Machine Learning-Based Matching Medium Design for Implant Communications
IEEE Transactions on Antennas and Propagation(2022)
摘要
Matching media are used in various applications to increase the power transmitted into the human body. The selection of the optimum matching medium (MM) permittivity is not a straightforward task, as the optimum value maximizing the transmitted power depends on the thickness of the MM and the electromagnetic properties of the target tissue. In this article, a computationally heavy empirical approach and a machine learning (ML)-based approach are utilized for the selection of the MM. The empirical approach demonstrates that the MM can increase the
$|S_{21}|$
values up to 8 dB, which is validated with measurements. Next, an ML-based tool is proposed to predict the optimum MM permittivity for any target tissue and any MM thickness. A 1-D convolutional neural network followed by a multilayer perceptron is trained with the simulated average Poynting vector magnitudes (APVMs) for muscle and fat as target tissues. The APVM and the dipole length for given system parameters are predicted by the trained artificial neural network. The accuracy is calculated by comparison with the results of the empirical analysis and found to be 1% and 12.3% mean absolute percentage error for dipole length and APVM, respectively. The proposed tool decreases the time required to milliseconds.
更多查看译文
关键词
Convolutional neural networks (CNNs),implants,in-body link,machine learning (ML)
AI 理解论文
溯源树
样例
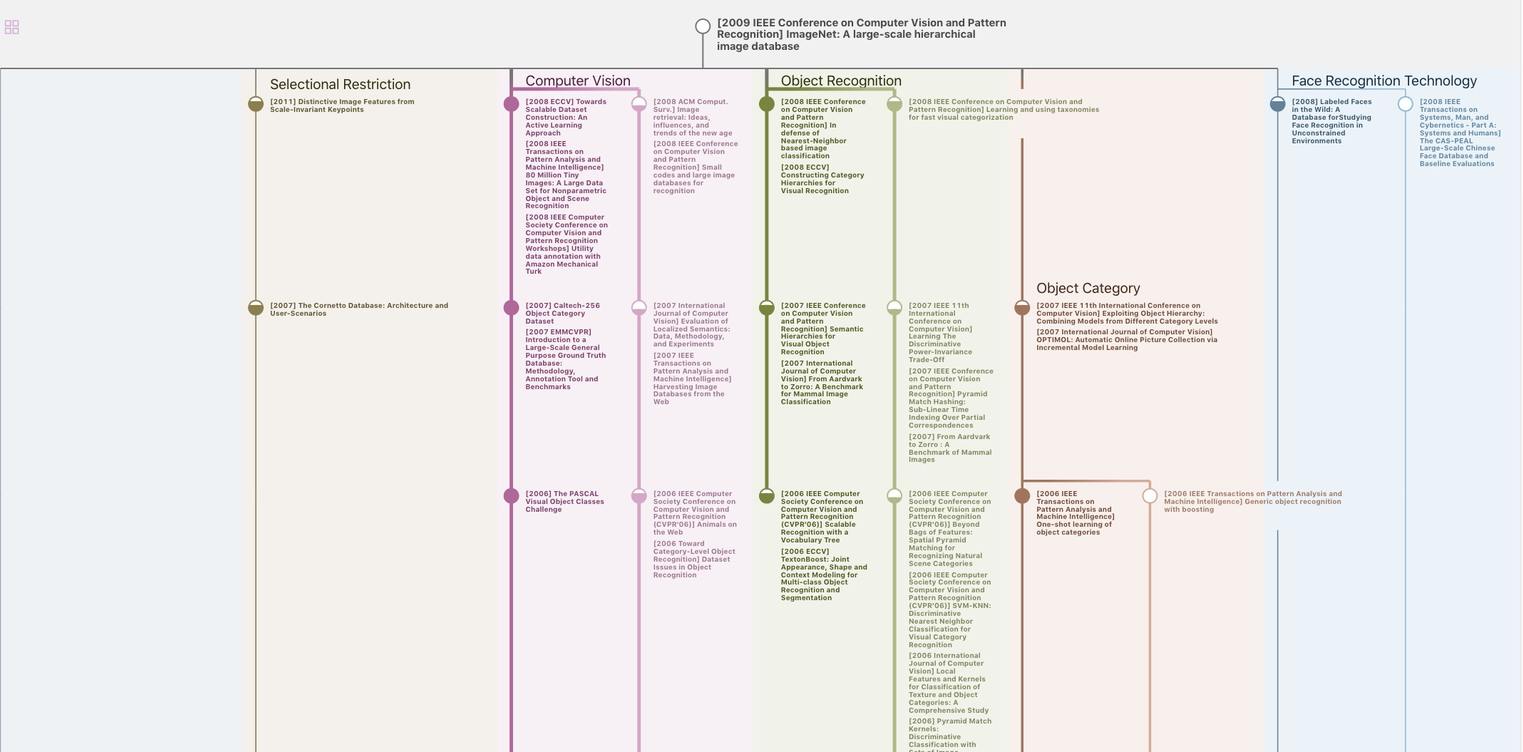
生成溯源树,研究论文发展脉络
Chat Paper
正在生成论文摘要