An Empirical Study of Reward Explanations With Human-Robot Interaction Applications
IEEE Robotics and Automation Letters(2022)
摘要
Explainable AI techniques that describe agent reward functions can enhance human-robot collaboration in a variety of settings. However, in order to effectively explain reward information to humans, it is important to understand the efficacy of different types of explanation techniques in scenarios of varying complexity. In this letter, we compare the performance of a broad range of explanation techniques in scenarios of differing reward function complexity through a set of human-subject experiments. To perform this analysis, we first introduce a categorization of reward explanation information types and then apply a suite of assessments to measure human reward understanding. Our findings indicate that increased reward complexity (in number of features) corresponded to higher workload and decreased reward understanding, while providing direct reward information was an effective approach across reward complexities. We also observed that providing full or near full reward information was associated with increased workload and that providing abstractions of the reward was more effective at supporting reward understanding than other approaches (besides direct information) and was associated with decreased workload and improved subjective assessment in high complexity settings.
更多查看译文
关键词
Human-robot collaboration,human factors and human-in-the-loop,human-centered automation
AI 理解论文
溯源树
样例
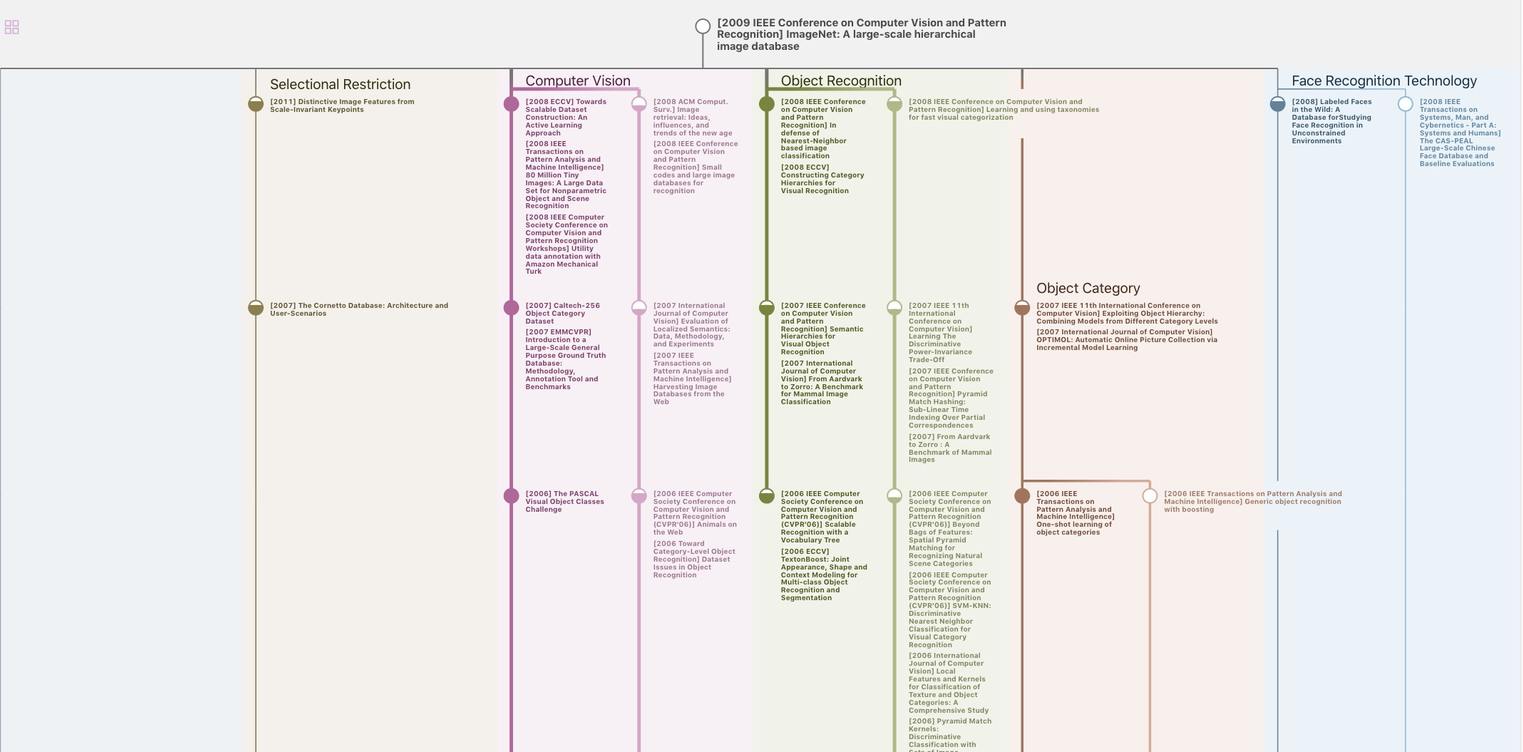
生成溯源树,研究论文发展脉络
Chat Paper
正在生成论文摘要