WCATN: Unsupervised deep learning to classify weather conditions from outdoor images
Engineering Applications of Artificial Intelligence(2022)
摘要
Weather classification from single images is significant for many outdoor computer vision applications, while it has not been thoroughly studied. Existing methods view it as a supervised task under the guidance of weather labels. Though these methods have made great success, they are not applicable to real-world applications because of their reliance on massive human-annotated weather images. In this paper, we take the initial attempt to treat weather classification as an unsupervised task, i.e. to classify weather conditions from unlabeled outdoor images. Specifically, we propose a three-step unsupervised approach to automatically group images into weather clusters. In the first step, i.e. weather feature learning, semantically meaningful weather features are learned by employing a self-supervised task. Furthermore, we also introduce online triplet mining into the self-supervised task to extract discriminative weather features. In the second step, i.e. weather clustering, we design a learnable clustering method via mining the nearest neighbors and enforcing consistency between each sample and its nearest neighbors. To alleviate the uncertainty of weather clustering due to noisy nearest neighbors, we propose a self-labeling method as the third step to employ the already well-classified samples for fine-tuning. Experimental results on two publicly available weather image datasets demonstrate that the proposed approach achieves promising performance.
更多查看译文
关键词
Weather classification,Weather feature learning,Weather clustering,Fine-tuning
AI 理解论文
溯源树
样例
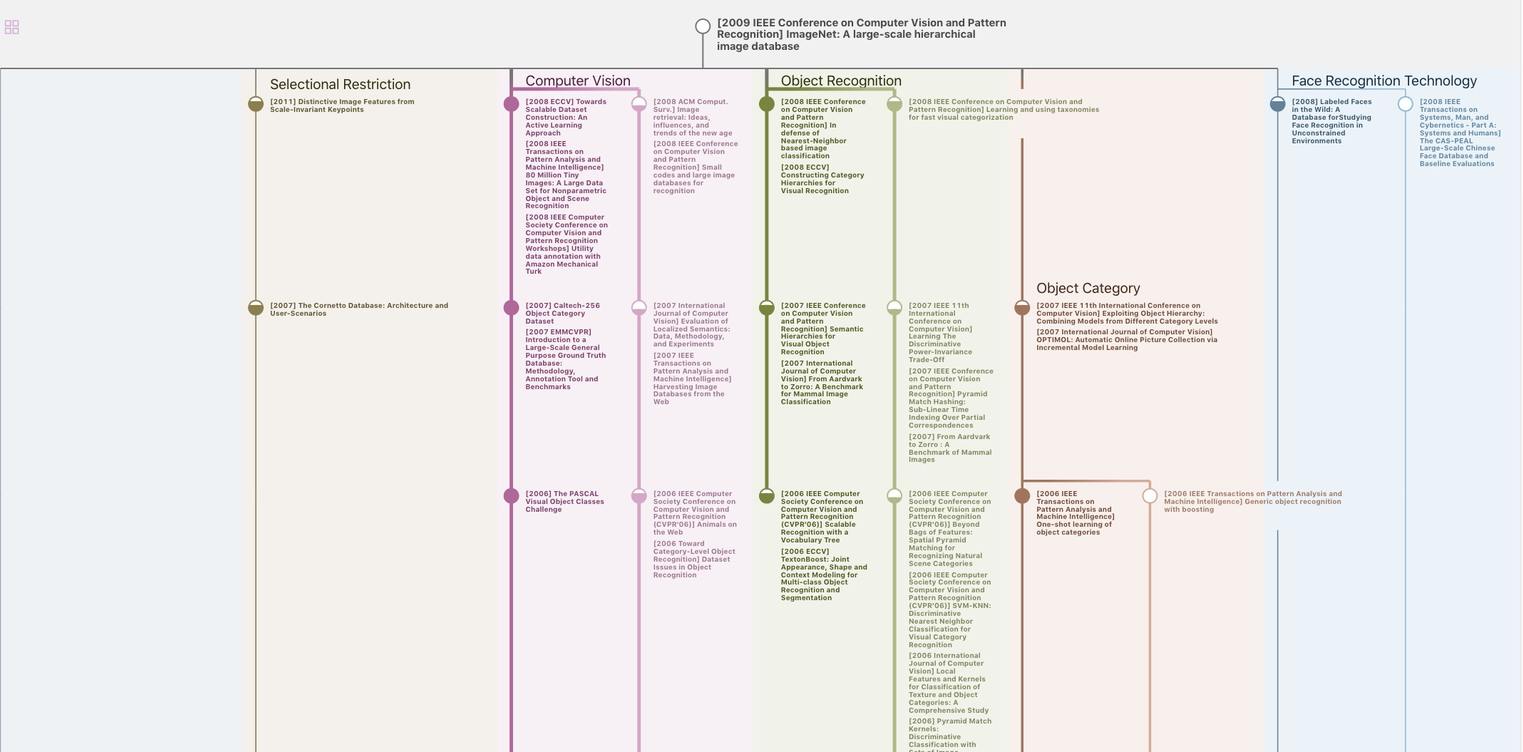
生成溯源树,研究论文发展脉络
Chat Paper
正在生成论文摘要