Incorporate radiograph-reading behavior and knowledge into deep reinforcement learning for lesion localization
MEDICAL IMAGING 2022: COMPUTER-AIDED DIAGNOSIS(2022)
摘要
We design an intelligent tool that imitates radiologists' reading behavior and knowledge for lesion localization on radiographs using deep reinforcement learning (DRL). We formulate a human visual search behavior, i.e., 'first searching for, then focusing on' a region of interest (ROI), as a Markov Decision Process (MDP). The state of MDP is represented by a candidate ROI's imaging features and historical actions of lesion searching. The imaging features are extracted by a convolutional neural network that is pre-trained for disease classification. The state transition in MDP is achieved by Q-learning. Specifically, we design a Q network to simulate a radiologist's succession of saccades and fixations - iteratively choosing the next ROI of radiograph to pay attention to while reading images. Guided by a novel rewarding scheme, our algorithm learns to iteratively zoom in for a close-up assessment of the potential abnormal sub-regions, until the termination condition is met. We train and test our model with 80% and 20% of the ChestX-ray8 dataset with pathologically confirmed bounding boxes (B-Boxes), respectively. The localization accuracy is measured at different thresholds of intersection over union (IoU) between the DRL-generated and the ground truth B-Box. The proposed method achieves accuracy of 0.996, 0.412, 0.667, 0.650 at threshold 0.1 respectively for cardiomegaly, mass, pneumonia, and pneumothorax. While our study is a preliminary work, it demonstrates a potential approach and the feasibility of incorporating human expert experience into a lesion detection-based machine learning task. Further investigation and evaluation of our method will be needed in future work.
更多查看译文
关键词
Thoracic disease,X-ray,Deep reinforcement learning,Q-learning,Disease localization
AI 理解论文
溯源树
样例
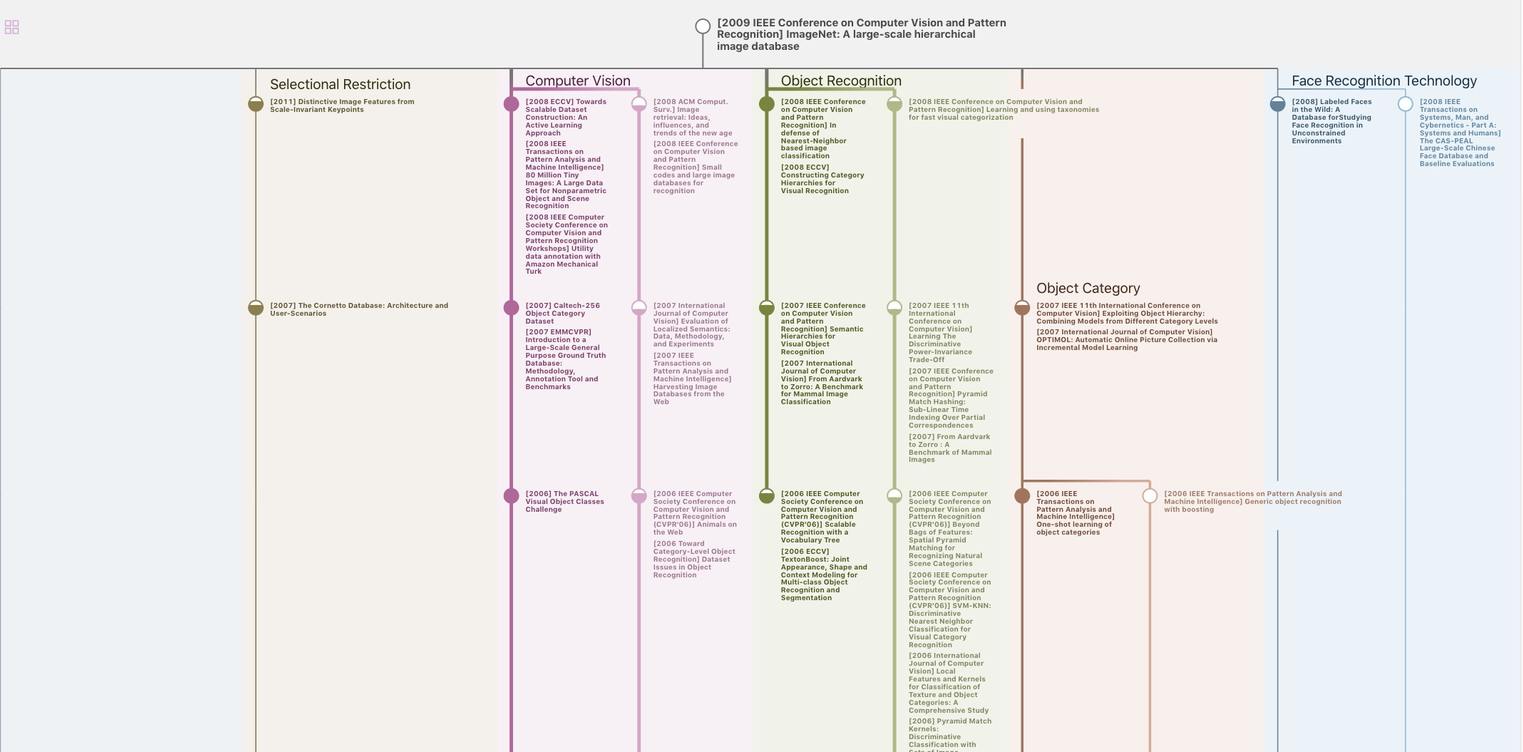
生成溯源树,研究论文发展脉络
Chat Paper
正在生成论文摘要