Single image depth estimation based on sculpture strategy
Knowledge-Based Systems(2022)
摘要
Supervised deep learning approaches have achieved state-of-the-art performance in single image depth estimation. However, the procedure to train the network is not intervened, due to the black box character of deep learning. To enhance the generalization ability of trained models, we employ a learned prior that is inspired by the process of sculpturing, to guide the model’s learning. From the view of sculpturing, the depth estimation can be divided into two consecutive steps: the initial contour estimation and the detail refinement. The contour of scene can be introduced as the intermediate supervision which enables the network to learn true knowledge and accelerate training. In addition, the edges of the scene contain much semantic information. To better use this structured knowledge, we use an attention mechanism to integrate the edges of the scene to guide training. We also create a more precise ground truth by using self-adaptive Gaussian interpolation. Compared to other datasets, our dataset is denser, and the details of small objects and the ordinal relation in the depths of different objects is preserved. Additionally, the distribution of depth values does not change. The experimental results on the KITTI, Make3D and NYU Depth V2 datasets show that the method achieved better performance with respect to other state-of-the-art depth estimation approaches. Code is available at https://github.com/XTU-PR-LAB/HG-Net.
更多查看译文
关键词
3D reconstruction,Depth estimation,Coarse to fine,Gaussian interpolation
AI 理解论文
溯源树
样例
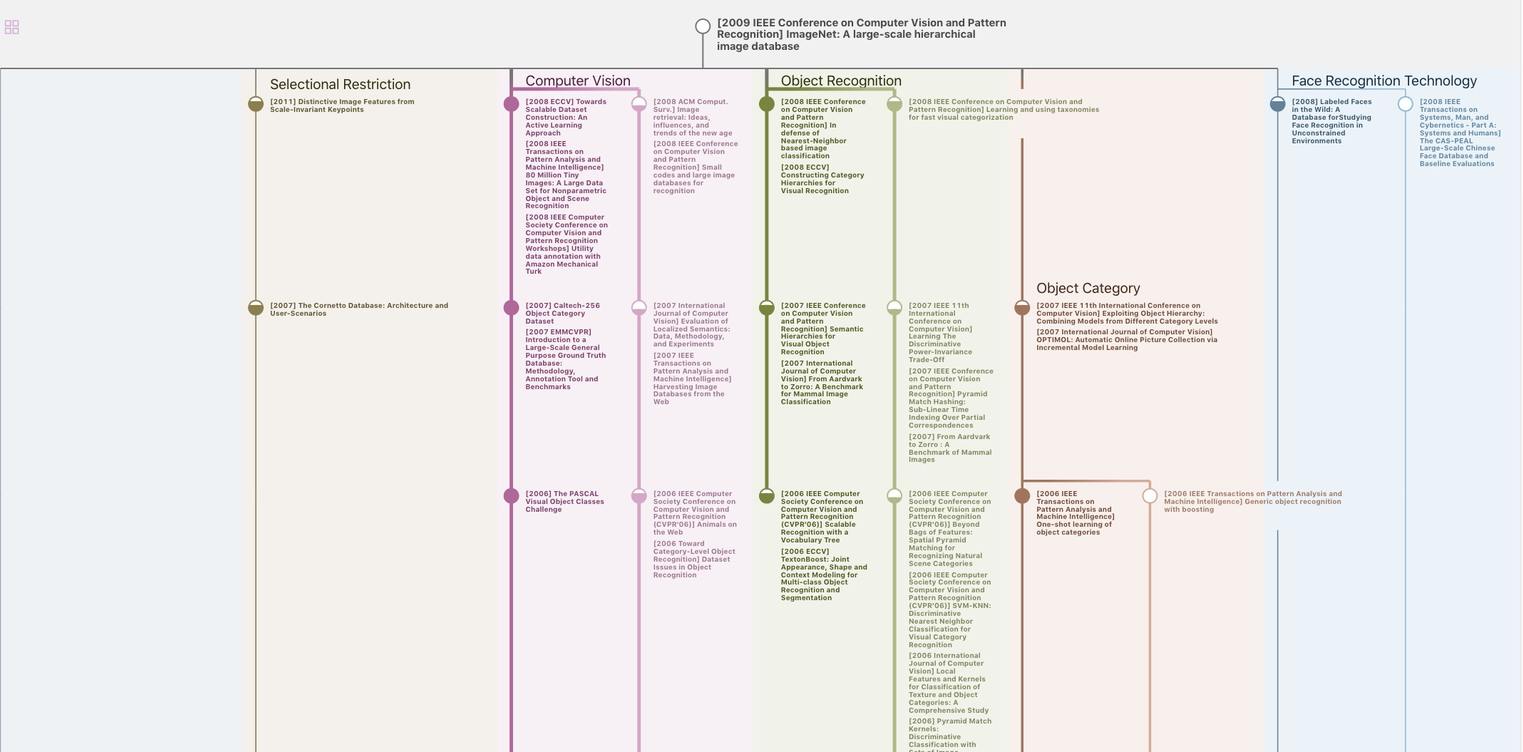
生成溯源树,研究论文发展脉络
Chat Paper
正在生成论文摘要