water transportation industry are lacking. Moreover, such type of prediction relies primarily on past traffic patterns and perhaps other pertinent facts. Thus, we propose a deep learning-based computing process, namely Convolution Neural Network-Long Short-Term Memory Network (CNN-LSTM), a progressive predictor of employing uncertain spatiotemporal information to decrease navigation mishaps, traffic and flow prediction failures during transportation. Spatiotemporal correlation of current traffic flow may be processed using a simplified CNN-LSTM model. This hybridized prediction technique decreases update costs and meets the prediction needs with minimal computing overhead. A short case study on the waterways of the Indian state of Assam from Sandiya (27.835090 latitude, 95.658590 longitude) to Dhubri (26.022699 latitude, 89.978401 longitude) is undertaken to assess the model's performance. The evaluation of the suggested method includes a variety of trajectories of water transportation vehicles, including ferries, sailing boats, container ships, etc. The suggested approach outperforms conventional traffic flow predicting methods when it comes to short-term prediction with minimal predictive error (< 2.75) and exhibited a major difference of more than 45% on the comparison of other methods.
更多查看译文
关键词
Deep learning, CNN-LSTM, Traffic flow, Prediction, Waterways, Relative error, RoI, RNN, Optimizer, Drop rate
AI 理解论文
溯源树
样例
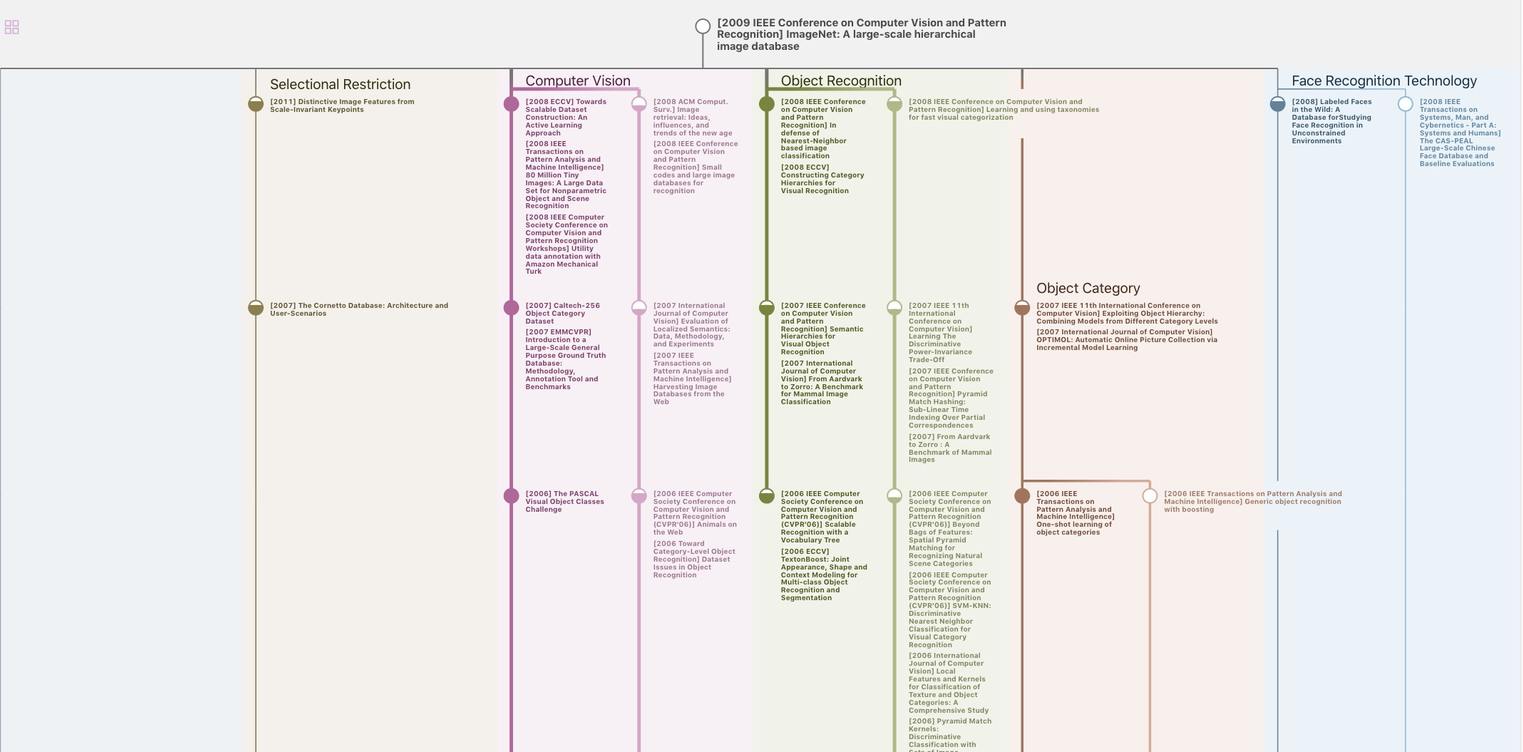
生成溯源树,研究论文发展脉络
Chat Paper
正在生成论文摘要