Towards an efficient backbone for preserving features in speech emotion recognition: deep-shallow convolution with recurrent neural network
NEURAL COMPUTING & APPLICATIONS(2022)
摘要
Speech emotion recognition (SER) has attracted a great deal of research interest, which plays as a critical role in human-machine interactions. Unlike other visual tasks, SER becomes intractable when the convolutional neural networks (CNNs) are employed, owing to their limitation in handling log-mel spectrograms. Therefore, it is useful to establish a feature-extraction backbone that allows CNNs to maintain information integrity of speech utterances when utilizing log-mel spectrograms. Moreover, a neural network with a deep stack of layers can lead to a performance degradation due to various challenges, including information loss, overfitting, or vanishing gradient issues. Many studies employ hybrid/multi-modal methods or specialized network designs to mitigate these obstacles. However, those methods often are unstable, hard to configure and non-adaptive to different tasks. In this research, we propose a reusable backbone pertaining to CNN blocks for undertaking SER tasks, as inspired by the FishNet model. denoted as deep-swallow convolution with RNN (DSCRNN), this proposed backbone method preserves features from both deep and shallow layers, which is effective in improving quality of features extracted from log-mel spectrograms. Simulation results indicate that our proposed DSCRNN backbone achieves improved accuracy rates of 2% and 11% when comparing with those from a baseline model with traditional CNN blocks in a speaker-independent evaluation utilizing the RAVDESS dataset with 4 classes and 8 classes, respectively.
更多查看译文
关键词
Speech emotion recognition,SER,FishNet,Log-mel spectrogram,Deep learning,IEMOCAP,RAVDESS,Human machine interaction,CNN,RNN
AI 理解论文
溯源树
样例
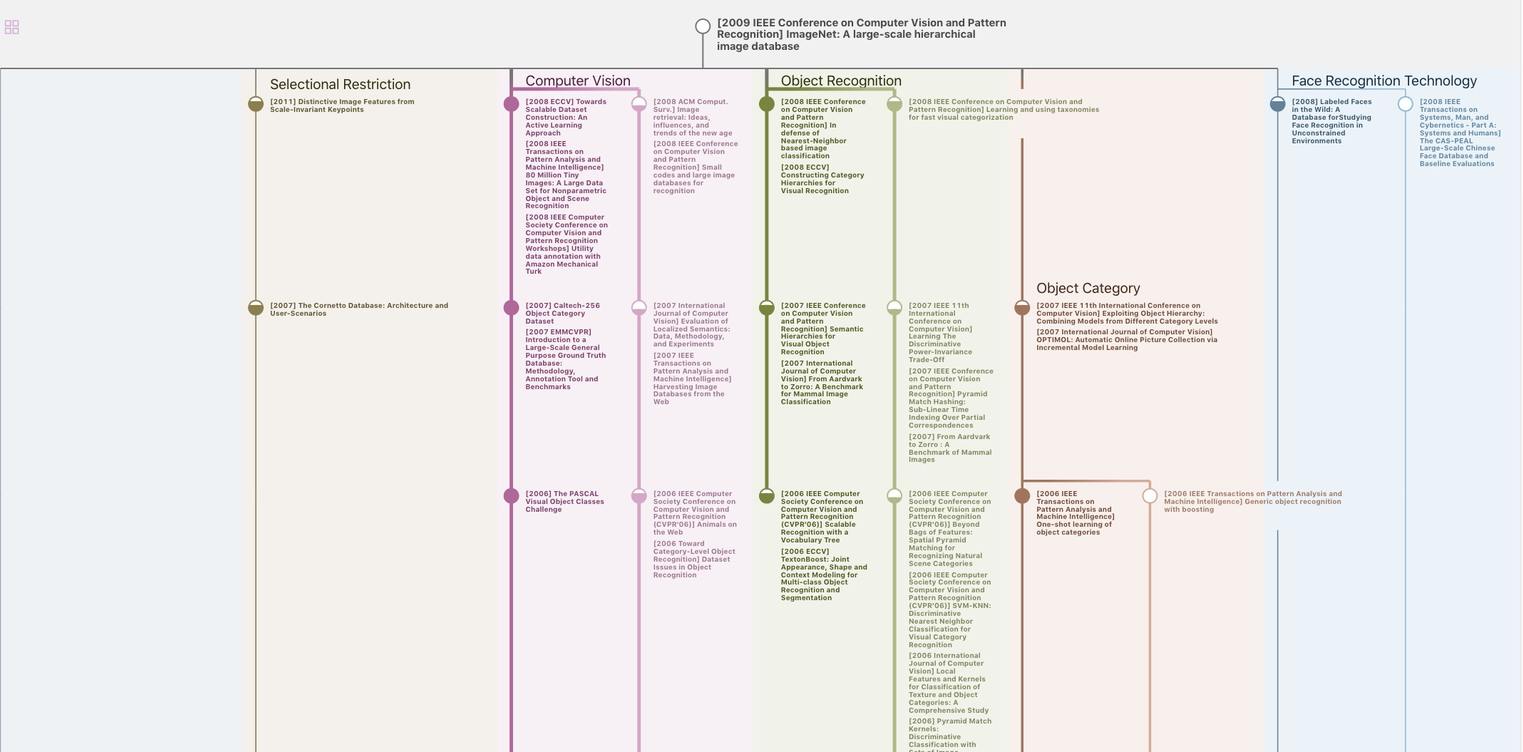
生成溯源树,研究论文发展脉络
Chat Paper
正在生成论文摘要